FFRNet: DCNN for Real-Time Distracted Driving Detection Toward Embedded Deployment
IEEE TRANSACTIONS ON INTELLIGENT TRANSPORTATION SYSTEMS(2023)
摘要
Real-time running deep convolutional neural networks on embedded electronics is one recent focus for distracted driving detection. In this work, we proposed FRNet, which is a unique, efficient, and real-time architecture. The FRNet converts spatially distributed features into depth distribution by a feature reorganization block. This block compresses the volume of backbone, reduces the memory read/write volumes along with multiply-accumulate operations, and extracts key features faster. In addition, a ultra-lightweight backbone was designed, with an atypical reshape strategy. This atypical strategy designed based on pixel-level analysis, for compensating the accuracy decline along with feature reorganization. The proposed FRNet (A) over cap offered excellentA real-time performance on low power embedded platforms, and has a competitive accuracy with previous state-of-the-art models. It achieved 97.55% accuracy on the SFD+AUCDD-V1, and 99.86% on 3MDAD. On an automotive-grade embedded demo board, it costs 16.93 ms per frame and achieved 59 FPS. As of today, this is the fastest record for end-to-end distraction detection. Experiments revealed (A) over cap the real-time and accuracy are best balanced with FRNet. The model is publicly available at https://github.com/congduan-HNU/FRNet.
更多查看译文
关键词
Feature extraction,Real-time systems,Vehicles,Sensors,Biological system modeling,Manuals,Deep learning,DCNN,distracted driver detection,key features,model compression,real-time embedded deployment
AI 理解论文
溯源树
样例
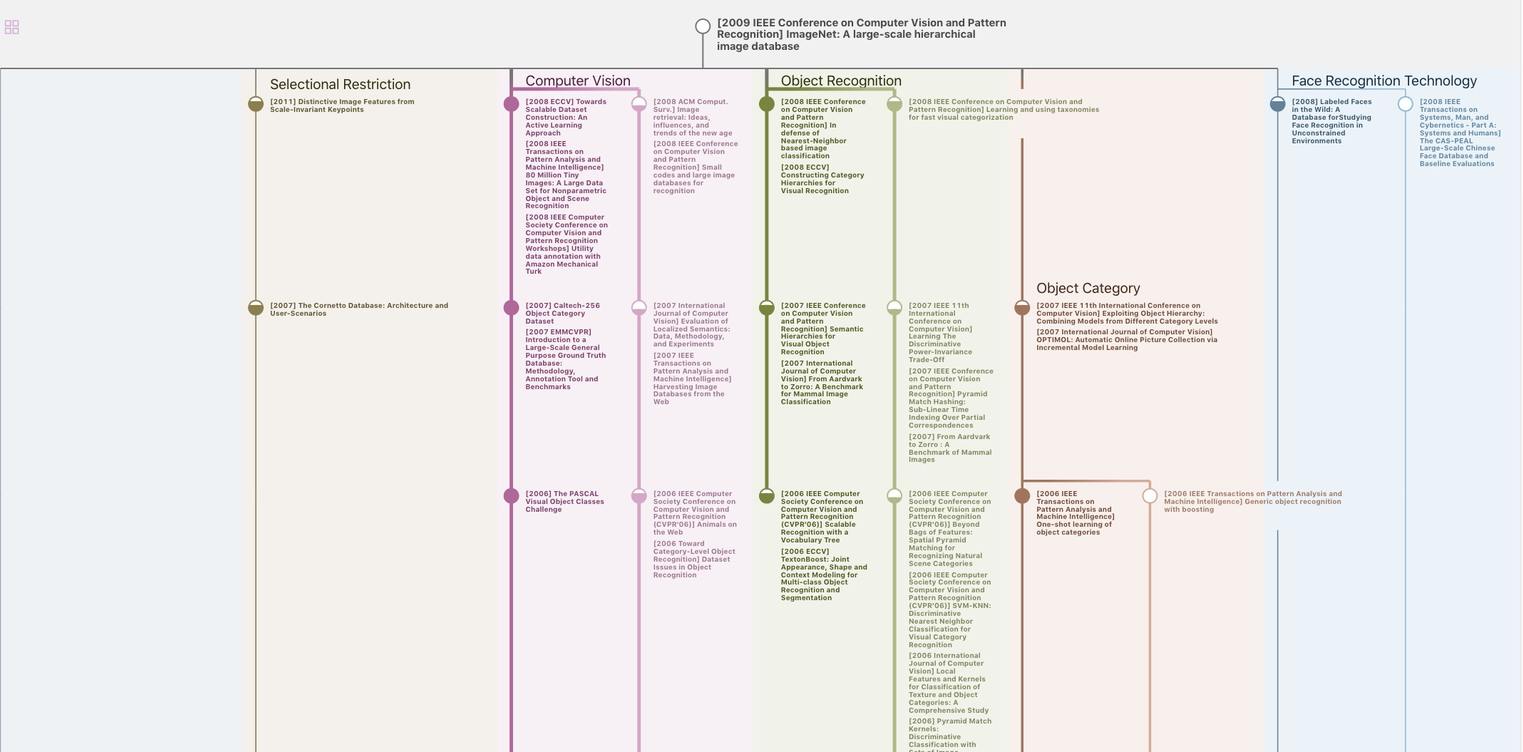
生成溯源树,研究论文发展脉络
Chat Paper
正在生成论文摘要