Symmetry preserving neural network models for spur gear static transmission error curves
MECHANISM AND MACHINE THEORY(2023)
摘要
This paper proposes the use of neural networks to predict static transmission error (STE) curves for spur gears. Initially, a dataset spanning a parametric space of 17 parameters and comprising 20000 STE curves is created with a physics based solver, utilizing a dimensionless formulation. This data is used to train and evaluate different neural network architectures, which incorporate the symmetries of periodicity and input-output interchangeability. Results show that a small fully connected network with 3 hidden layers of 60 neurons can capture the highly non-linear STE response accurately, achieving a mean absolute percentage error of 0.075% on previously unseen data, while the incorporation of symmetries noticeably improves performance. The highest errors are below 1% and occur in border regions of the dataset, where training data is sparse. These results indicate that neural network models can faithfully reproduce the predictions of traditional, non-linear solvers and are thus a promising approach for modeling the static response of spur gears over extended parametric spaces. Finally, indicative dynamic simulations investigate the extension of these results to the dynamic regime.
更多查看译文
关键词
Static transmission error, Spur gears, Surrogate model, Machine learning, Periodic neural network
AI 理解论文
溯源树
样例
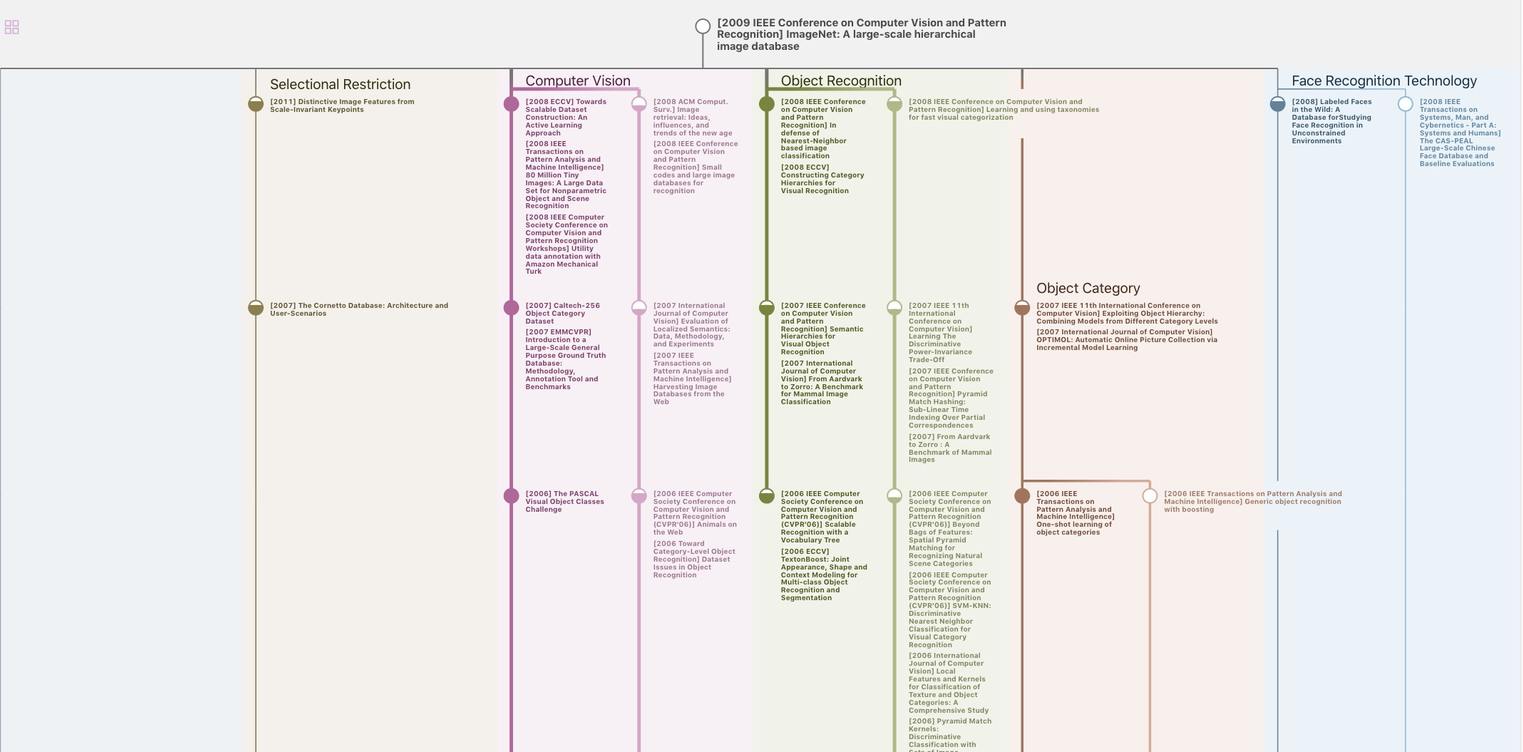
生成溯源树,研究论文发展脉络
Chat Paper
正在生成论文摘要