Improved duelling deep Q-networks based path planning for intelligent agents
INTERNATIONAL JOURNAL OF VEHICLE DESIGN(2023)
摘要
The natural deep Q-network (DQN) usually requires a long training time because the data usage efficiency is relatively low due to uniform sampling. Importance sampling (IS) can promote important experiences and is more efficient in the neural network training process. In this paper, an efficient learning mechanism using the IS technique is incorporated into duelling DQN algorithm, and is further applied to path planning task for an agent. Different from the traditional DQN algorithm, proposed algorithm improves the sampling efficiency. In this experiment, four target points on the map are deployed to evaluate the loss and the accumulated reward. Simulations and comparisons in various simulation situations demonstrate the effectiveness and superiority of the proposed path planning scheme for an intelligent agent.
更多查看译文
关键词
path planning,DQNs,deep Q-networks,reinforcement learning,importance sampling
AI 理解论文
溯源树
样例
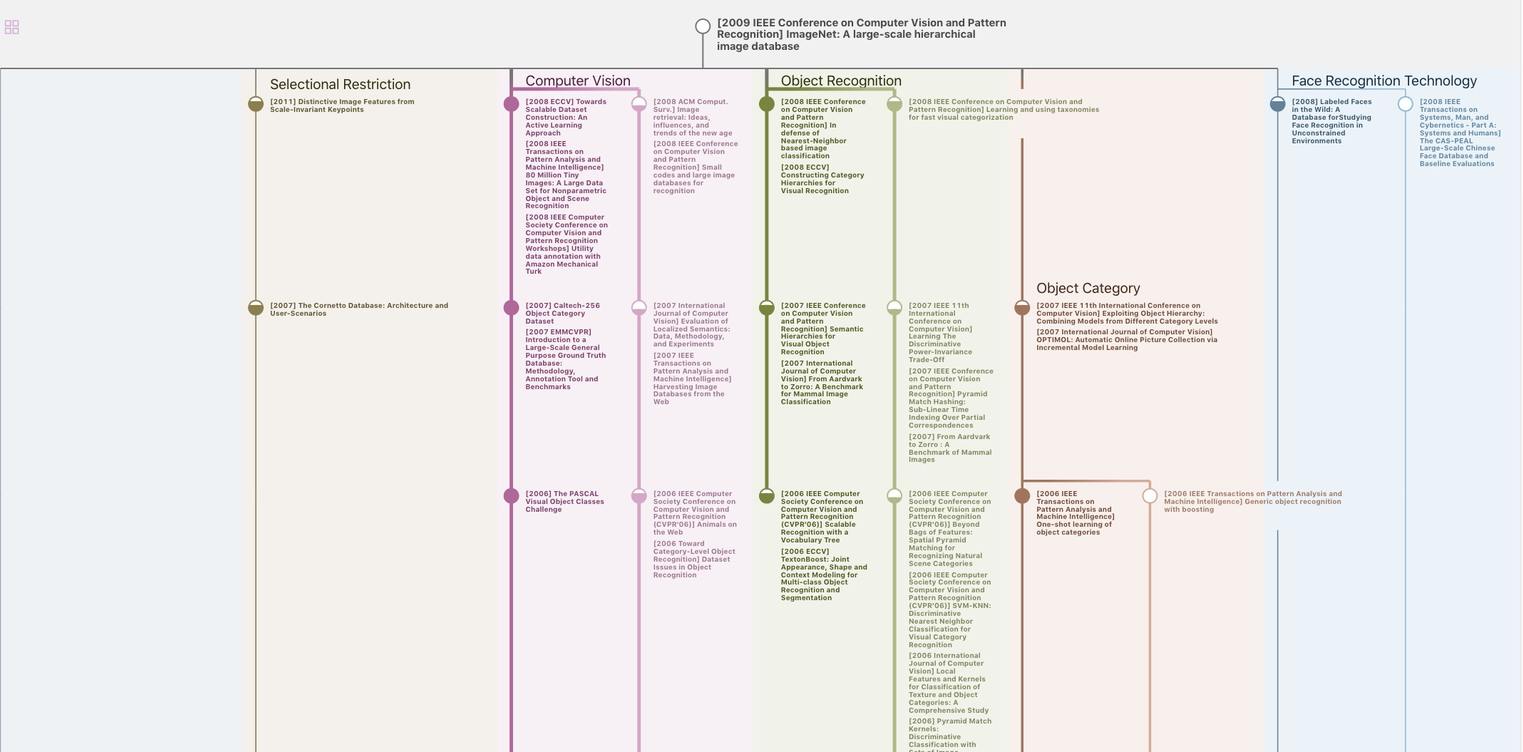
生成溯源树,研究论文发展脉络
Chat Paper
正在生成论文摘要