Learning run-and-tumble chemotaxis with support vector machines
EPL(2023)
摘要
- To navigate in spatial fields of sensory cues, bacterial cells employ gradient sensing by temporal comparison for run-and-tumble chemotaxis. Sensing and motility noise imply trade-off choices between precision and accuracy. To gain insight into these trade-offs, we learn optimal chemotactic decision filters using supervised machine learning, applying support vector machines to a biologically motivated training dataset. We discuss how the optimal filter depends on the level of sensing and motility noise, and derive an empirical power law for the optimal measurement time Teff similar to D-alpha rot with alpha = 0.2, ... , 0.3 as a function of the rotational diffusion coefficient Drot characterizing motility noise. A weak amount of motility noise slightly increases chemotactic performance.
更多查看译文
AI 理解论文
溯源树
样例
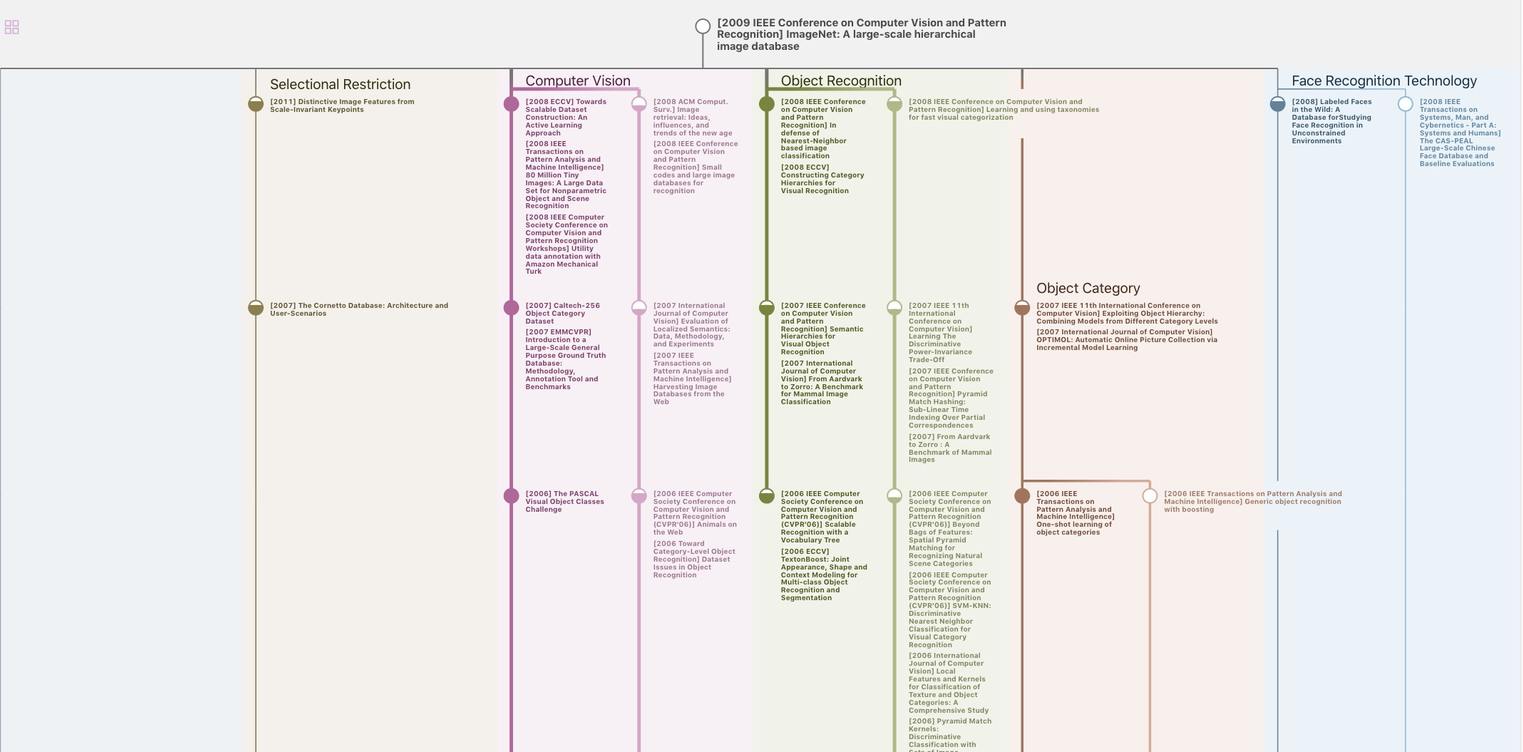
生成溯源树,研究论文发展脉络
Chat Paper
正在生成论文摘要