Evaluation of light atmospheric plume inversion methods using synthetic XCO2 satellite images to compute Paris CO2 emissions
Remote Sensing of Environment(2024)
摘要
There is a growing interest in estimating urban CO2 emission from space-borne imagery of XCO2. Here, we evaluate computationally-light methods (mass-balance approaches and inversions of Gaussian plume models) for estimating urban emissions with Paris, France, as an example. This evaluation is based on the application of those methods to synthetic high-resolution images of the XCO2 field in the Paris area, either with hypothetical 165km×200km images at 1km×1km resolution or using actual ≈2km resolution sampling by OCO-2 and OCO-3. Our work quantifies and analyzes the different sources of error when applying these methods and the corresponding pre-processing steps in detail. We start with ideal test cases with perfect knowledge of the background concentration field and of the driving effective wind and area of the plume from Paris, and without measurement noise in the images. Then we introduce sources of errors associated to these components of the estimation problem step by step. With the best configuration for the different methods, and when accounting for these sources of errors, we obtain total errors in the emission estimates with little bias (<10% of the actual emissions) and a relatively large interquartile range (≈75% of the actual emissions). Overall, the different methods that are tested show similar skills for the emission calculation. The two main sources of uncertainty are the estimates of the background concentration and of the effective wind speed. The error statistics are sensitive to meteorological conditions, mainly to the spatial variability of the wind direction, and to the variability of the background XCO2 field. These sources of error and the results' sensitivity to background and wind field variabilities are theoretically shared by the complex atmospheric inversion methods currently used to quantify urban emissions and the computationally-light methods tested here. Therefore, these conclusions can be extended to the general problem of quantifying urban CO2 emissions from XCO2 imagery. Using OCO-2-like or OCO-3-like samplings, rather than hypothetical 200 km wide images at 1 km resolution, increases the random uncertainty in the emission estimates but not the biases in these estimates.
更多查看译文
关键词
CO2,Urban emissions,Mass-balance methods,Gaussian plumes,OCO-3,OCO-2,GHGs
AI 理解论文
溯源树
样例
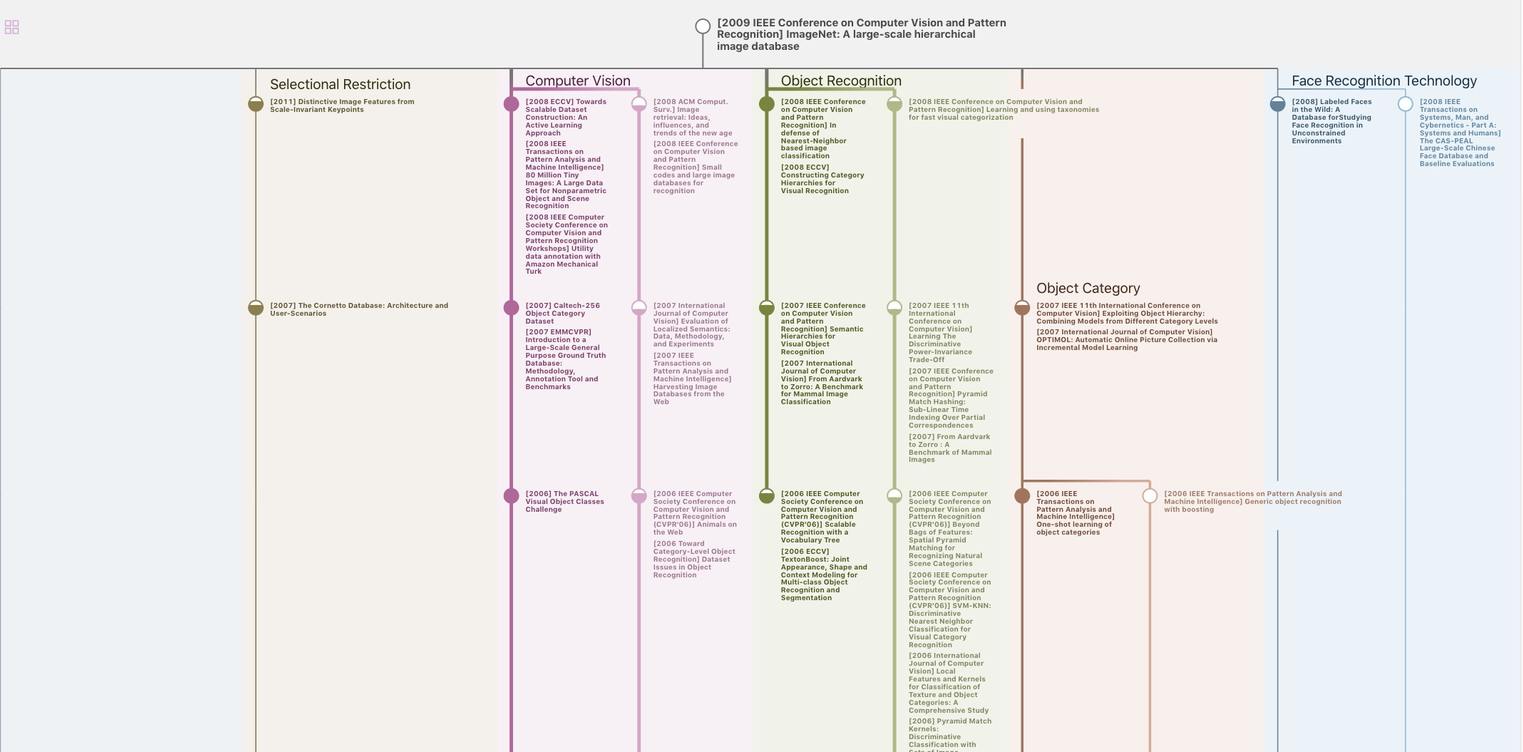
生成溯源树,研究论文发展脉络
Chat Paper
正在生成论文摘要