Milling tool wear prediction: optimized long short-term memory model based on attention mechanism
FERROELECTRICS(2023)
摘要
To improve the prediction accuracy of milling tool wear, a prediction method based on Attention-LSTM is proposed. In the training phase, first, the data are pre-processed by truncation, downsampling, and the Hampel filtering method, and then features are extracted by the time domain, frequency domain, and time-frequency domain analysis methods. Second, a deep neural network is designed to describe the complex nonlinear function between features and tool wear. Last, aiming at the insufficient prediction accuracy due to the LSTM lacking feature extraction and enhancement, the Attention mechanism is introduced to optimize the model. The results suggest that this prediction method provides an efficient strategy for milling tool wear prediction.
更多查看译文
关键词
memory,prediction,tool,attention,short-term
AI 理解论文
溯源树
样例
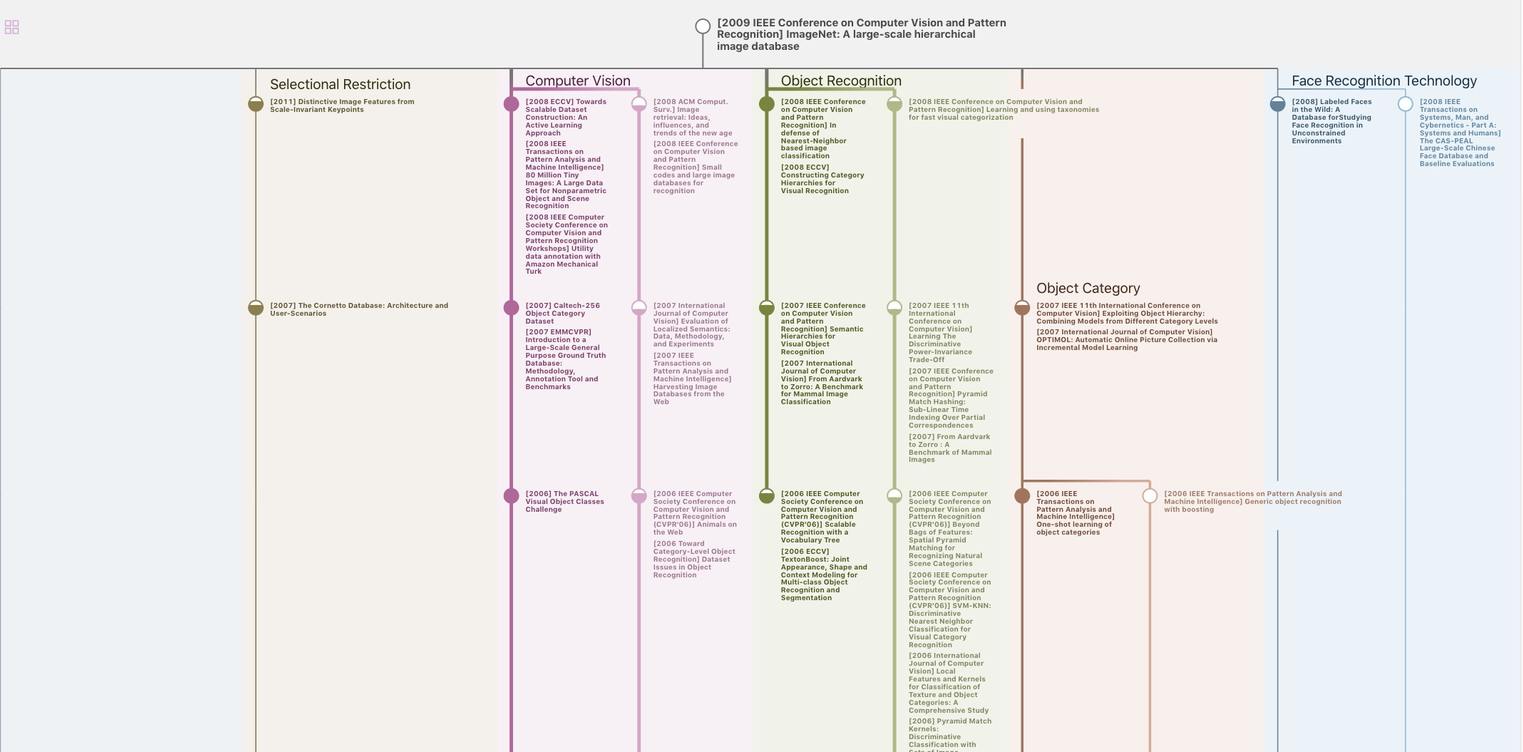
生成溯源树,研究论文发展脉络
Chat Paper
正在生成论文摘要