Recognition of field roads based on improved U-Net plus plus Network
INTERNATIONAL JOURNAL OF AGRICULTURAL AND BIOLOGICAL ENGINEERING(2023)
Abstract
Unmanned driving of agricultural machinery has garnered significant attention in recent years, especially with the development of precision farming and sensor technologies. To achieve high performance and low cost, perception tasks are of great importance. In this study, a low-cost and high-safety method was proposed for field road recognition in unmanned agricultural machinery. The approach of this study utilized point clouds, with low-resolution Lidar point clouds as inputs, generating high-resolution point clouds and Bird's Eye View (BEV) images that were encoded with several basic statistics. Using a BEV representation, road detection was reduced to a single-scale problem that could be addressed with an improved U -Net++ neural network. Three enhancements were proposed for U-Net++: 1) replacing the convolutional kernel in the original U -Net++ with an Asymmetric Convolution Block (ACBlock); 2) adding a multi-branch Asymmetric Dilated Convolutional Block (MADC) in the highest semantic information layer; 3) adding an Attention Gate (AG) model to the long-skip-connection in the decoding stage. The results of experiments of this study showed that our algorithm achieved a Mean Intersection Over Union of 96.54% on the 16-channel point clouds, which was 7.35 percentage points higher than U-Net++. Furthermore, the average processing time of the model was about 70 ms, meeting the time requirements of unmanned driving in agricultural machinery. The proposed method of this study can be applied to enhance the perception ability of unmanned agricultural machinery thereby increasing the safety of field road driving.
MoreTranslated text
Key words
image segmentation, unmanned agricultural machinery, field roads, point cloud super-resolution, point cloud bird's eye view
AI Read Science
Must-Reading Tree
Example
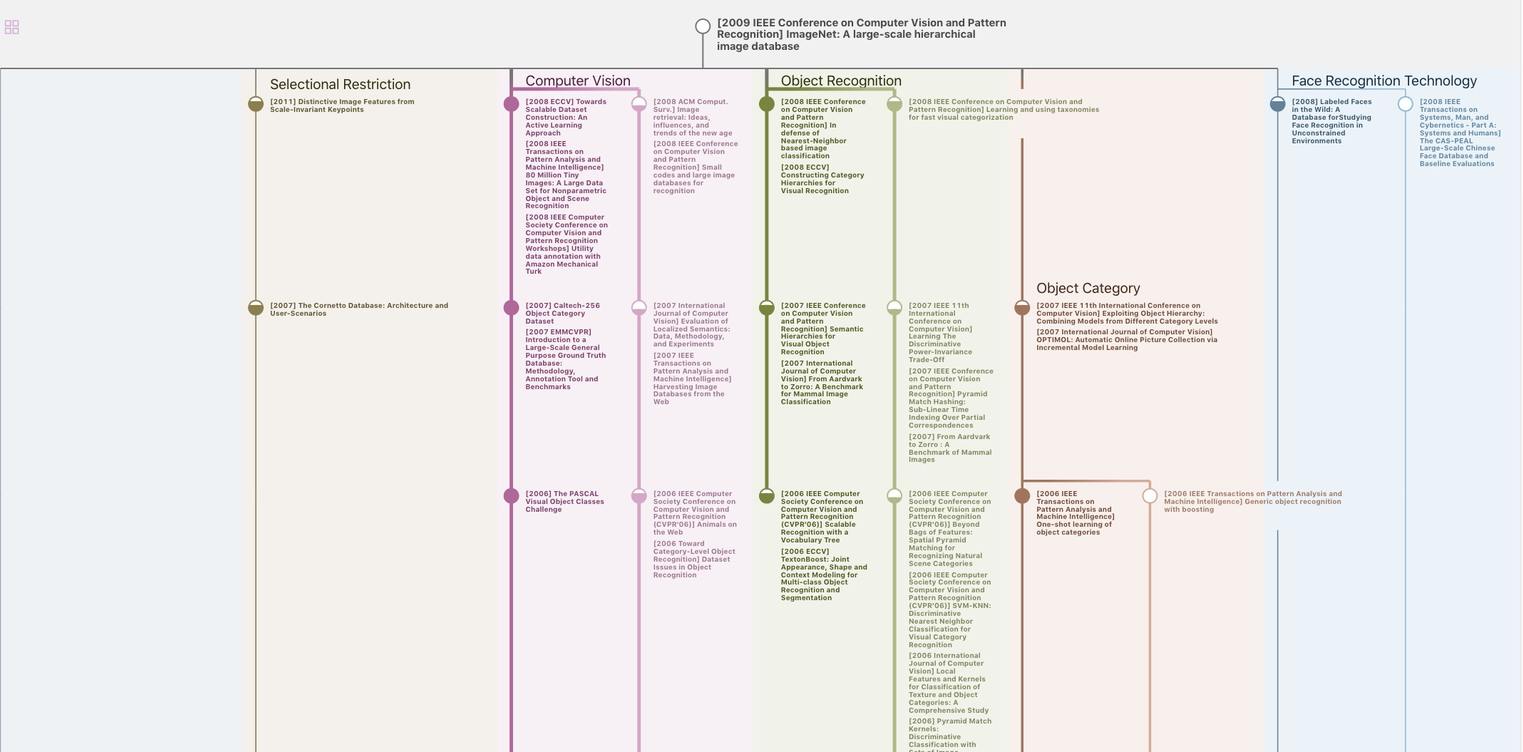
Generate MRT to find the research sequence of this paper
Chat Paper
Summary is being generated by the instructions you defined