Leveraging vehicle connectivity and autonomy for highway bottleneck congestion mitigation using reinforcement learning
TRANSPORTMETRICA A-TRANSPORT SCIENCE(2023)
摘要
Automation and connectivity based platforms have great potential for managing highway traffic congestion including bottlenecks. Speed harmonisation (SH), one of such platforms, is an Active Traffic Management (ATM) strategy that addresses flow breakdown in real-time by adjusting upstream traffic speeds. However, SH has limitations including the need for supporting roadway infrastructure that is immovable and has limited coverage; the inability to enact control beyond its range; and the dependence on human driver compliance. These issues could be addressed by leveraging connected and automated vehicles (CAVs), which can collect information and execute control along their trajectories, irrespective of drivers’ awareness or compliance. In addressing this objective, this study utilises reinforcement learning to present a CAV control model to achieve efficient speed harmonisation. The results suggest that even at low market penetration, CAVs can significantly mitigate traffic congestion bottlenecks to a greater extent compared to traditional SH approaches.
更多查看译文
关键词
Speed harmonisation,congestion,bottleneck,deep reinforcement learning,connected and autonomous vehicles,active traffic management
AI 理解论文
溯源树
样例
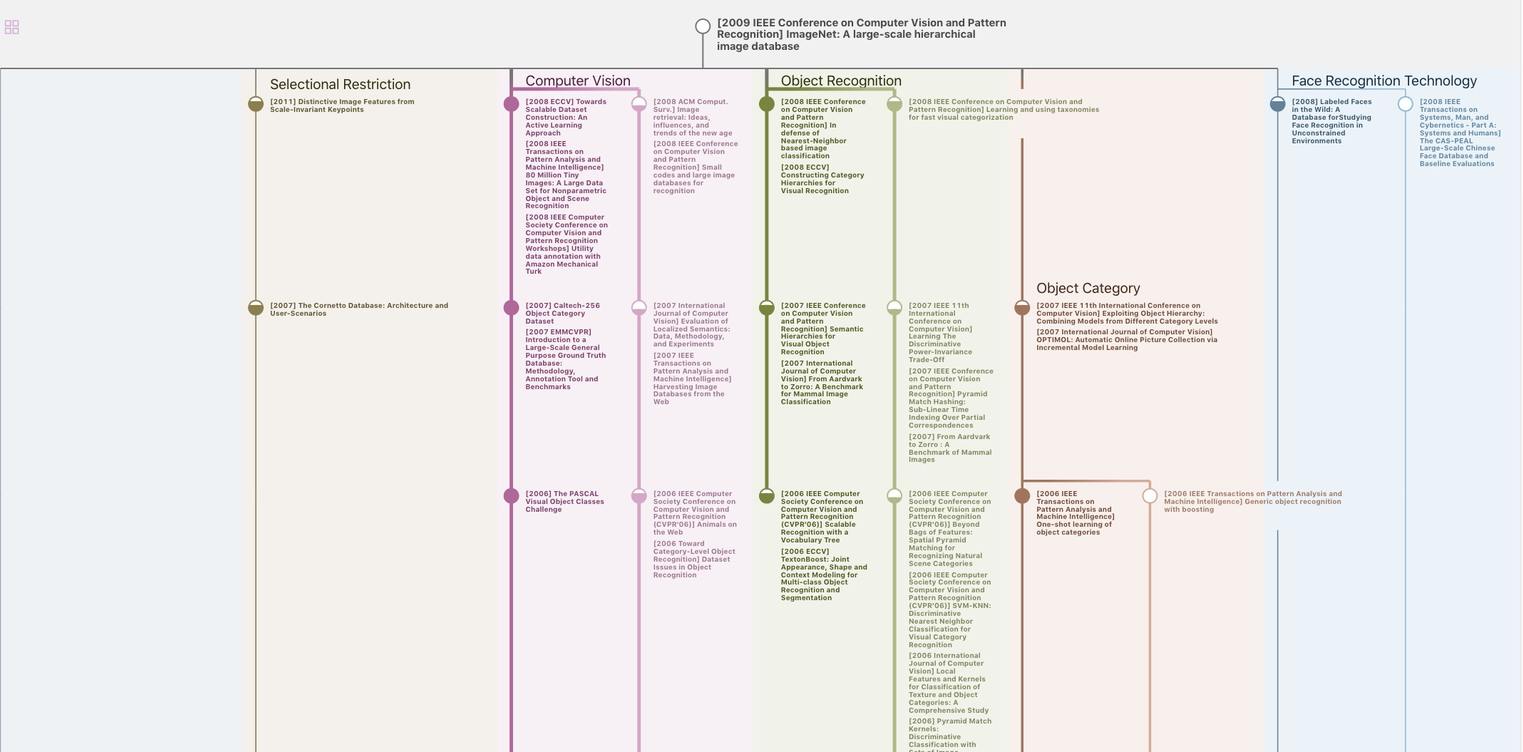
生成溯源树,研究论文发展脉络
Chat Paper
正在生成论文摘要