Reassignment Algorithm of the Ride-Sourcing Market Based on Reinforcement Learning
IEEE TRANSACTIONS ON INTELLIGENT TRANSPORTATION SYSTEMS(2023)
摘要
Reassignment strategies are of great significance to improve the dispatching efficiency of the ride-sourcing market by reassigning drivers and passengers. However, due to the focus on the feasibility of the reassignment strategy in the short period, previous studies ignore possible reassignment opportunities in the future and inevitably make short-sighted reassignment decisions. To fully exploit the effect of the reassignment strategy, this study proposes a two-stage reassignment framework, which integrates a reinforcement learning algorithm and the bilateral matching reassignment model. The Markov decision process is adopted to dynamically model the reassignment problem. In the framework, the reinforcement learning algorithm is utilized to first learn the randomness and dynamics of travel patterns from historical data and select vehicles participating in the reassignment process. Then, the bilateral matching reassignment model formulates the matching relationship after reassignment for passengers (drivers). Furthermore, for the cases where reassignment may increase individual matching distance, a personalized bilateral matching reassignment model is developed to avoid that. Experiments based on real data in Beijing found that learning passenger travel patterns and adjusting vehicle reassignment moments can greatly improve the passenger experience and reduce driving costs. The results also suggest that the efficiency of the reassignment strategy is influenced by the supply and demand conditions of the ride-sourcing system. This justifies that the framework can be applied to optimize the dispatching process, reduce carbon emissions, and build an eco-friendly travel system.
更多查看译文
关键词
Dispatching,Vehicles,Heuristic algorithms,Vehicle dynamics,Spatiotemporal phenomena,Reinforcement learning,Optimization,Ride-sourcing,reassignment,reinforcement learning,Markov decision process
AI 理解论文
溯源树
样例
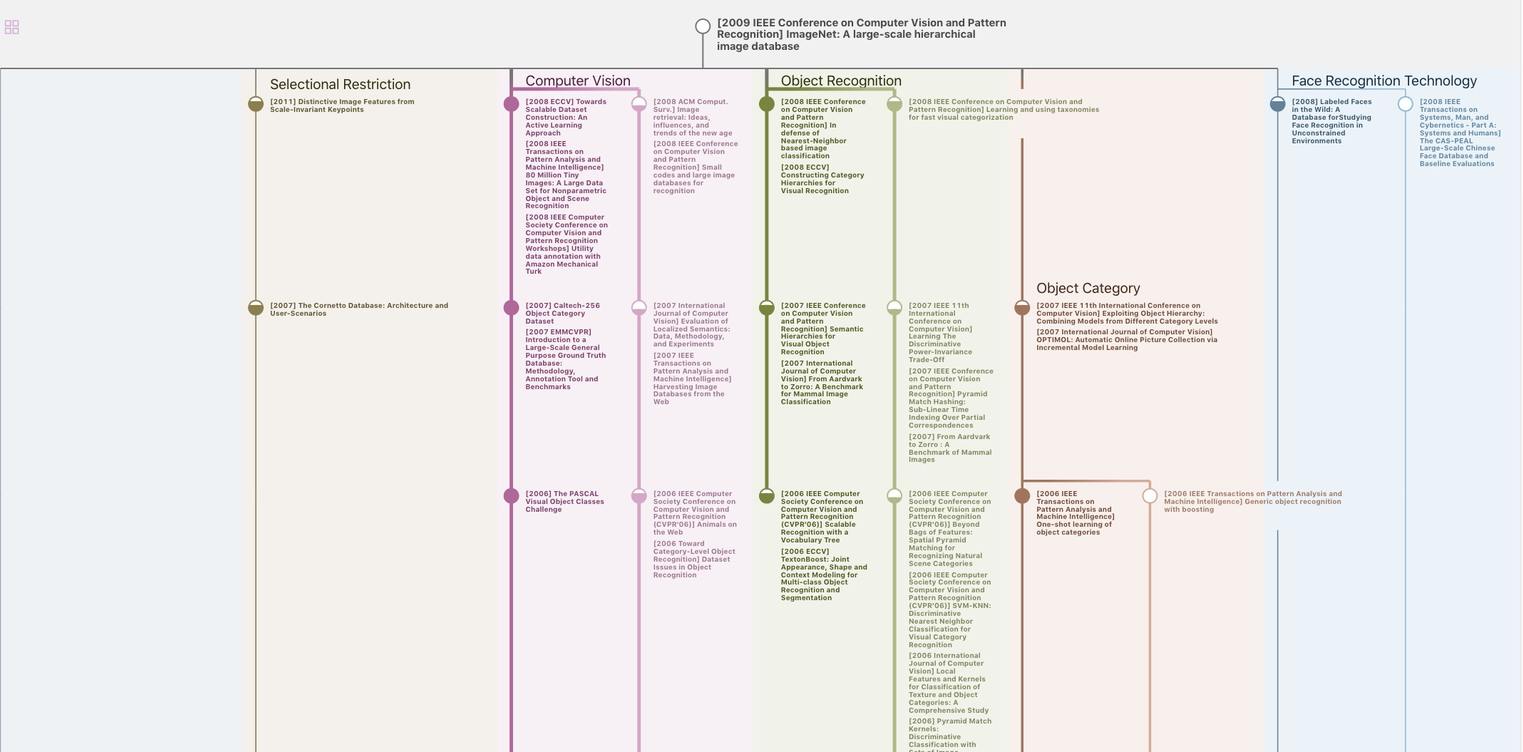
生成溯源树,研究论文发展脉络
Chat Paper
正在生成论文摘要