Re-thinking the fixed-criterion model of perceptual decision-making
crossref(2021)
Abstract
Perceptual decision-making is often conceptualized as the process of comparing an internal decision variable to a categorical boundary, or criterion. How the mind sets such a criterion has been studied from at least two perspectives. First, researchers interested in consciousness have proposed that criterion-crossing determines whether a stimulus is consciously perceived. Second, researchers interested in decision-making have studied how the criterion depends on a range of stimulus and task variables. Both communities have considered the question of how the criterion behaves when sensory information is weak or uncertain. Interestingly, however, they have arrived at different conclusions. Consciousness researchers investigating a phenomenon called "subjective inflation" – a form of metacognitive mismatch in which observers overestimate the quality of their sensory representations in the periphery or at an unattended location – have proposed that the criterion governing subjective visibility is fixed. That is, it does not adjust to changes in sensory uncertainty. Decision-making researchers, on the other hand, have concluded that the criterion does adjust to account for sensory uncertainty, including under inattention. Here, we mathematically demonstrate that previous empirical findings supporting subjective inflation are consistent with either a fixed or a flexible decision criterion. We further show that specific experimental task requirements are necessary to make inferences about the flexibility of the criterion: 1) a clear mapping from decision variable space to stimulus feature space, and 2) a task incentive for observers to adjust their decision criterion as response variance increases. We conclude that the fixed-criterion model of subjective inflation requires re-thinking in light of new evidence from the probabilistic reasoning literature that decision criteria flexibly adjust according to response variance.
MoreTranslated text
AI Read Science
Must-Reading Tree
Example
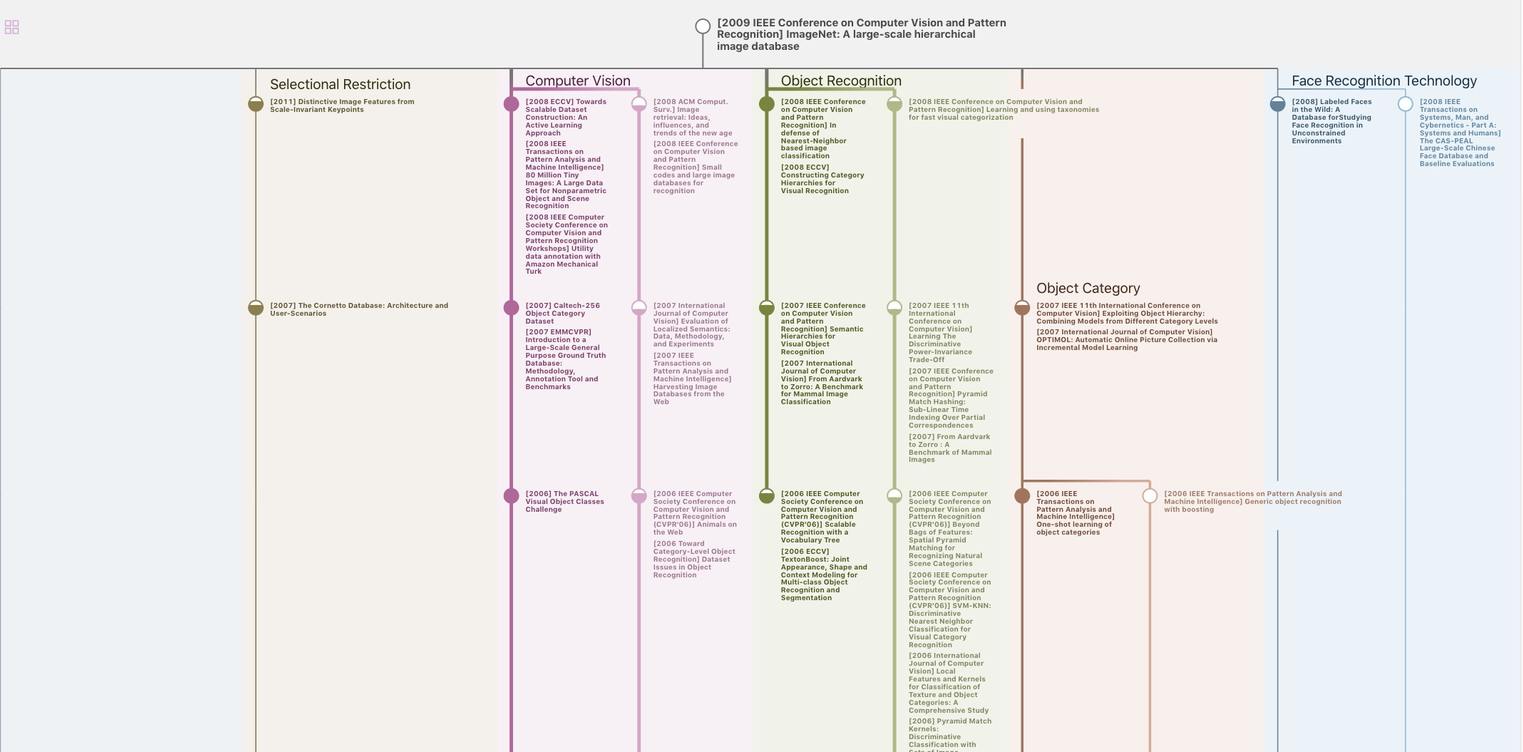
Generate MRT to find the research sequence of this paper
Chat Paper
Summary is being generated by the instructions you defined