Implement the Materials Genome Initiative: Machine Learning Assisted Fluorescent Probe Design for Cellular Substructure Staining
ADVANCED MATERIALS TECHNOLOGIES(2023)
Abstract
The Materials Genome Initiative (MGI) is accelerating the pace of advanced materials development by integrating high-throughput experimentation, database construction, and intelligence computation. Live-cell imaging agents, such as fluorescent dyes, are exemplary candidates for MGI applications for two reasons: i) they are essential in visualizing cellular structures and functional processes, and ii) the unclear relationship between the chemical structure of fluorescent dyes and their live-cell imaging properties severely restricts the current trial-and-error dye development. Herein, the MGI is followed to present an intelligent combinatorial methodology for predicting the staining cell ability of dyes utilizing machine learning (ML) driven by a structurally diverse combinatorial library. This study demonstrates how to high-throughput synthesize 1,536 dyes and evaluate their imaging properties to establish a feature dataset for ML. A set of high-precision ML-predictors is then successfully modeled for assisting live-cell staining and endoplasmic reticulum judgment. This approach is believed to bridge the gap between dye structure and corresponding staining behavior, and can accelerate the discovery of novel organelle-specific stains.
MoreTranslated text
Key words
combinatorial library,fluorescent dyes,live-cell imaging,materials genomes,machine learning
AI Read Science
Must-Reading Tree
Example
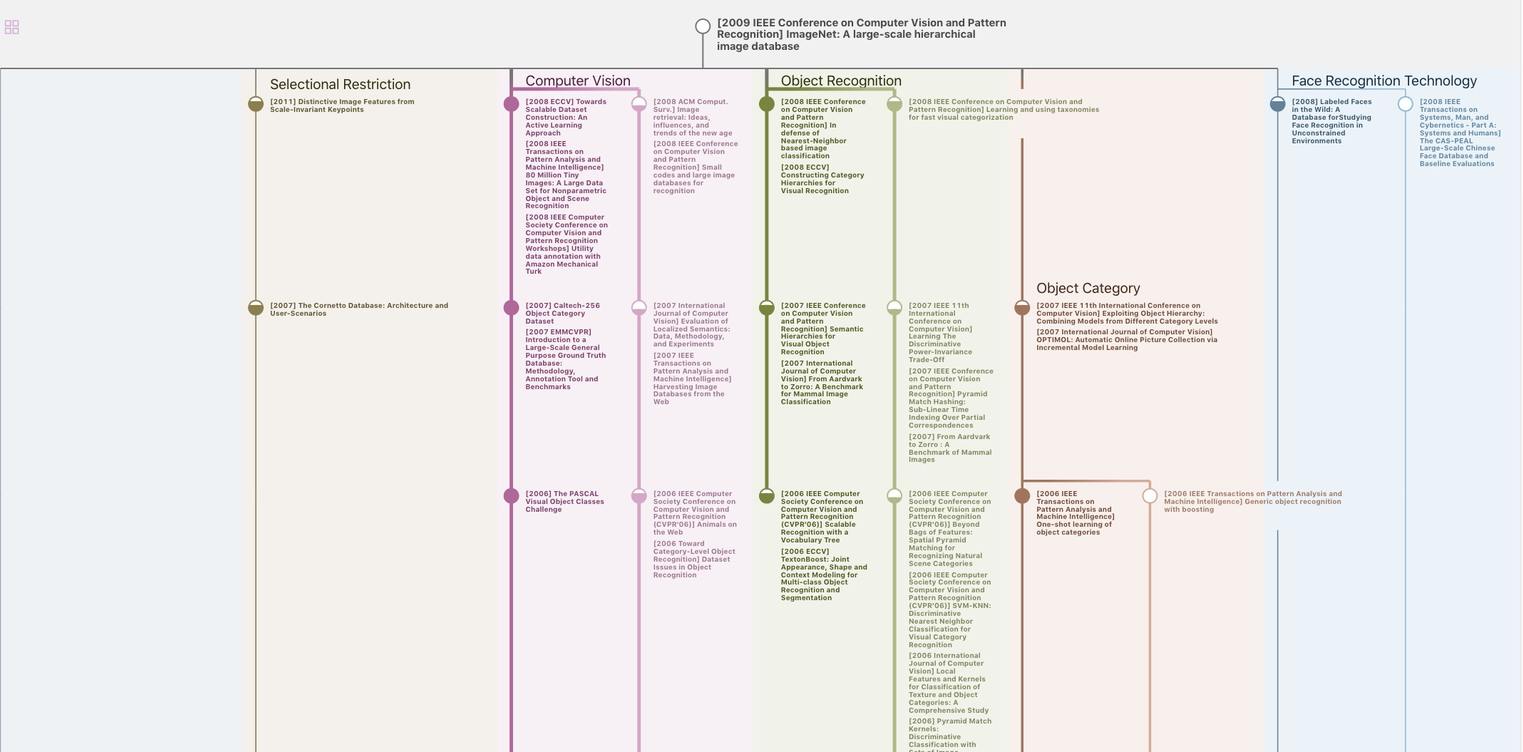
Generate MRT to find the research sequence of this paper
Chat Paper
Summary is being generated by the instructions you defined