AI-guided use of balanced PPARα/γ dual agonist finetunes macrophage responses in inflammatory bowel disease
crossref(2021)
Abstract
Abstract A computational platform, the Boolean network explorer (BoNE), has recently been developed to infuse AI-enhanced precision into drug discovery; it enables querying and navigating invariant Boolean Implication Networks of disease maps for prioritizing high-value targets. Here we used BoNE to query an Inflammatory Bowel Disease (IBD)-map and prioritize two nuclear receptors, PPARα/γ. Balanced agonism of PPARα/γ was predicted to impact macrophage processes, ameliorate colitis in network-prioritized animal models, ‘reset’ the gene expression network from disease to health, and achieve a favorable therapeutic index that tracked other FDA-approved targets. Predictions were validated using a balanced and potent PPARα/γ-dual agonist (PAR5359) in two pre-clinical murine models, i.e., Citrobacter rodentium-induced infectious colitis and DSS-induced colitis. Mechanistically, we show that such balanced dual agonism promotes bacterial clearance more efficiently than individual agonists both in vivo and in vitro; PPARα/γ is required and its agonism is sufficient to induce the pro-inflammatory cytokines and cellular ROS, which are essential for bacterial clearance and immunity, whereas PPARα/γ-agonism blunts these responses, delays microbial clearance and induces the anti-inflammatory cytokine, IL10. Balanced agonism achieved controlled inflammation while protecting the gut barrier and ‘reversal’ of the transcriptomic network. Furthermore, dual agonism effectively reversed the defective bacterial clearance observed in PBMCs derived from IBD patients. These findings not only deliver a macrophage modulator for use as barrier-protective therapy in IBD, but also highlight the potential of BoNE to accelerate and enhance the precision of drug discovery.
MoreTranslated text
AI Read Science
Must-Reading Tree
Example
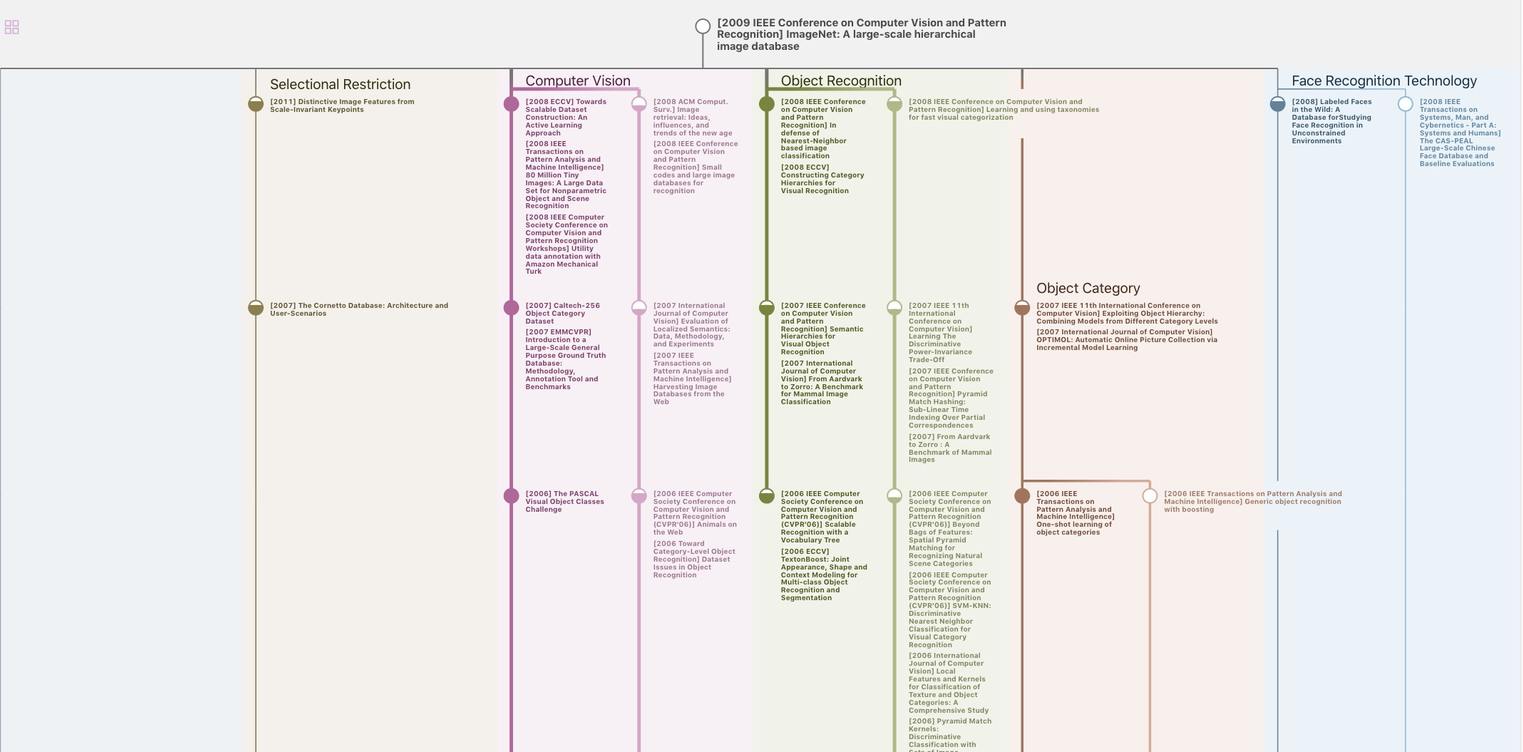
Generate MRT to find the research sequence of this paper
Chat Paper
Summary is being generated by the instructions you defined