Robust full-waveform inversion based on automatic differentiation and differentiable dynamic time warping
JOURNAL OF GEOPHYSICS AND ENGINEERING(2023)
摘要
Full waveform inversion is a methodology that determines high-resolution parameters. The widely used L-2-norm misfit function has local minima if the low wavenumber components are not accurate. Suffering from a cycle skipping problem, the solution of waveform inversion will be trapped in the local minima. Dynamic time warping aims to find an optimal alignment between two signals, which is a more robust measure to avoid cycle-skipping challenges. However, the discontinuity makes the conventional dynamic time warping distance unsuitable for waveform inversion. We introduce a soft dynamic time warping distance as the misfit function, which is differentiable in that the inverted solution can converge to the accurate global minimum. We compare the convexity of the L-2-norm and soft dynamic time warping distance and show that the soft dynamic time warping distance has a wider convexity range with different time shift and amplitudes. It can alleviate the half-wavelength limitation of the conventional L-2-norm. We calculate the gradient using the automatic differentiation technique and the minibatch strategy and then analyse the alignment paths of different smooth parameters. A significant smooth parameter gamma makes the Soft-DTW distance tending to the L-2-norm, which generates new local minima. We recommend a small smooth parameter to ensure the convexity of the Soft-DTW distance. Numerical examples show that the soft dynamic time warping can effectively reconstruct the deep velocity parameters of the BG Compass and Marmousi models with noise robustness and lower dependence on the initial model.
更多查看译文
关键词
differentiable dynamic time warping,full waveform inversion,automatic differentiation,minibatch,cycle skipping
AI 理解论文
溯源树
样例
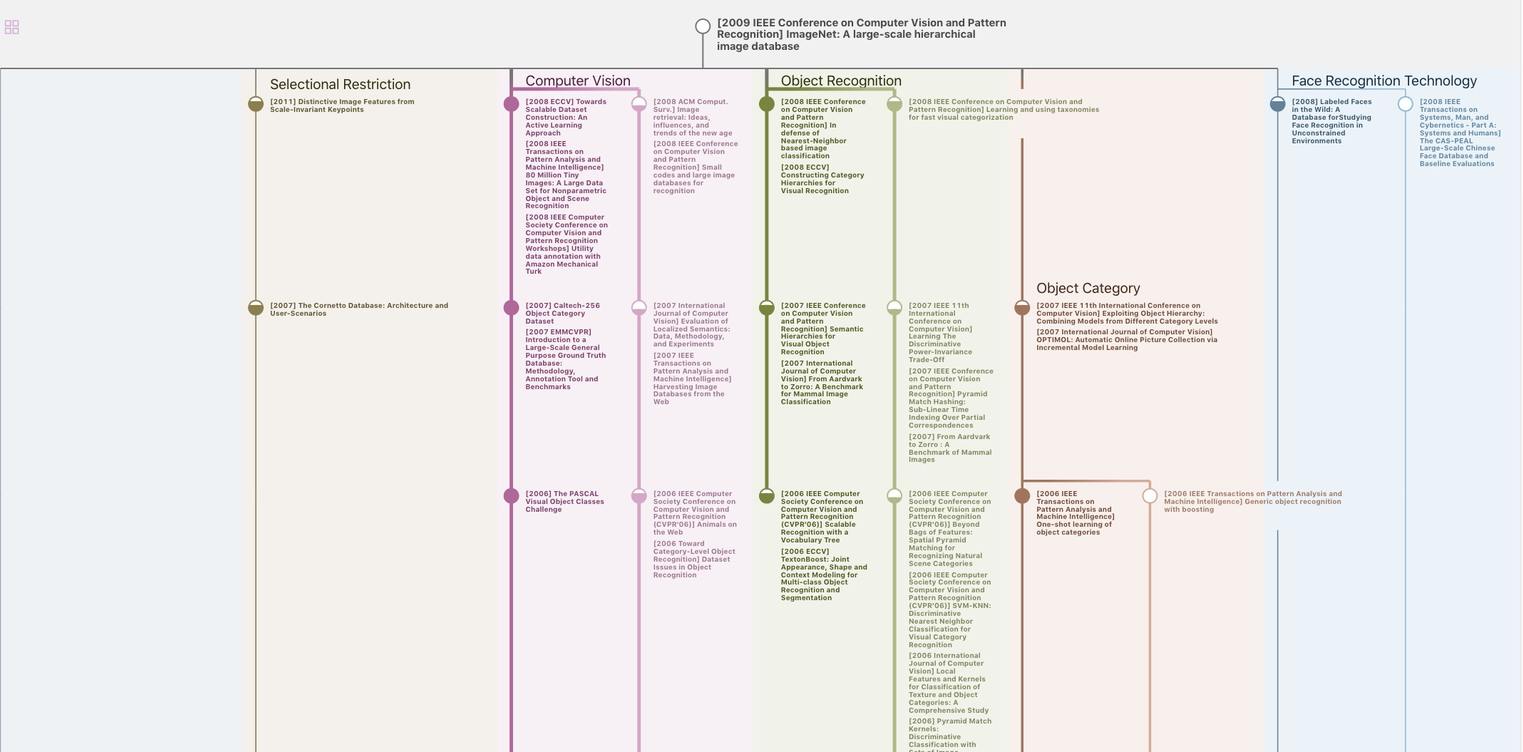
生成溯源树,研究论文发展脉络
Chat Paper
正在生成论文摘要