Applying Machine Learning Methods to Improve All-Terminal Network Reliability
2023 ANNUAL RELIABILITY AND MAINTAINABILITY SYMPOSIUM, RAMS(2023)
Abstract
SUMMARY & CONCLUSIONSOne essential task in practice is to quantify and improve the reliability of an infrastructure network in terms of the connectivity of network components (i.e., all-terminal reliability). However, as the number of edges and nodes in the network increases, computing the all-terminal network reliability using exact algorithms becomes prohibitive. This is extremely burdensome in network designs requiring repeated computations. In this paper, we propose a novel machine learning-based framework for evaluating and improving all-terminal network reliability using Deep Neural Networks (DNNs) and Deep Reinforcement Learning (DRL). With the help of DNNs and Stochastic Variational Inference (SVI), we can effectively compute the all-terminal reliability for different network configurations in DRL. Furthermore, the Bayesian nature of the proposed SVI+DNN model allows for quantifying the estimation uncertainty while enforcing regularization and reducing overfitting. Our numerical experiment and case study show that the proposed framework provides an effective tool for infrastructure network reliability improvement.
MoreTranslated text
Key words
reliability models, reliability growth analysis, deep reinforcement learning
AI Read Science
Must-Reading Tree
Example
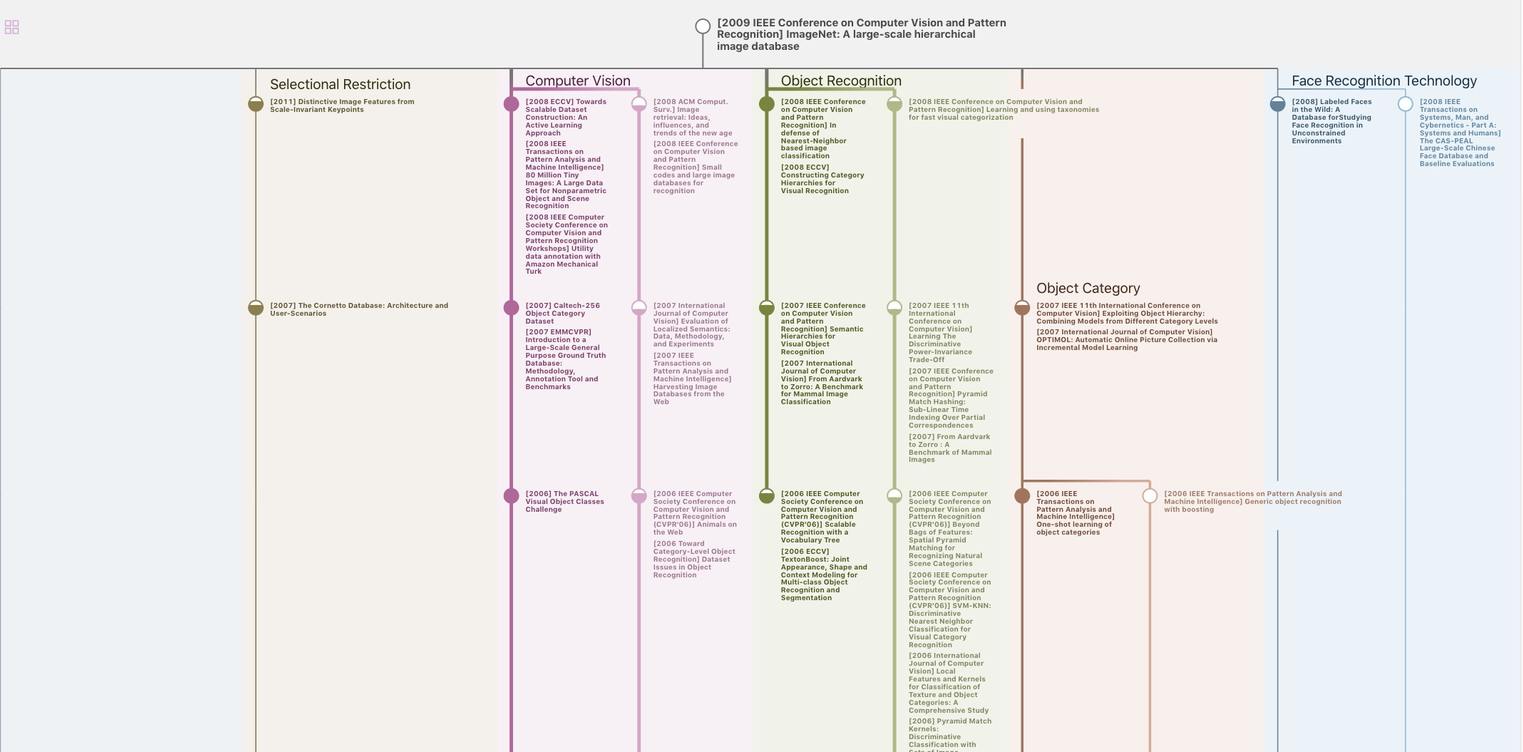
Generate MRT to find the research sequence of this paper
Chat Paper
Summary is being generated by the instructions you defined