Event-based Low-Power and Low-Latency Regression Method for Hand Kinematics from Surface EMG
2023 9th International Workshop on Advances in Sensors and Interfaces (IWASI)(2023)
摘要
Human-Machine Interfaces (HMIs) are a rapidly progressing field, and gesture recognition is a promising method in industrial, consumer, and health use cases. Surface electromyography (sEMG) is a State-of-the-Art (SoA) pathway for human-to-machine communication. Currently, the research goal is a more intuitive and fluid control, moving from signal classification of discrete positions to continuous control based on regression. The sEMG-based regression is still scarcely explored in research since most approaches have addressed classification. In this work, we propose the first event-based EMG encoding applied to the regression of hand kinematics suitable for working in streaming on a low-power microcontroller (STM32 F401, mounting ARM Cortex-M4). The motivation for event-based encoding is to exploit upcoming neuromorphic hardware to benefit from reduced latency and power consumption. We achieve a Mean Absolute Error of $8.8\pm 2.3$ degrees on 5 degrees of actuation on the public dataset NinaPro DB8, comparable with the SoA Deep Neural Network (DNN). We use $9\times$ less memory and $13\times$ less energy per inference, with $10\times$ shorter latency per inference compared to the SoA deep net, proving suitable for resource-constrained embedded platforms.
更多查看译文
关键词
electromyography,EMG,surface electromyography,sEMG,hand kinematics,time series,event-based,silicon cochlea,spike trains,regression,machine learning,ML,embedded,microcontrollers,MCU,STM32,ultra-low-power,low-latency,real-time,streaming,event-driven,neuromorphic
AI 理解论文
溯源树
样例
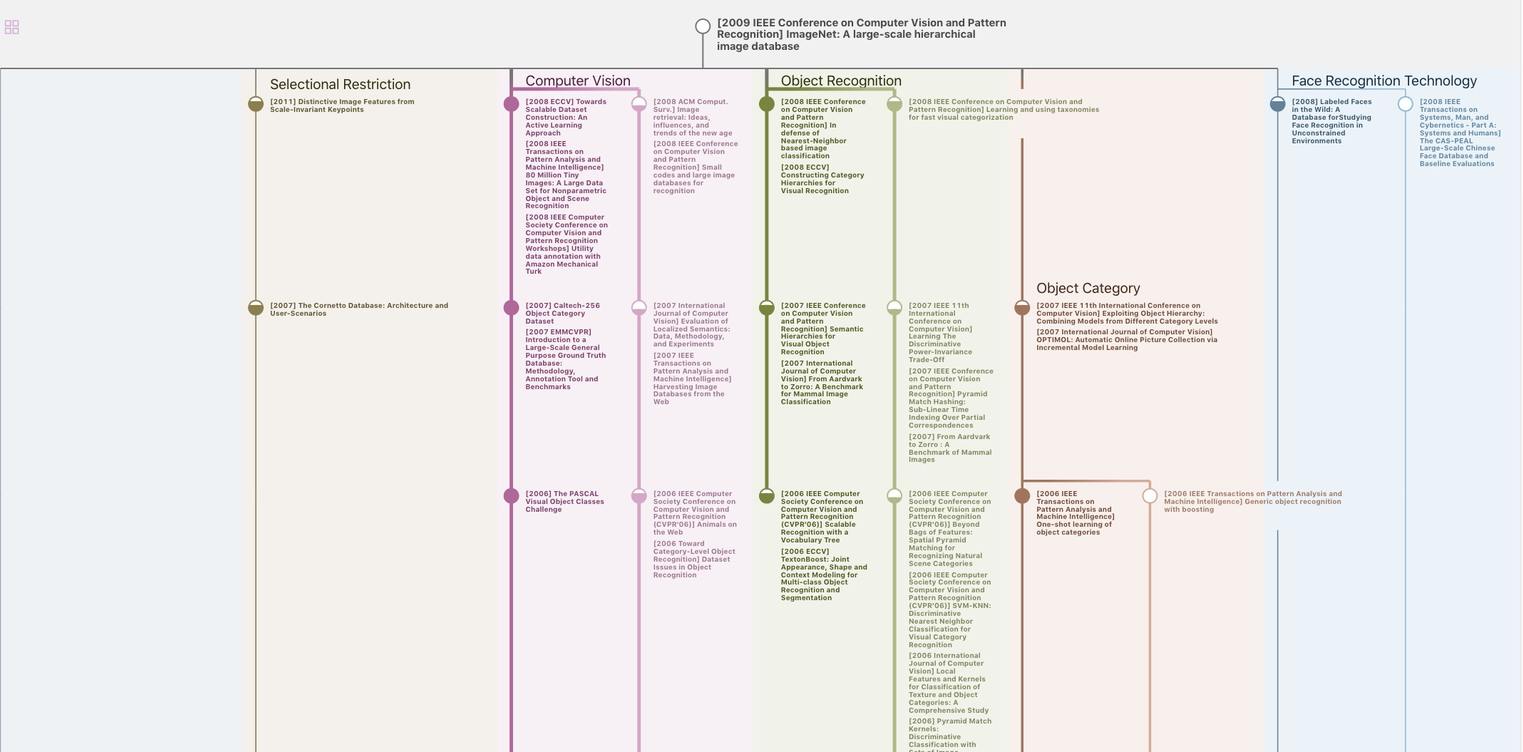
生成溯源树,研究论文发展脉络
Chat Paper
正在生成论文摘要