Empirically Validating Conformal Prediction on Modern Vision Architectures Under Distribution Shift and Long-tailed Data
CoRR(2023)
摘要
Conformal prediction has emerged as a rigorous means of providing deep learning models with reliable uncertainty estimates and safety guarantees. Yet, its performance is known to degrade under distribution shift and long-tailed class distributions, which are often present in real world applications. Here, we characterize the performance of several post-hoc and training-based conformal prediction methods under these settings, providing the first empirical evaluation on large-scale datasets and models. We show that across numerous conformal methods and neural network families, performance greatly degrades under distribution shifts violating safety guarantees. Similarly, we show that in long-tailed settings the guarantees are frequently violated on many classes. Understanding the limitations of these methods is necessary for deployment in real world and safety-critical applications.
更多查看译文
关键词
conformal prediction,modern vision
AI 理解论文
溯源树
样例
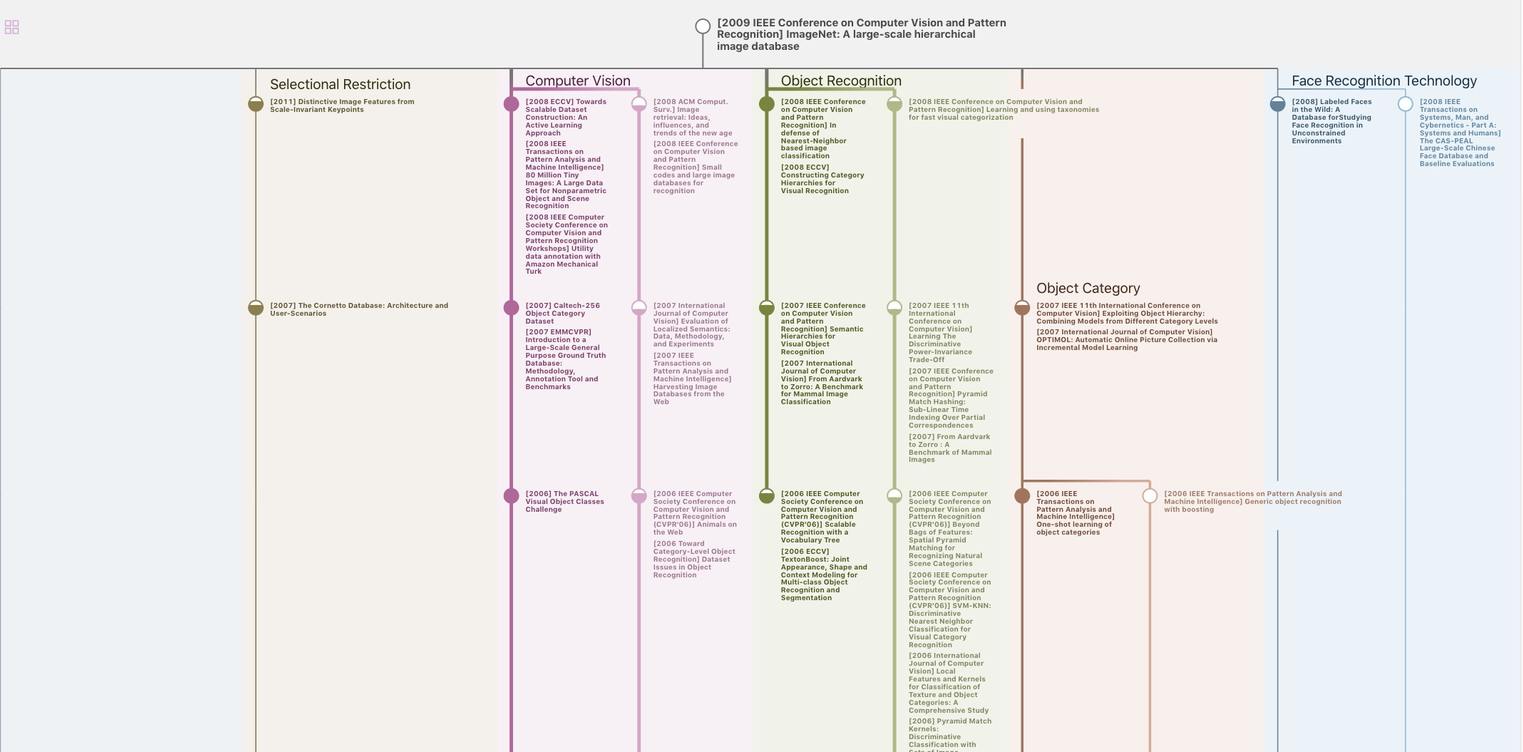
生成溯源树,研究论文发展脉络
Chat Paper
正在生成论文摘要