Rapid and Flexible Semantic Segmentation of Electron Microscopy Data Using Few-Shot Machine Learning
crossref(2021)
摘要
Abstract Semantic segmentation of key microstructural features in atomic-scale electron microscope images is critical to improved understanding of structure-property relationships in many important materials and chemical systems. However, the present paradigm involves time-intensive manual analysis that is inherently biased, error-prone, and unable to accommodate the large volumes of data produced by modern instrumentation. While more automated approaches have been proposed, many are not robust to a high variety of data, and do not generalize well to diverse microstructural features and material systems. Here, we present a flexible, semi-supervised few-shot machine learning approach for semantic segmentation of scanning transmission electron microscopy images of three oxide material systems: (1) epitaxial heterostructures of SrTiO3 / Ge, (2) La0.8Sr0.2FeO3 thin films, and (3) MoO3 nanoparticles. We demonstrate that the few-shot learning method is more robust against noise, more reconfigurable, and requires less data than conventional image analysis methods. This approach can enable rapid image classification and microstructural feature mapping needed for emerging high-throughput and autonomous microscope platforms.
更多查看译文
AI 理解论文
溯源树
样例
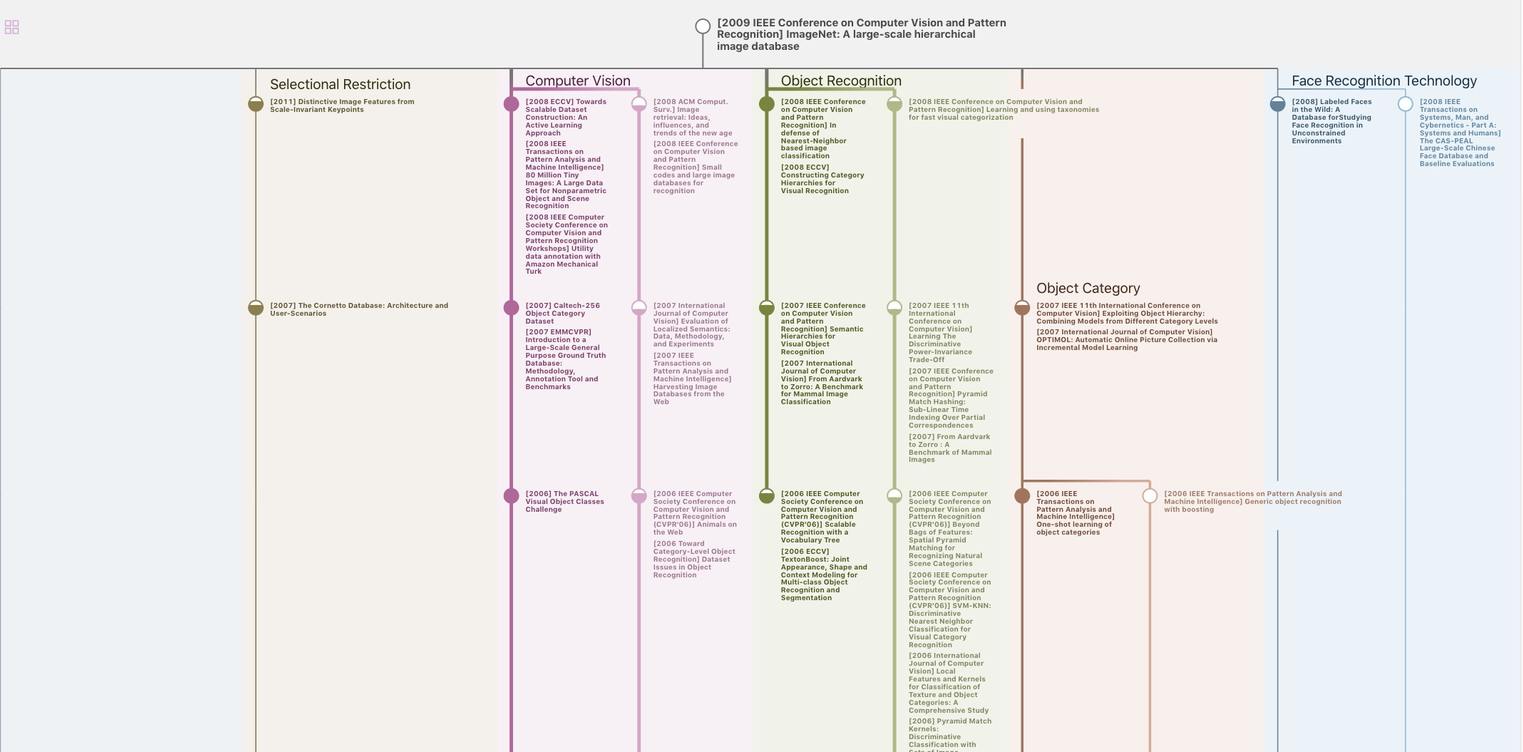
生成溯源树,研究论文发展脉络
Chat Paper
正在生成论文摘要