Detecting Under-Resolved Flow Physics Using Supervised Machine Learning
AIAA JOURNAL(2023)
摘要
The accuracy of flow simulations is a major concern in computational fluid dynamics (CFD) applications. A possible outcome of inaccuracy in CFD results is missing a major feature in the flowfield. Many methods have been proposed to reduce numerical errors and increase overall accuracy, but these are not always efficient or even feasible. In this study, the principal component analysis (PCA) has been performed on compressible flow simulations around an airfoil to map the high-dimensional CFD data to a lower-dimensional PCA subspace. A machine learning classifier based on the extracted principal components has been developed to detect the simulations that miss the separation bubble behind the airfoil. The evaluative measures indicate that the model is able to detect most of the simulations where the separation region is poorly resolved. Moreover, a single mode responsible for the missing flow separation was uncovered that could be the subject of future studies. The results demonstrate that a machine learning model based on the principal components of the dataset is a promising tool for detecting possible missing flow features in CFD.
更多查看译文
关键词
Machine Learning, Computational Fluid Dynamics, Boundary Layers, Aerodynamic Simulation, Statistical Analysis, Flow Separation, Numerical Analysis, Numerical Simulation, Artificial Intelligence, Data Science
AI 理解论文
溯源树
样例
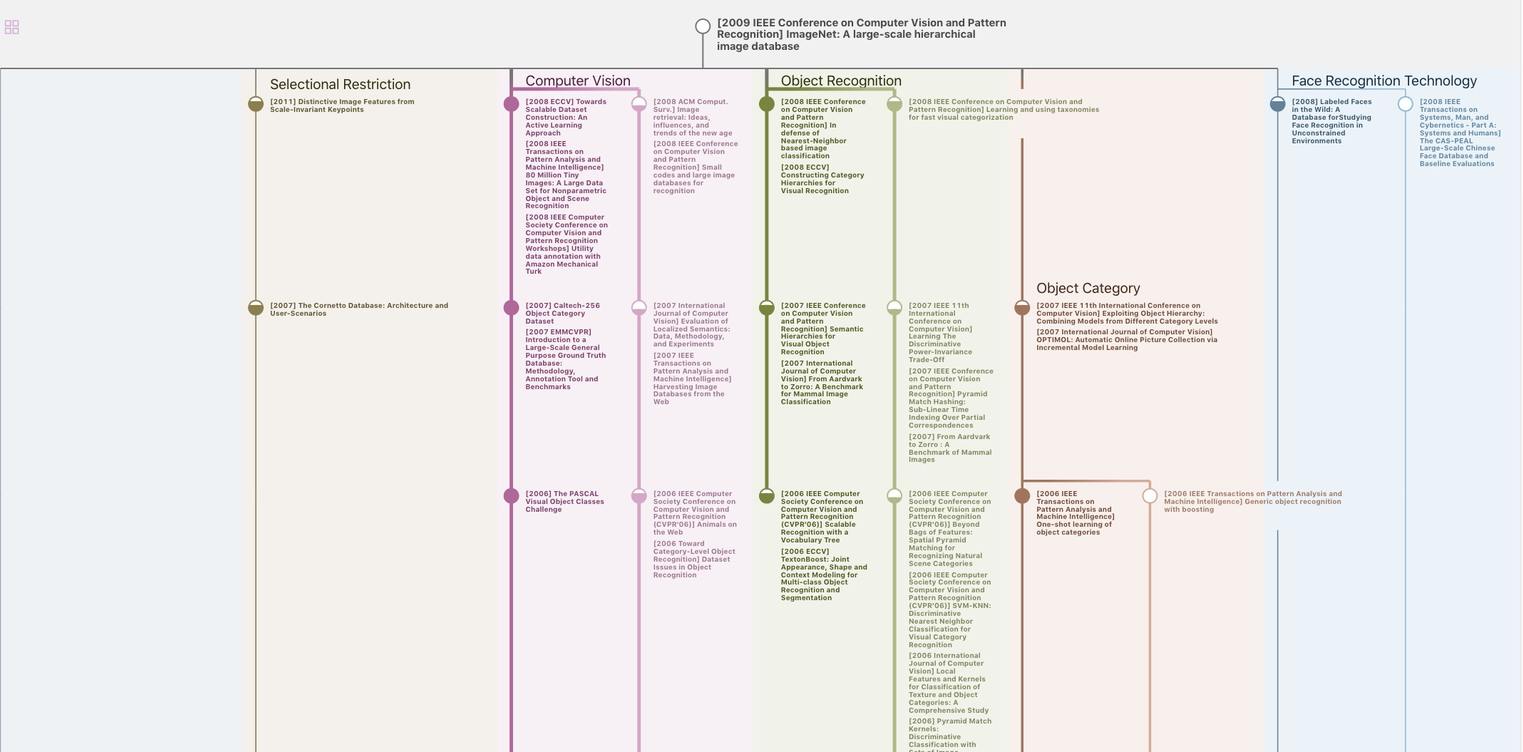
生成溯源树,研究论文发展脉络
Chat Paper
正在生成论文摘要