Enhancement of Predictive Bayesian Network Model for Corrosion Alarm of Steel Reinforcement with Uncertainty of NDT Measurements
JOURNAL OF NONDESTRUCTIVE EVALUATION(2023)
Abstract
In this paper, a methodology based on a Bayesian Network (BN) is proposed to create reliable corrosion alarm maps of first layer reinforcements, which is related to corrosion levels. The methodology consists of three principal steps: building the database, learning the BN structure and its parameters from non-destructive testing (NDT) data, and using the BN for corrosion alarm mapping. Uncertainty of the on-site measurement is accounted in the predictive model to enhance accuracy of the obtained corrosion alarm probability. The parameters of the physically optimal BN structure are corrosion potential, corrosion rate, electrical resistivity, and corrosion alarm. NDT inspection data of a bridge pier face (1.3 m wide and 2 m high) is used as new information for updating the BN in the last step. The methodology allows the probability of corrosion alarm to be estimated where the NDT indicators are not simultaneously available. Accounting the uncertainty of measurement in the BN model allows more nuanced estimates of the corrosion alarm probability, and decrease the number of points for which the probability of a corrosion alarm is 50% (undetermined state between “alarm” and “no alarm”).
MoreTranslated text
Key words
corrosion alarm,predictive bayesian network model,steel reinforcement,uncertainty
AI Read Science
Must-Reading Tree
Example
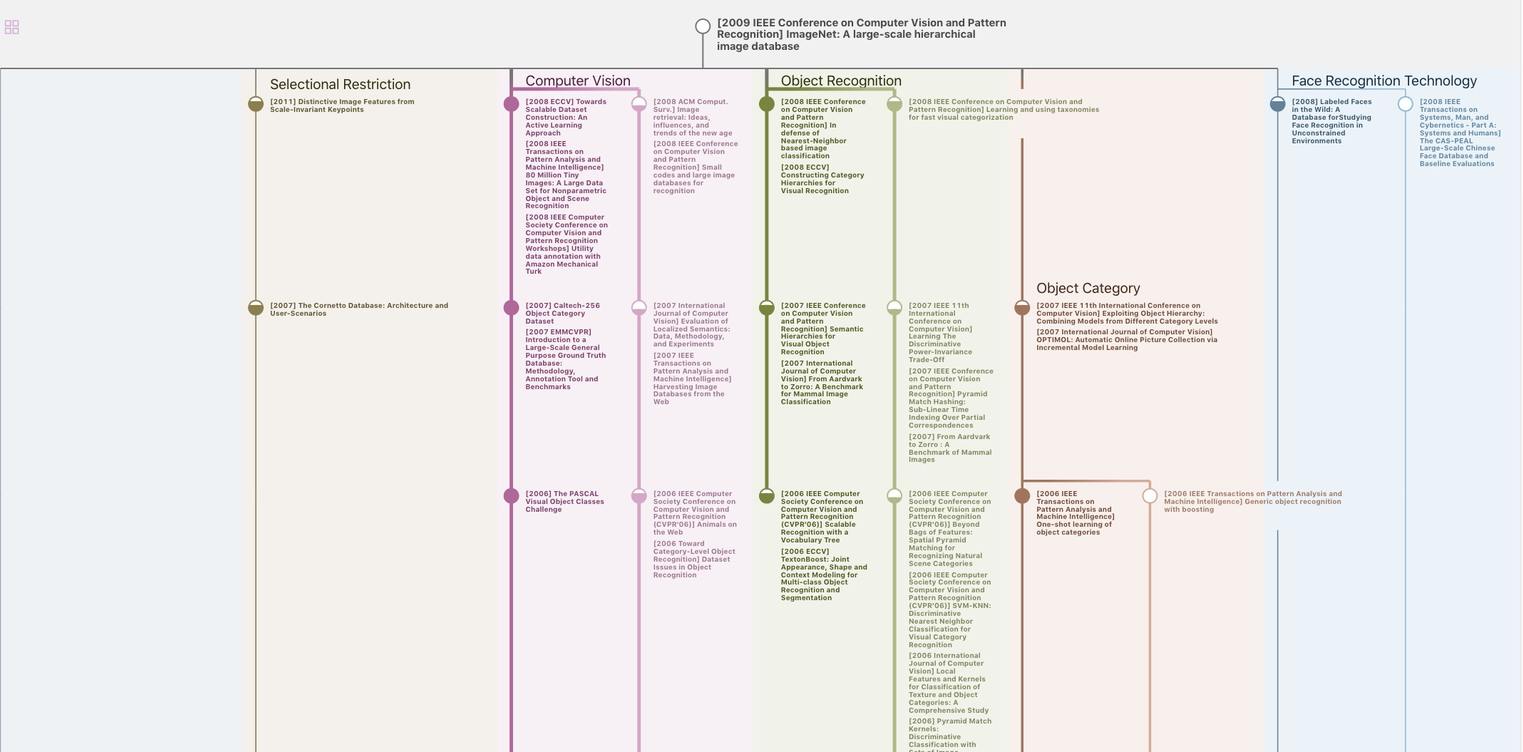
Generate MRT to find the research sequence of this paper
Chat Paper
Summary is being generated by the instructions you defined