A Unified Batch Hierarchical Reinforcement Learning Framework for Pedagogical Policy Induction with Deep Bisimulation Metrics.
AIED (Posters/Late Breaking Results/...)(2023)
摘要
Intelligent Tutoring Systems (ITSs) leverage AI to adapt to individual students, and employ pedagogical policies to decide what instructional action to take next. A number of researchers applied Reinforcement Learning (RL) and Deep RL (DRL) to induce effective pedagogical policies. Most prior work, however, has been developed independently for a specific ITS and cannot directly be applied to another. In this work, we propose a Multi-Task Learning framework that combines Deep BIsimulation Metrics and DRL, named MTL-BIM, to induce a unified pedagogical policy for two different ITSs across different domains: logic and probability. Based on empirical classroom results, our unified RL policy performed significantly better than the expert-crafted policies and independently induced DQN policies on both ITSs.
更多查看译文
关键词
pedagogical policy induction,reinforcement,learning
AI 理解论文
溯源树
样例
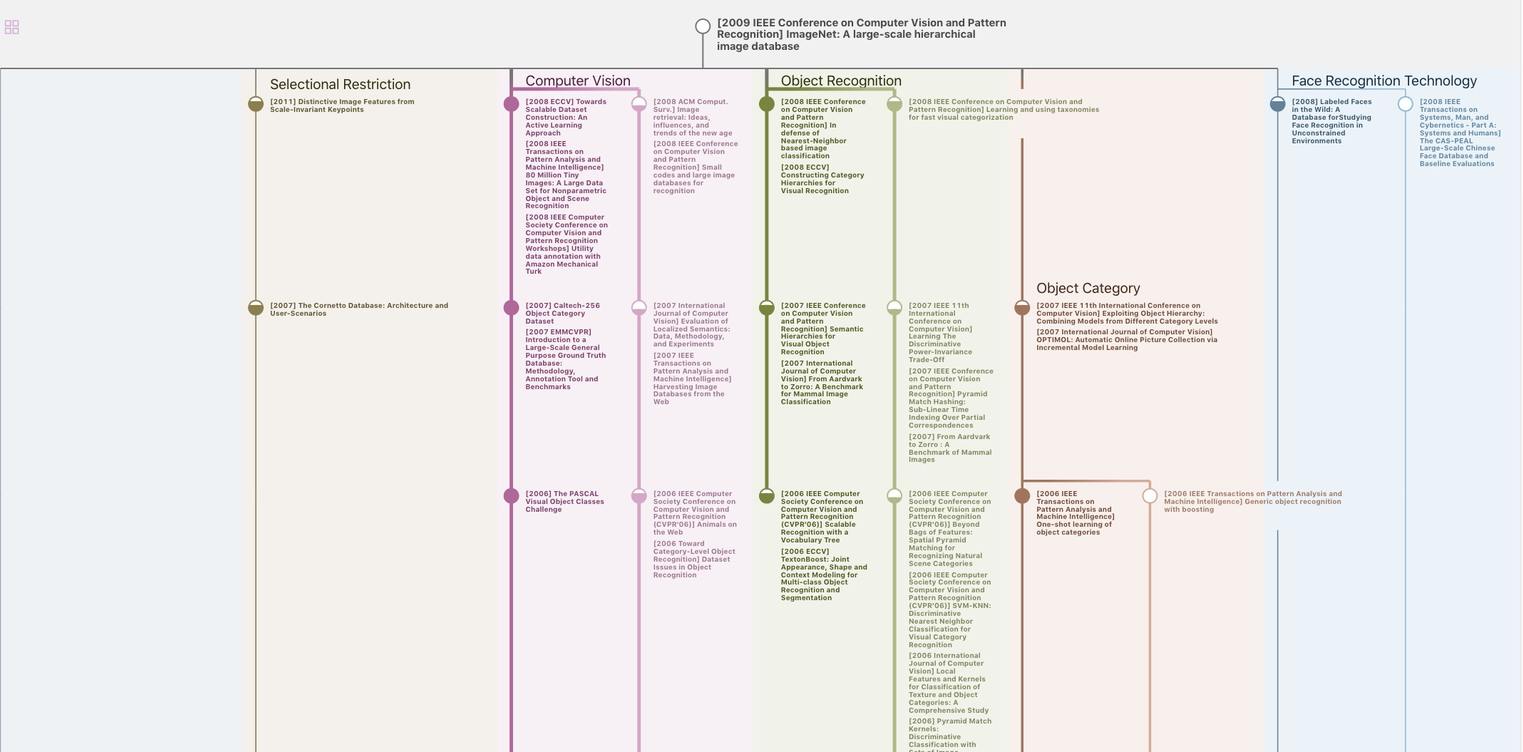
生成溯源树,研究论文发展脉络
Chat Paper
正在生成论文摘要