A feature enhancement loss for person re-identification
SYSTEMS SCIENCE & CONTROL ENGINEERING(2023)
摘要
The goal of person re-identification (ReID) is to recognize the same person across cameras. Classification loss is one of the most widely used objective functions in person ReID tasks based on deep learning. However, the features, which are learned with the classification loss, are not sufficiently discriminative enough when they are close to the origin. In this study, we propose a feature enhancement loss to move features of person images away from the origin. During training, our proposed method adds a regularization item to avoid the feature vector near the origin point. Our method was evaluated on two benchmark person ReID benchmark datasets, Market1501 and DukeMTMC-reID. Results show that the proposed method outperforms the state-of-the-art method by 0.9% and 1.2% on rank-1 accuracy and mean average precision (mAP) index on Market-1501, 1.0% and 1.4% on rank-1 accuracy and mAP index on DukeMTMC-reID. This means that when learning features with a classification loss, making the features far away from the origin point is meaningful.
更多查看译文
关键词
feature enhancement loss,person,re-identification
AI 理解论文
溯源树
样例
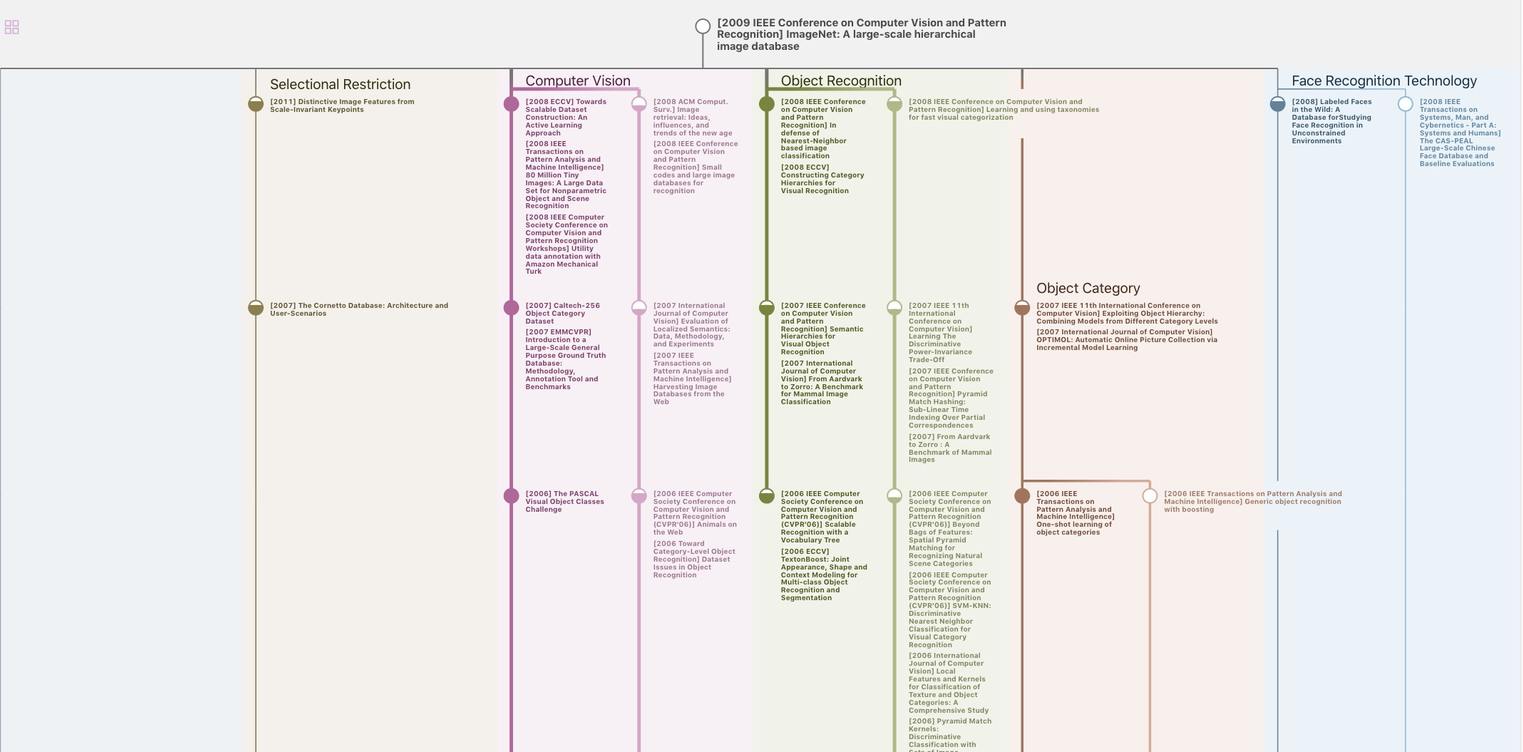
生成溯源树,研究论文发展脉络
Chat Paper
正在生成论文摘要