Face Aging by Explainable Conditional Adversarial Autoencoders.
Journal of imaging(2023)
Abstract
This paper deals with Generative Adversarial Networks (GANs) applied to face aging. An explainable face aging framework is proposed that builds on a well-known face aging approach, namely the Conditional Adversarial Autoencoder (CAAE). The proposed framework, namely, xAI-CAAE, couples CAAE with explainable Artificial Intelligence (xAI) methods, such as Saliency maps or Shapley additive explanations, to provide corrective feedback from the discriminator to the generator. xAI-guided training aims to supplement this feedback with explanations that provide a "reason" for the discriminator's decision. Moreover, Local Interpretable Model-agnostic Explanations (LIME) are leveraged to provide explanations for the face areas that most influence the decision of a pre-trained age classifier. To the best of our knowledge, xAI methods are utilized in the context of face aging for the first time. A thorough qualitative and quantitative evaluation demonstrates that the incorporation of the xAI systems contributed significantly to the generation of more realistic age-progressed and regressed images.
MoreTranslated text
Key words
explainable AI (xAI),generative adversarial networks (GANs),face aging
AI Read Science
Must-Reading Tree
Example
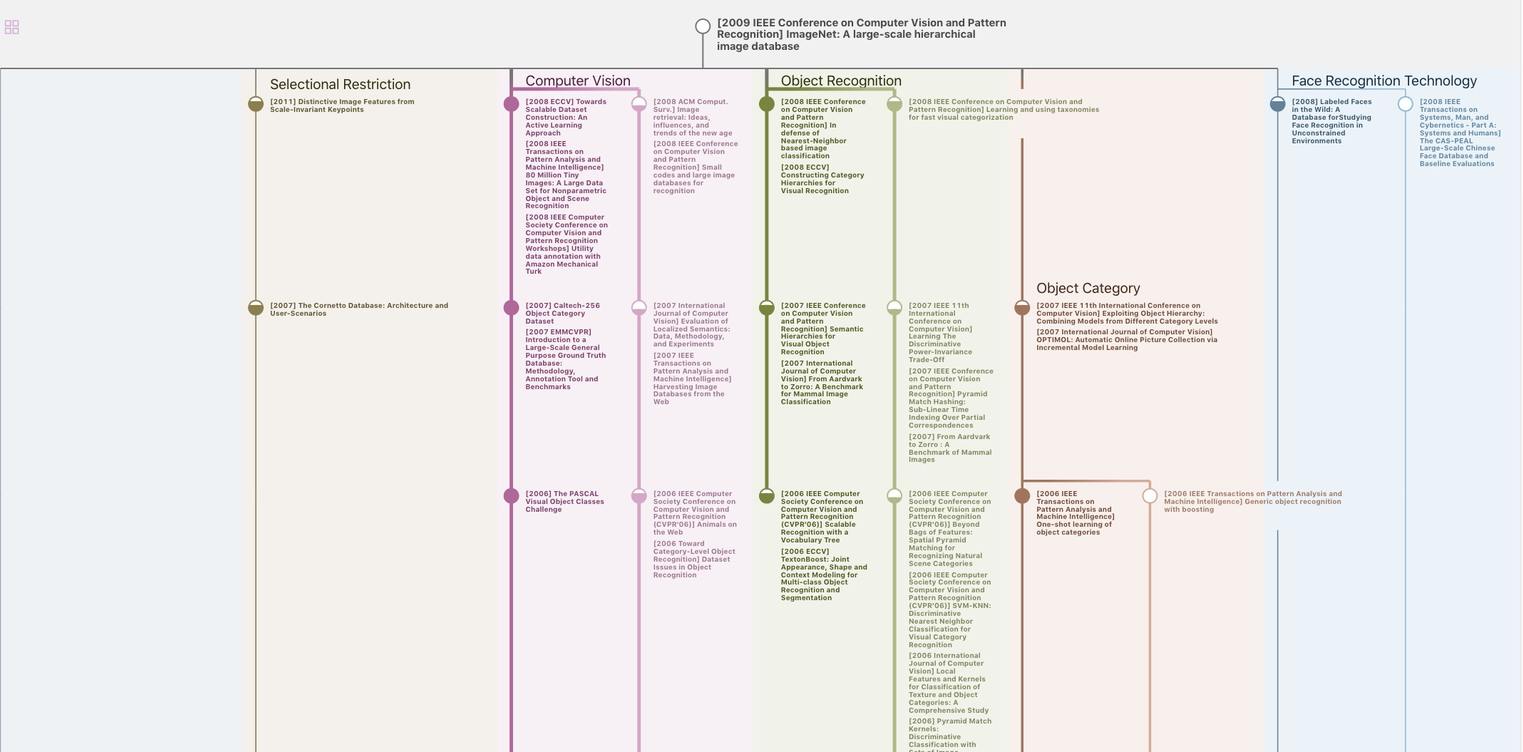
Generate MRT to find the research sequence of this paper
Chat Paper
Summary is being generated by the instructions you defined