MARC-Net: Terrain Classification in Parallel Network Architectures Containing Multiple Attention Mechanisms and Multi-Scale Residual Cascades
FORESTS(2023)
摘要
To address the problem that traditional deep learning algorithms cannot fully utilize the correlation properties between spectral sequence information and the feature differences between different spectra, this paper proposes a parallel network architecture land-use classification based on a combined multi-head attention mechanism and multiscale residual cascade called MARC-Net. This parallel framework is firstly implemented by deeply mining the features generated by grouped spectral embedding for information among spectral features by adding a multi-head attention mechanism, which allows semantic features to have expressions from more subspaces while fully considering all spatial location interrelationships. Secondly, a multiscale residual cascade CNN (convolutional neural network) is designed to fully utilize the fused feature information at different scales, thereby improving the network's ability to represent different levels of information. Lastly, the features obtained by the multi-head attention mechanism are fused with those obtained by the CNN, and the merged resultant features are downgraded through the fully connected layer to obtain the classification results and achieve pixel-level multispectral image classification. The findings show that the algorithm proposed in this paper has an aggregate precision of 97.22%, compared to that of the Vision Transformer (ViT) with 95.08%; its performance on the Sentinel-2 dataset shows a huge improvement. Moreover, this article mainly focuses on the change rate of forest land in the study area. The Forest land area was 125.1143 km(2) in 2017, 105.6089 km(2) in 2019, and 76.3699 km(2) in 2021, with an increase of 15.59%, an decrease of 0.97%, and increase of 14.76% in 2017-2019, 2019-2021 and 2017-2021, respectively.
更多查看译文
关键词
MARC-Net, GSE, residual cascade, semantic features
AI 理解论文
溯源树
样例
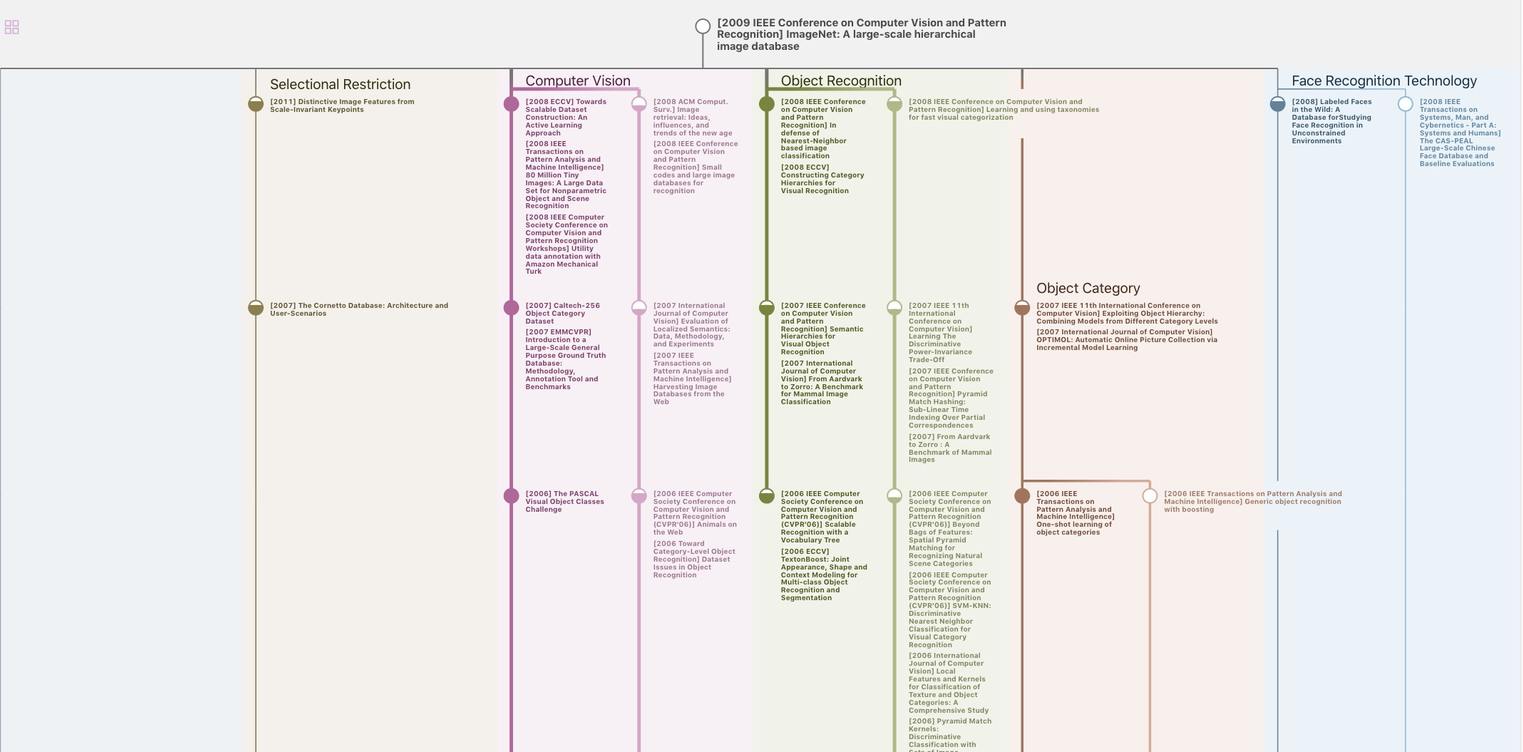
生成溯源树,研究论文发展脉络
Chat Paper
正在生成论文摘要