Histological classification of non-small cell lung cancer using Gabor filtering Minkowski functionals and Neighborhood Component Analysis on CT images.
Research Square (Research Square)(2021)
Abstract
Abstract Information regarding the histological types of non-small cell lung cancer is essential to determine the treatment strategy. Although several radiomics studies using almost similar feature variables were reported, a considerable variation in the performances has been observed. In this study, as novel radiomic features, 2D Gabor filtering Minkowski functionals were used. They were calculated in rotational invariant and both scale and rotational invariant ways using circular shift operations of Gabor filters on nonenhanced computed tomographic images. Eighty-six patients (47 adenocarcinomas, 39 squamous cell carcinomas) were analyzed. Two independent observers manually delineated a single slice segmentation of a tumor. Feature selection was made by neighborhood component analysis. Among various classifiers, 1-nearest neighbor gave a promising performance. The observer-averaged accuracy of rotational invariant analysis was 86.28% and that of both scale and rotational invariant one was 88.27%. However, there was no common feature among the ten top-ranked features of each observer with the identical Gabor filtering type. Hence further study of the robustness is necessary to create a more reliable model.
MoreTranslated text
Key words
lung cancer,filtering minkowski functionals,functionals images,neighborhood component analysis,histological classification,non-small
AI Read Science
Must-Reading Tree
Example
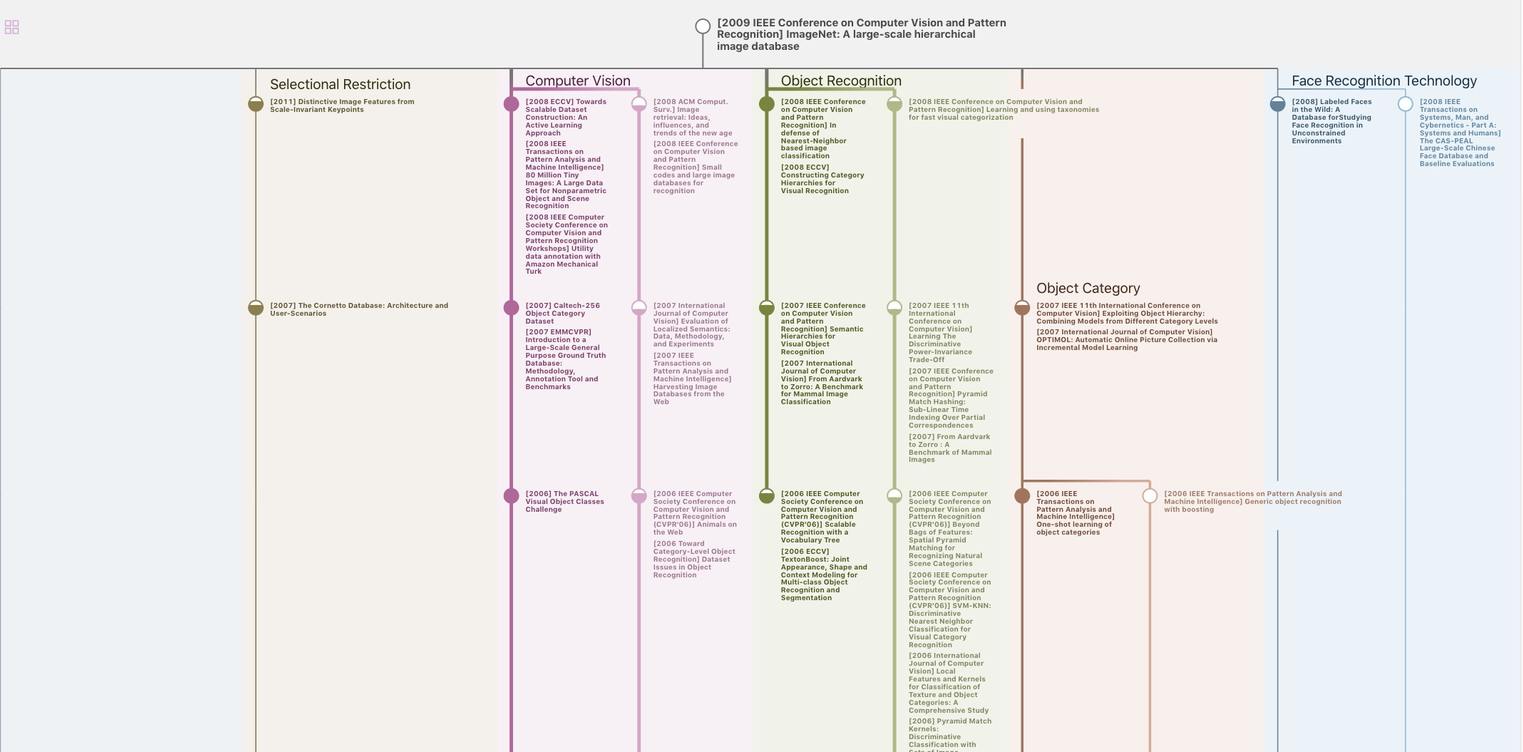
Generate MRT to find the research sequence of this paper
Chat Paper
Summary is being generated by the instructions you defined