A Survey on Auto-Parallelism of Large-Scale Deep Learning Training
IEEE Transactions on Parallel and Distributed Systems(2023)
Abstract
Deep learning (DL) has gained great success in recent years, leading to state-of-the-art performance in research community and industrial fields like computer vision and natural language processing. One of the reasons for this success is the huge amount parameters adopted in DL models. However, it is impractical to train a moderately large model with a large number of parameters on a typical single device. Thus, It is necessary to train DL models in clusters with distributed training algorithms. However, traditional distributed training algorithms are usually sub-optimal and highly customized, which owns the drawbacks to train large-scale DL models in varying computing clusters. To handle the above problem, researchers propose auto-parallelism, which is promising to train large-scale DL models efficiently and practically in various computing clusters. In this survey, we perform a broad and thorough investigation on challenges, basis, and strategy searching methods of auto-parallelism in DL training. First, we abstract basic parallelism schemes with their communication cost and memory consumption in DL training. Further, we analyze and compare a series of current auto-parallelism works and investigate strategies and searching methods which are commonly used in practice. At last, we discuss several trends in auto-parallelism which are promising in further research.
MoreTranslated text
Key words
deep learning,large-scale large-scale,auto-parallelism
AI Read Science
Must-Reading Tree
Example
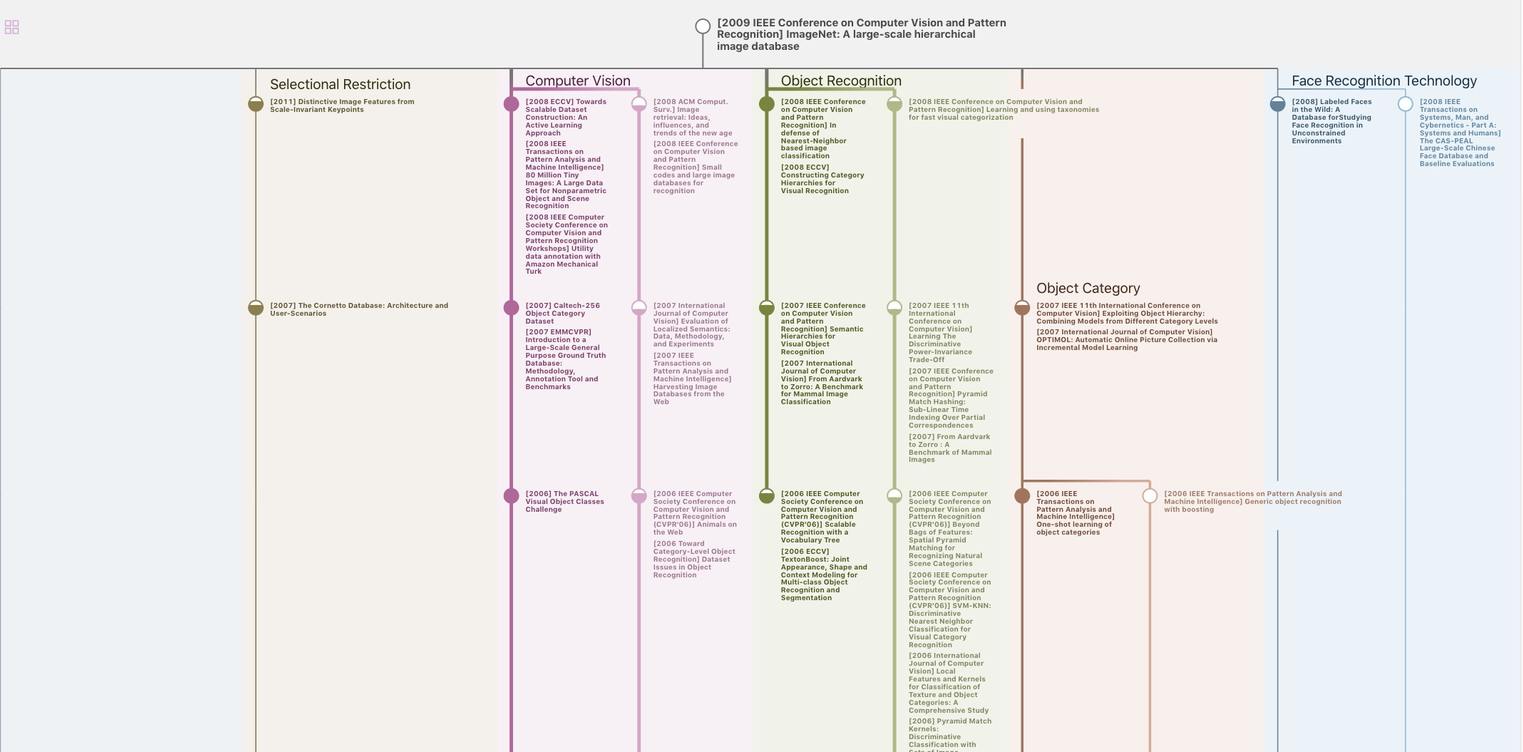
Generate MRT to find the research sequence of this paper
Chat Paper
Summary is being generated by the instructions you defined