Neighboring relation enhanced inductive knowledge graph link prediction via meta-learning
WORLD WIDE WEB-INTERNET AND WEB INFORMATION SYSTEMS(2023)
摘要
Inductive link prediction over knowledge graphs(KGs) aims to perform inference over a new graph with unseen entities. In contrast to transductive link prediction, which learns a fixed set of known entities and cannot handle unseen entities, inductive link prediction is closer to real-world scenarios because of the evolving nature of KGs. The recently proposed methods utilize the enclosing subgraph of candidate triples to obtain inductive ability. However, these methods explicitly encode the local subgraph surrounding each node pair and neglect the entity-independent neighboring relation modeling, resulting in poor generalization to unseen entities and high time complexity. Inspired by the success of meta-learning on out-of-domain generalization, we propose a novel N eighb O ring relati O n enhance D meta- L earn E r(NOODLE) for inductive link prediction. NOODLE sufficiently exploits the neighboring relations from two aspects: commonsense semantic features and topological correlation information. Moreover, NOODLE rapidly learns transferable relations-specific information via meta-learning and generalizes well to unseen entities. Extensive experiments show that NOODLE outperforms the state-of-the-art methods on commonly used benchmark datasets in terms of effectiveness, generalization, and efficiency.
更多查看译文
关键词
Knowledge graph,Inductive link prediction,Neighboring relations,Meta-learning
AI 理解论文
溯源树
样例
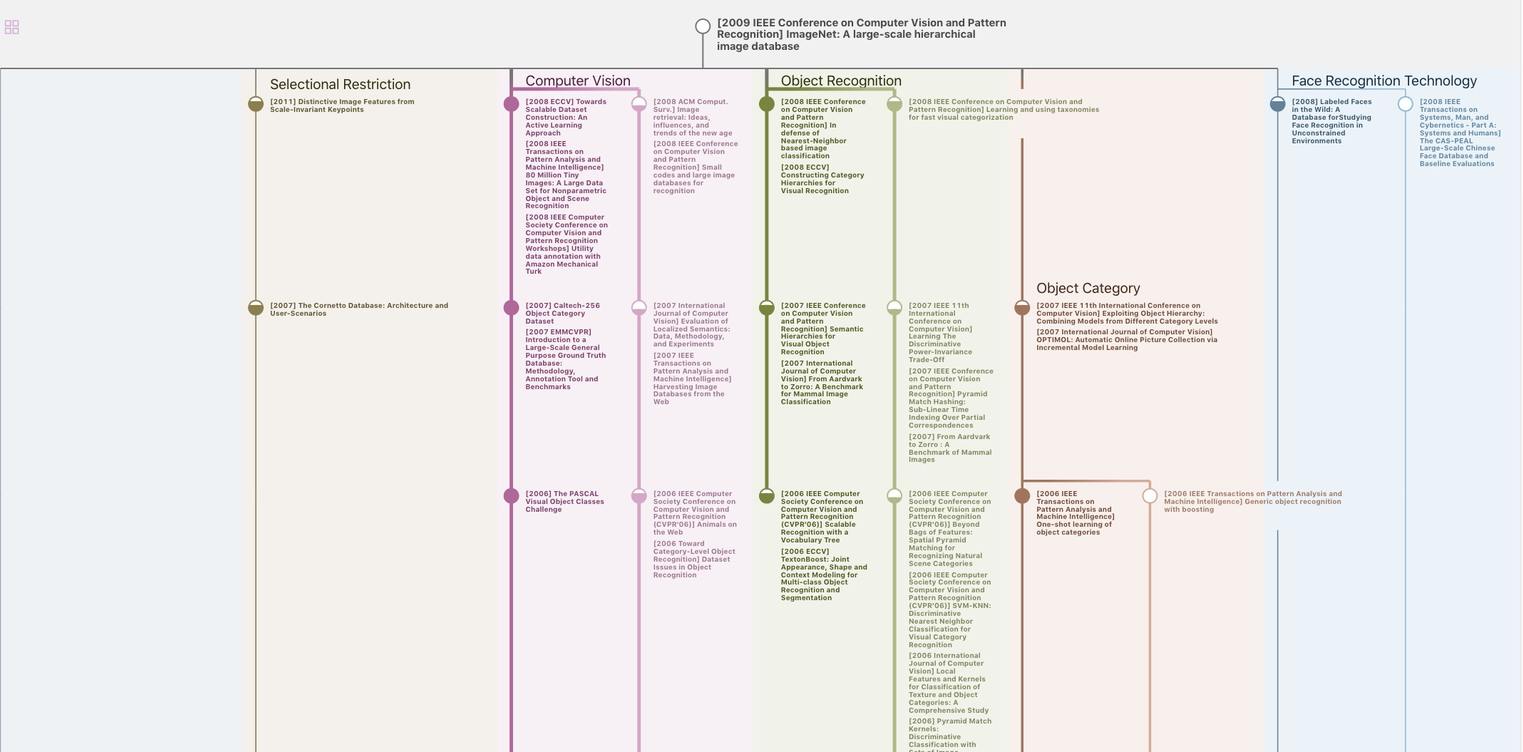
生成溯源树,研究论文发展脉络
Chat Paper
正在生成论文摘要