Lagged Dependent Variable Predictors, Classical Measurement Error, and Path Dependency: The Conditions Under Which Various Estimators are Appropriate
SOCIOLOGICAL METHODS & RESEARCH(2023)
摘要
Lagged dependent variables (LDVs) are often used as predictors in ordinary least squares (OLS) models in the social sciences. Although several estimators are commonly employed, little is known about their relative merits in the presence of classical measurement error and different longitudinal processes. We assess the performance of four commonly used estimators: (1) the standard OLS estimator, (2) an average of past measures (AVG), (3) an instrumental variable (IV) measured at one period previously (IV), and (4) an IV derived from information from more than one time before (IV2). We also propose a new estimator for fixed effects models-the first difference instrumental variable (FDIV) estimator. After exploring the consistency of these estimators, we demonstrate their performance using an empirical application predicting primary school test scores. Our results demonstrate that for a Markov process with classic measurement error (CME), IV and IV2 estimators are generally consistent; LDV and AVG estimators are not. For a semi-Markov process, only the IV2 estimator is consistent. On the other hand, if fixed effects are included in the model, only the FDIV estimator is consistent. We end with advice on how to select the appropriate estimator.
更多查看译文
关键词
dependent variable predictors,various estimators,classical measurement error,path dependency
AI 理解论文
溯源树
样例
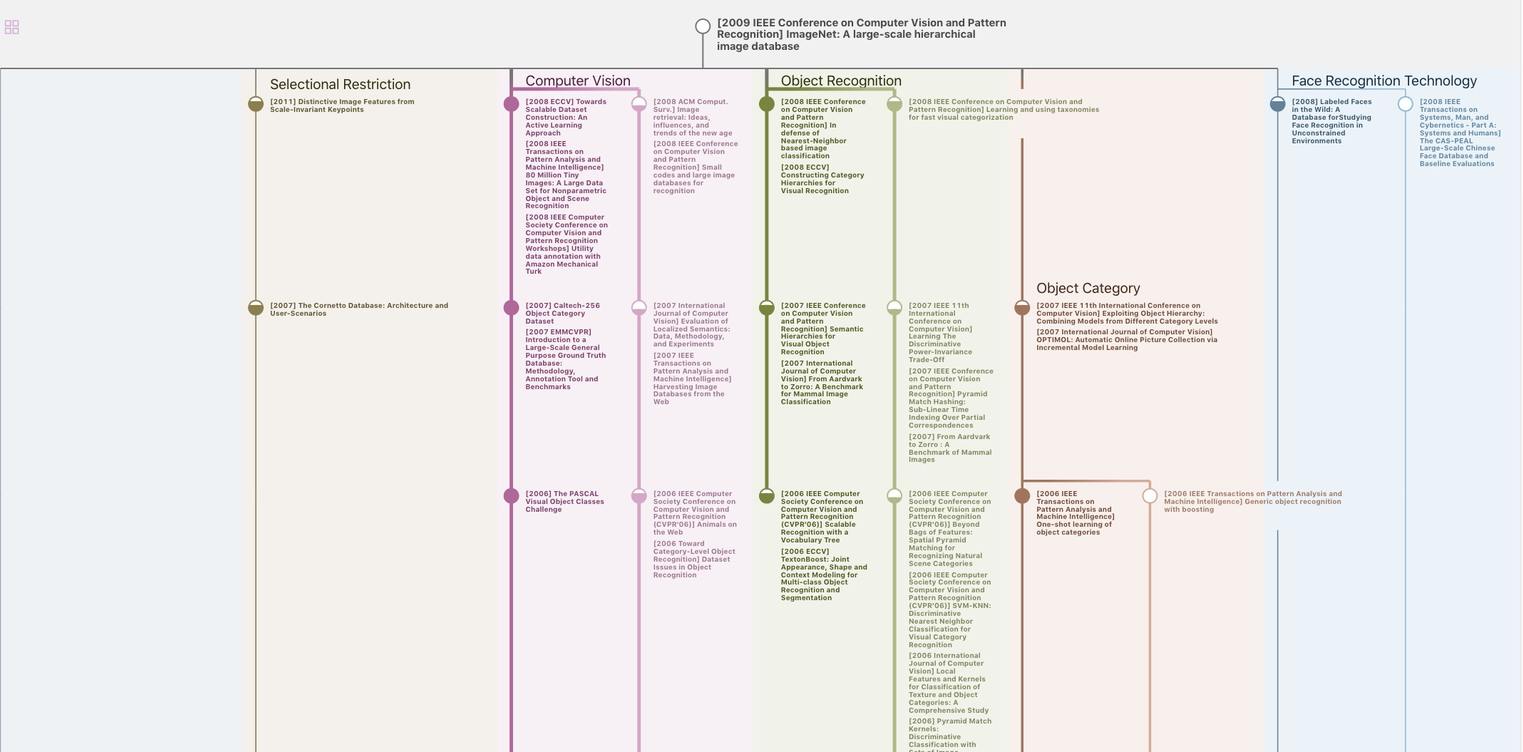
生成溯源树,研究论文发展脉络
Chat Paper
正在生成论文摘要