Application of super-resolution convolutional neural network technique to improve the quality of soft-tissue window cone-beam CT images
Research Square (Research Square)(2021)
Abstract
Abstract Objectives To assess the feasibility of using a super-resolution convolutional neural network to improve the quality of cone-beam computed tomography (CBCT) images for visualizing soft-tissue structures. Methods Multidetector computed tomography (CT) images of 200 subjects who were assessed for the status of an impacted third molar were collected as training datasets. CBCT images of 10 subjects who were also examined with CT were collected as testing datasets. The training process used a modified U-Net and bone and soft-tissue window CT images. After creating a model to convert bone images to soft-tissue images, CBCT images were provided as input and the model outputted estimated CBCT images. These estimated CBCT images were then compared with soft-tissue window CBCT and CT images, using slices through approximately the same anatomical regions. Image evaluation was performed with subjective observations and histogram descriptions. Results The visibility of soft-tissue structures was improved by the technique, with high visibility being attained in the submandibular region, although visibility remained a little obscured in the maxillary region. Conclusions The feasibility of a deep learning-based super resolution technique to improve the visibility of soft-tissue structures on estimated CBCT images was verified.
MoreTranslated text
Key words
super-resolution super-resolution,soft-tissue,cone-beam
AI Read Science
Must-Reading Tree
Example
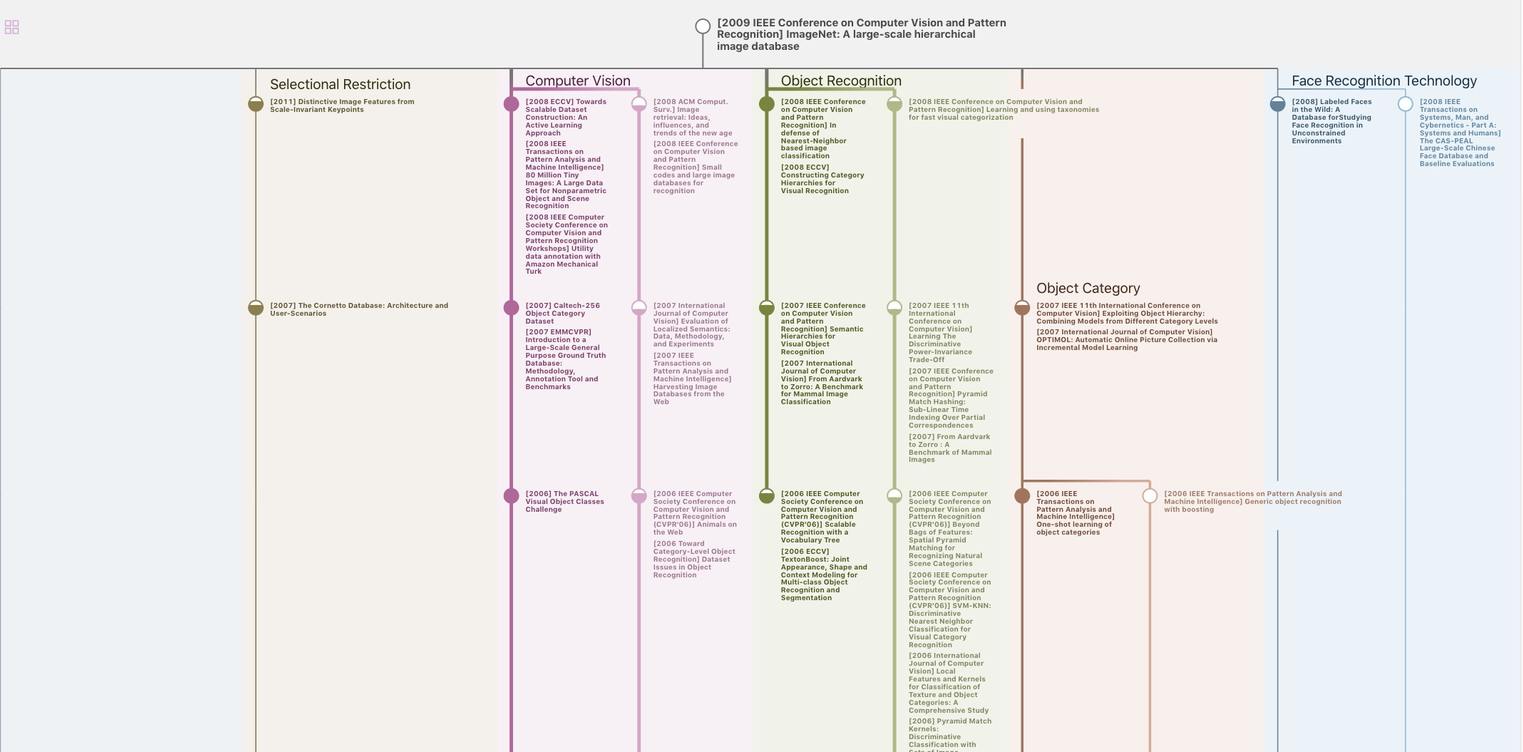
Generate MRT to find the research sequence of this paper
Chat Paper
Summary is being generated by the instructions you defined