PEPPR: A post-encoding pre-production reinstatement model of dual-list free recall
Memory & Cognition(2023)
Abstract
Recent events are easy to recall, but they also interfere with the recall of more distant, non-recent events. In many computational models, non-recent memories are recalled by using the context associated with those events as a cue. Some models, however, do little to explain how people initially activate non-recent contexts in the service of accurate recall. We addressed this limitation by evaluating two candidate mechanisms within the Context-Maintenance and Retrieval model. The first is a Backward-Walk mechanism that iteratively applies a generate/recognize process to covertly retrieve progressively less recent items. The second is a Post-Encoding Pre-Production Reinstatement (PEPPR) mechanism that formally implements a metacognitive control process that reinstates non-recent contexts prior to retrieval. Models including these mechanisms make divergent predictions about the dynamics of response production and monitoring when recalling non-recent items. Before producing non-recent items, Backward-Walk cues covert retrievals of several recent items, whereas PEPPR cues few, if any, covert retrievals of that sort. We tested these predictions using archival data from a dual-list externalized free recall paradigm that required subjects to report all items that came to mind while recalling from the non-recent list. Simulations showed that only the model including PEPPR accurately predicted covert recall patterns. That same model fit the behavioral data well. These findings suggest that self-initiated context reinstatement plays an important role in recall of non-recent memories and provides a formal model that uses a parsimonious non-hierarchical context representation of how such reinstatement might occur.
MoreTranslated text
Key words
Cognitive control,Free recall,Metacognition,Source monitoring,Temporal contiguity
AI Read Science
Must-Reading Tree
Example
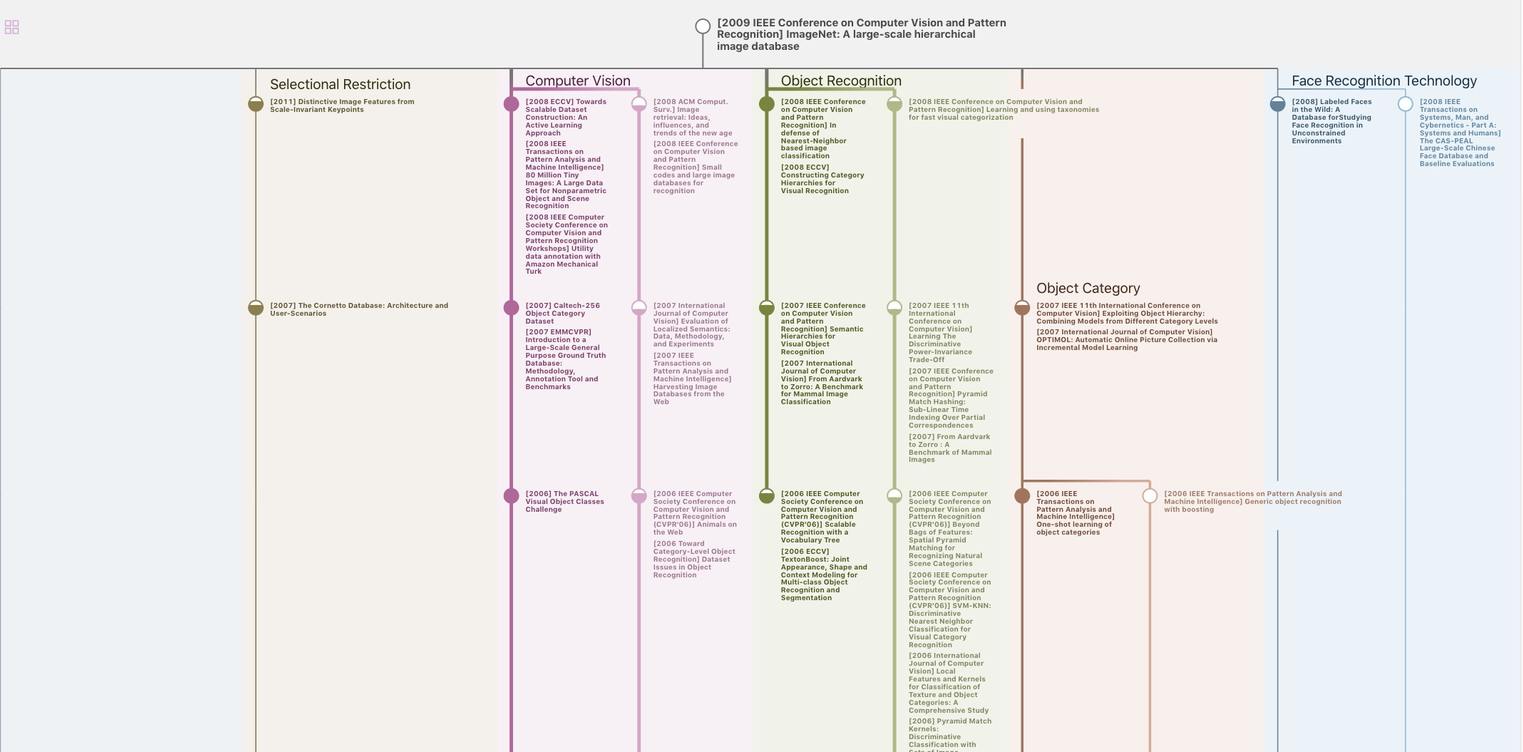
Generate MRT to find the research sequence of this paper
Chat Paper
Summary is being generated by the instructions you defined