Performance study of convolutional neural network architectures for 3D incompressible flow simulations
PASC(2023)
摘要
Recently, correctly handling spatial information from multiple scales has proven to be essential in Machine Learning (ML) applications on Computational Fluid Dynamics (CFD) problems. For these type of applications, Convolutional Neural Networks (CNN) that use Multiple Downsampled Branches (MDBs) to efficiently encode spatial information from different spatial scales have proven to be some of the most successful architectures. However, not many guidelines exist to build these architectures, particularly when applied to more challenging 3D configurations. Thus, this work focuses on studying the impact of the choice of the number of downsampled branches, accuracy and performance-wise in 3D incompressible fluid test cases, where a CNN is used to solve the Poisson equation. The influence of this parameter is assessed by performing multiple trainings of Unet architectures with varying MDBs on a cloud-computing environment. These trained networks are then tested on two 3D CFD problems: a plume and a Von Karman vortex street at various operating points, where the solution of the neural network is coupled to a nonlinear advection equation.
更多查看译文
关键词
CNN, Poisson equation, CFD, incompressible flows
AI 理解论文
溯源树
样例
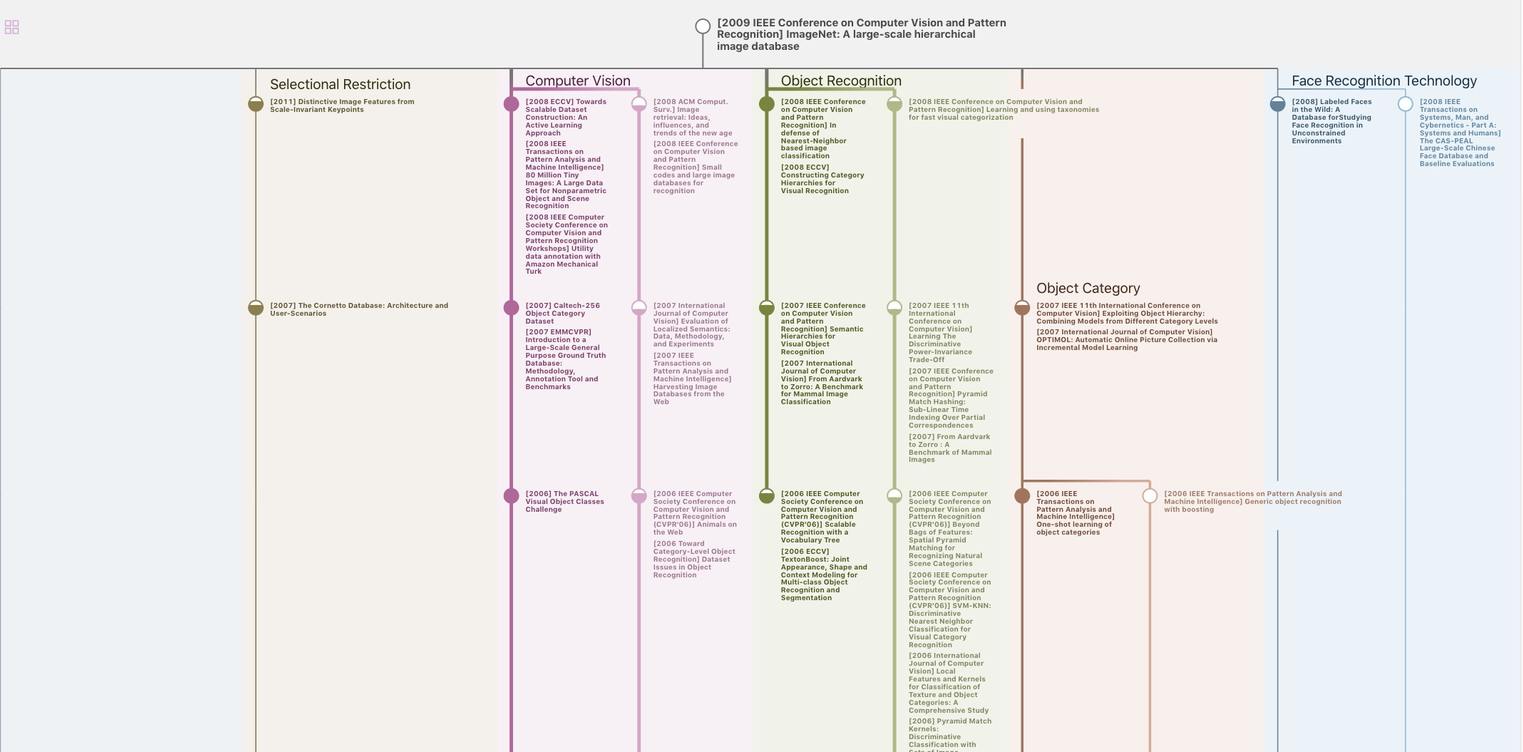
生成溯源树,研究论文发展脉络
Chat Paper
正在生成论文摘要