IH-TCGAN: Time-Series Conditional Generative Adversarial Network with Improved Hausdorff Distance for Synthesizing Intention Recognition Data.
Entropy(2023)
摘要
As military technology continues to evolve and the amount of situational information available on the battlefield continues to increase, data-driven deep learning methods are becoming the primary method for air target intention recognition. Deep learning is based on a large amount of high quality data; however, in the field of intention recognition, it often faces key problems such as low data volume and unbalanced datasets due to insufficient real-world scenarios. To address these problems, we propose a new method called time-series conditional generative adversarial network with improved Hausdorff distance (IH-TCGAN). The innovation of the method is mainly reflected in three aspects: (1) Use of a transverter to map real and synthetic data into the same manifold so that they have the same intrinsic dimension; (2) Addition of a restorer and a classifier in the network structure to ensure that the model can generate high-quality multiclass temporal data; (3) An improved Hausdorff distance is proposed that can measure the time order differences between multivariate time-series data and make the generated results more reasonable. We conduct experiments using two time-series datasets, evaluate the results using various performance metrics, and visualize the results using visualization techniques. The experimental results show that IH-TCGAN is able to generate synthetic data similar to the real data and has significant advantages in the generation of time series data.
更多查看译文
关键词
intention recognition data,ih-tcgan,time-series
AI 理解论文
溯源树
样例
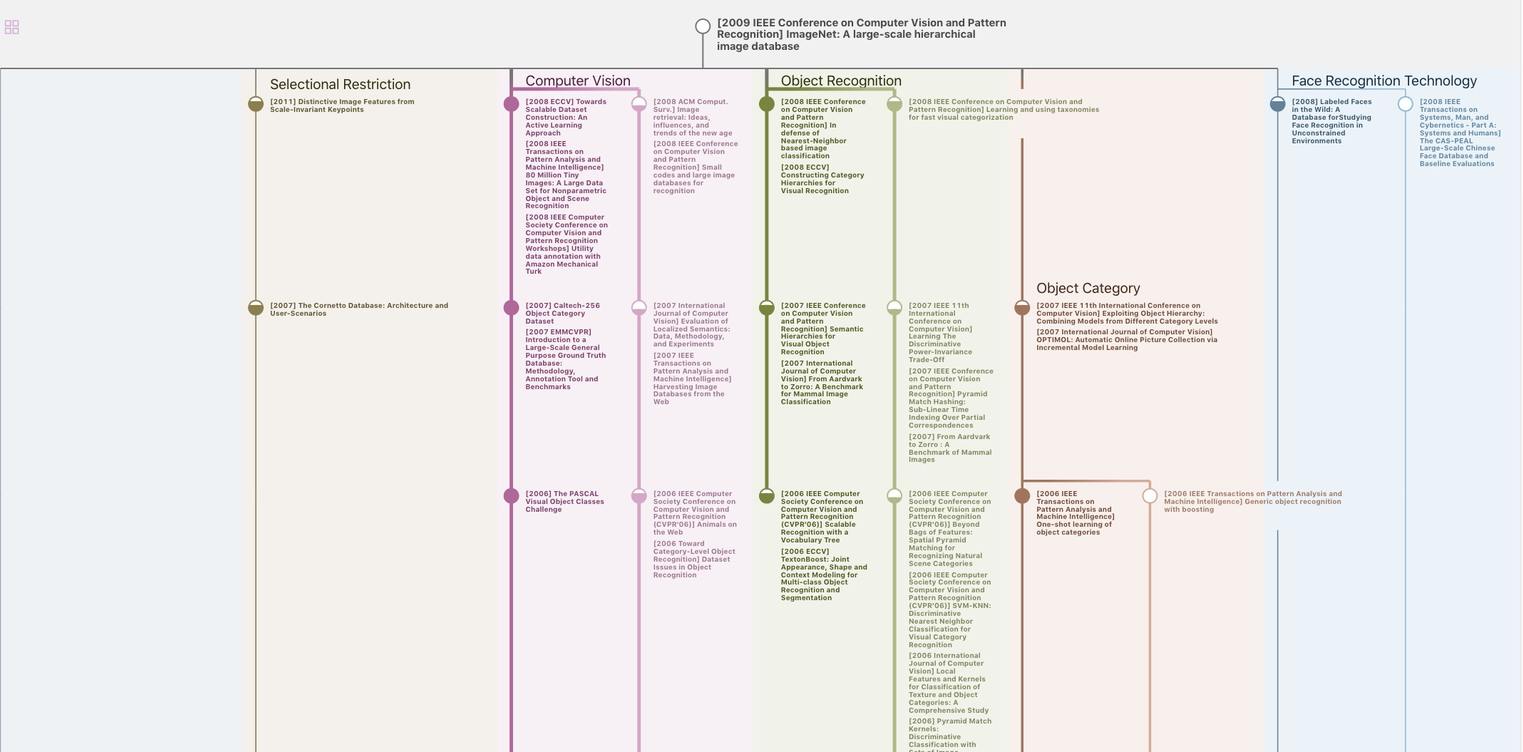
生成溯源树,研究论文发展脉络
Chat Paper
正在生成论文摘要