Towards High-Precision Data Modeling of SHM Measurements Using an Improved Sparse Bayesian Learning Scheme with Strong Generalization Ability
STRUCTURAL HEALTH MONITORING-AN INTERNATIONAL JOURNAL(2024)
关键词
SHM,data modeling,regression and forecasting,an improved sparse Bayesian learning,generalization ability
AI 理解论文
溯源树
样例
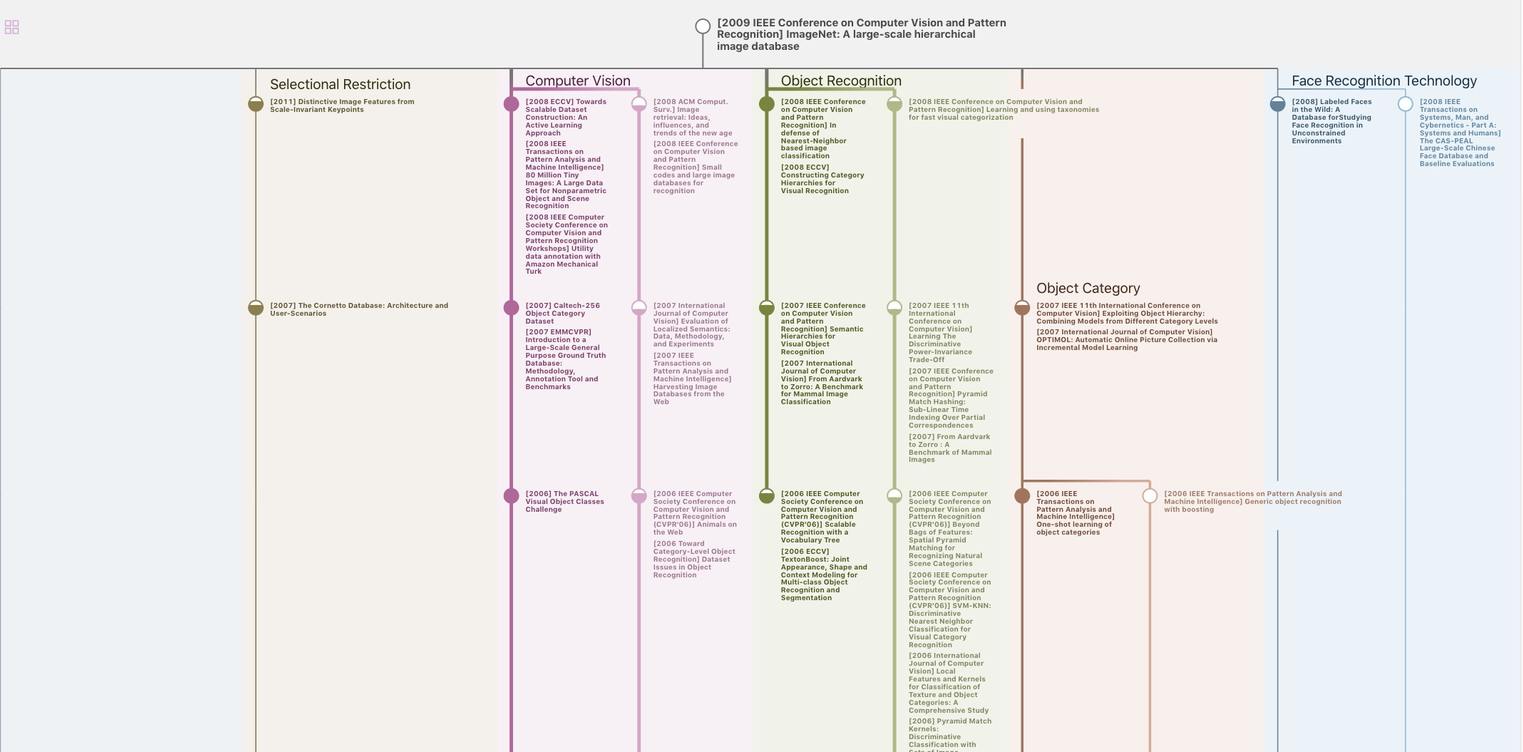
生成溯源树,研究论文发展脉络
Chat Paper
正在生成论文摘要