A novel approach for predicting epidemiological forecasting parameters based on real-time signals and Data Assimilation
CoRR(2023)
摘要
This paper proposes a novel approach to predict epidemiological parameters by integrating new real-time signals from various sources of information, such as novel social media-based population density maps and Air Quality data. We implement an ensemble of Convolutional Neural Networks (CNN) models using various data sources and fusion methodology to build robust predictions and simulate several dynamic parameters that could improve the decision-making process for policymakers. Additionally, we used data assimilation to estimate the state of our system from fused CNN predictions. The combination of meteorological signals and social media-based population density maps improved the performance and flexibility of our prediction of the COVID-19 outbreak in London. While the proposed approach outperforms standard models, such as compartmental models traditionally used in disease forecasting (SEIR), generating robust and consistent predictions allows us to increase the stability of our model while increasing its accuracy.
更多查看译文
关键词
epidemiological forecasting parameters
AI 理解论文
溯源树
样例
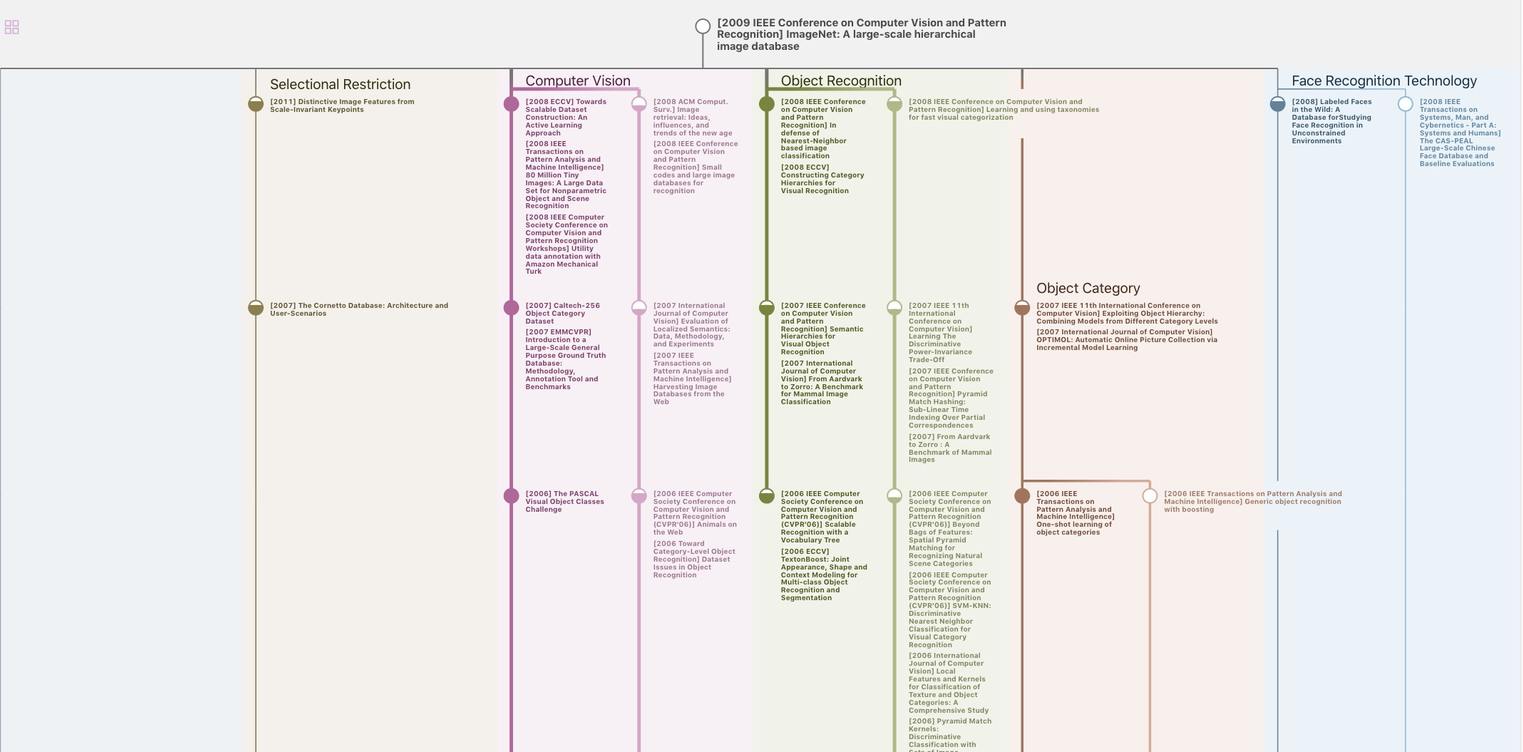
生成溯源树,研究论文发展脉络
Chat Paper
正在生成论文摘要