Error revision during morning period for deep learning and multi-variable historical data-based day-ahead solar irradiance forecast: towards a more accurate daytime forecast
EARTH SCIENCE INFORMATICS(2023)
摘要
With the increasing proportion of solar energy in the energy system, accurate solar irradiance forecast is of great significance for low-cost energy scheduling. This paper proposes a new forecasting idea for day-ahead solar irradiance forecast on the day-ahead scale: Firstly, based on formula derivation and big data correlation analysis, this paper finds out multiple parameters related to GHI, and jointly uses these parameters to forecast GHI. Error revision during morning period (ERDMP) is innovatively proposed on this basis, towards a more accurate daytime forecast. In order to prove the reliability and universality of the method, relevant data from five different-climatic regions are respectively used in the experiment. The multi-variable historical data-based day-ahead solar irradiance forecast uses deep neural networks, including Recurrent Neural Network (RNN), Convolutional Neural Network (CNN) and Long Short-Term Memory (LSTM) network. ERDMP uses a linear AutoRegressive (AR) model to predict the daytime error coefficients based on the morning error coefficients. According to the results, through the proposed ERDMP, Mean Absolute Error (MAE) decreases by about 25% to 30%, Root Mean Squared Error (RMSE) decreases by about 20%, and R 2 increases by about 5% to 10% when compared with the initial error of multi-parameter prediction models and other advanced models.
更多查看译文
关键词
Deep learning,Error revision during morning period,Solar irradiance forecast,Recurrent neural network,Solar energy
AI 理解论文
溯源树
样例
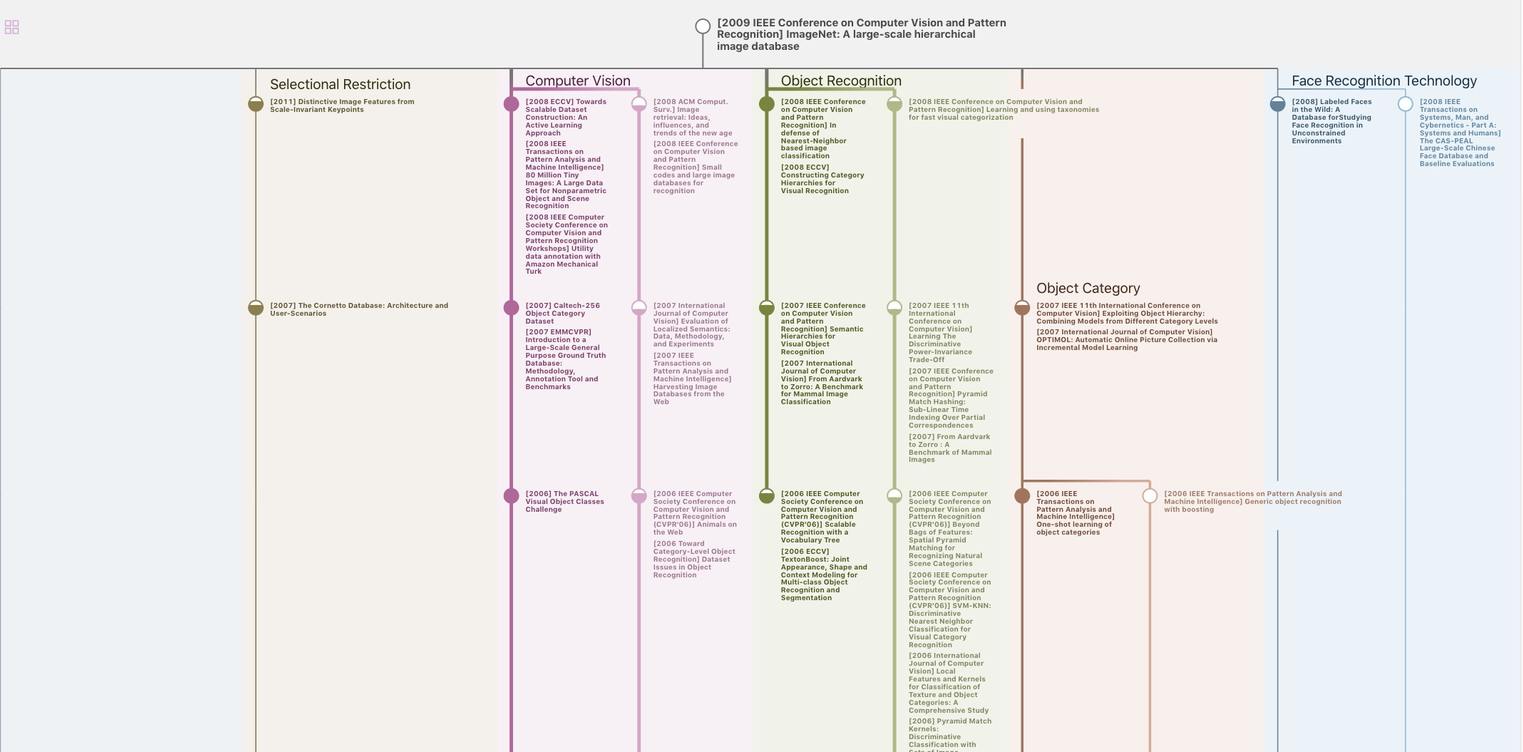
生成溯源树,研究论文发展脉络
Chat Paper
正在生成论文摘要