Infilling missing data and outliers for a conventional sewage treatment plant using a self-organizing map: a case study of Kauma Sewage Treatment Plant in Lilongwe, Malawi
H2OPEN JOURNAL(2023)
摘要
Data availability is key for modeling of wastewater treatment processes. However, process data are characterized by missing values and outliers. This study applied a self-organizing map (SOM), to fill in missing values and replace outliers in wastewater treatment data from Kauma Sewage Treatment Plant in Lilongwe, Malawi. We used primary and secondary wastewater data and executed the SOM algorithm to fill missing values and replace outliers in effluent pH, biochemical oxygen demand, and dissolved oxygen. The results suggest that SOM algorithm is reliable in filling gaps in wastewater time series data with less than 50% missing values with correlation coefficient (R) values of >0.90. The SOM algorithm failed to reliably fill gaps and replace outliers in time series data with >50% missing values. For instance, high mean square error (MSE) values of 3,655.57, 10.62, and 2,153.34 for pH, DO, and BOD, respectively, were registered in datasets with more than 50% missing values, while very small MSE values (MSE approximate to 0) were associated with effluent pH, BOD, and DO data with missing values of >50%. Practitioners can use this approach to improve the planning and management of wastewater treatment facilities where available data records are riddled with missing observations.
更多查看译文
关键词
kauma sewage treatment plant,conventional sewage treatment plant,outliers,malawi,self-organizing
AI 理解论文
溯源树
样例
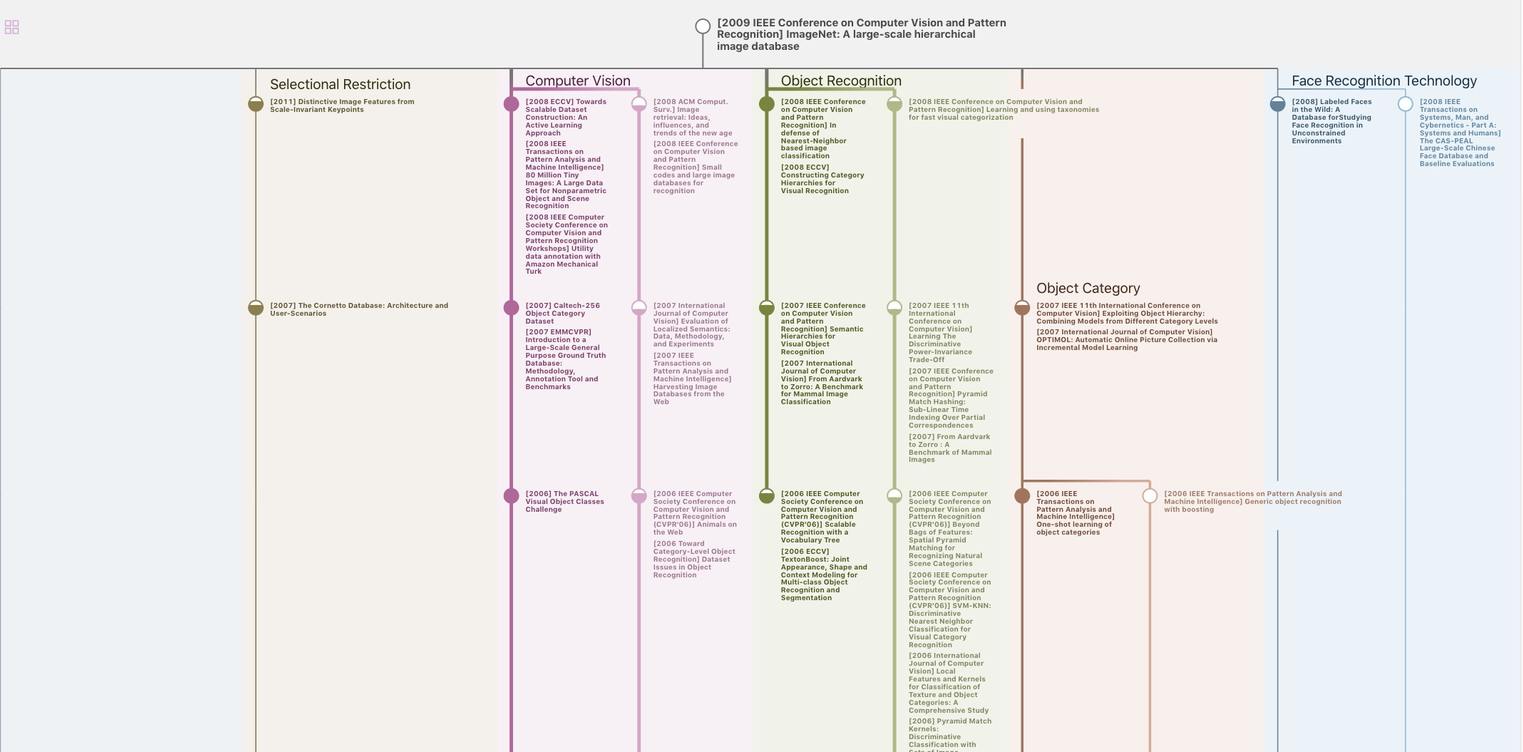
生成溯源树,研究论文发展脉络
Chat Paper
正在生成论文摘要