Development and internal verification of the hospitalization risk score to triage COVID-19 patients upon admission: a multicenter nested case-control study.
Research Square (Research Square)(2021)
摘要
Abstract Background: Novel coronavirus disease 2019(COVID-19)has a large population base of infection, and only worsening cases required hospitalization. A hospitalization risk score of COVID-19(HRS-COVID-19) to identify and treat worsening patients early thus seems crucial.Method: We conducted a multicenter nested case-control study with 200 cases enrolling confirmed symptomatic COVID-19 patients from four designated hospitals before worsened. Least Absolute Shrinkage and Selection Operator(LASSO), Directed Acyclic Graph(DAG)and Change-in-Estimate(CIE) screened out independent risk factors for HRS-COVID-19 from demographic, clinical and imaging data of the cases. The HRS-COVID-19 was evaluated by the area under curve(AUC), calibration curve, decision curve and clinical impact curve; internal validation by the bootstrap-resampling(boot=1000); and through a nomogram and a network calculator to show.Results: In the nested case cohort, 50 patients reached the compound endpoint(requiring hospitalization) and 150 patients were able to avoid hospitalization. Dyspnea, incubation period, age, lymphocyte count, C-reactive protein and semi-quantitative CT scores were included in HRS-COVID-19. The HRS-COVID-19 has good fitting(Hosmer-Lemeshow goodness,P=0.45); high discrimination(AUC 0.980,95%CI, 0.965-0.996); with excellent calibration and clinical benefits. We make a free online risk calculator (https://hospitalization-risk-score-of-covid-19.shinyapps.io/DynNomapp/).Conclusion: In the study, the HRS-COVID-19 based on the clinical characteristics at admission is helpful for early identification and active treatment of aggravated high-risk COVID-19 patients.
更多查看译文
关键词
hospitalization risk score,case-control
AI 理解论文
溯源树
样例
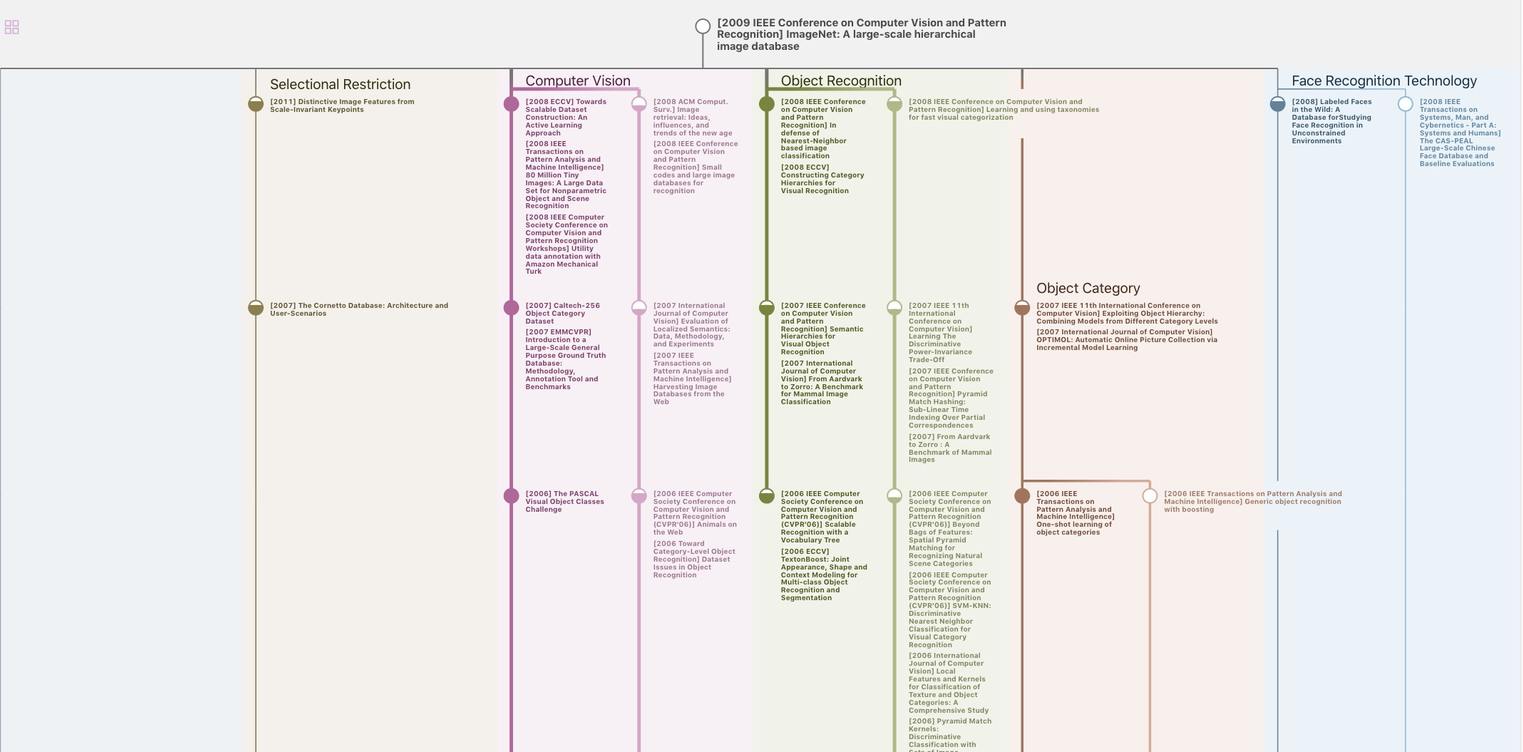
生成溯源树,研究论文发展脉络
Chat Paper
正在生成论文摘要