Predicting agricultural drought using meteorological and ENSO parameters in different regions of Iran based on the LSTM model
STOCHASTIC ENVIRONMENTAL RESEARCH AND RISK ASSESSMENT(2023)
摘要
Agricultural drought refers to soil moisture deficit. It can directly impact crops and adversely affect various economic sectors. Hence, its accurate and precise prediction can help develop plans for agricultural drought and reduce crop damage. This research used a long short-term memory (LSTM) model for estimating drought over Iran during spring and summer. The predictions were made based on the large-scale indicator named El Nino-Southern Oscillation (ENSO), normalized difference vegetation index (NDVI), and meteorological parameters (i.e., precipitation and temperature). For this purpose, the agreement between NDVI, meteorological parameters, and ENSO was studied at time lags of 1–4 months to understand the agricultural drought predictors. The calculated RMSE and NSE of the LSTM model revealed that the model showed a better performance in predicting NDVI values in semi-arid and arid areas such that the RMSE and NSE of the LSTM model are less than 0.3 and higher than 0.8, respectively. Furthermore, the prediction performance in humid and semi-humid areas was better in spring than in summer. The average RMSE across Iran was 0.4 in the spring, whereas the RMSE increased to 0.78 in the summer. In addition, precipitation deficit exhibited its effects on agricultural drought at a time lag of 1 month, whereas ENSO caused agricultural drought at longer time lags. Moreover, In April, agricultural droughts in the north, northwest, and southwest of Iran occurred under the ENSO effects. In northern regions (Caspian Sea coasts), the correlation between agricultural drought and ENSO is close to 0.1. The correlation equals 0.8 in the west and northwest of Iran. ENSO had the greatest impact on NDVI in these regions in April, with a 1 month delay. These findings can be exploited for issuing agricultural drought warnings in Iran.
更多查看译文
关键词
Prediction,Agriculture drought,ENSO,LSTM
AI 理解论文
溯源树
样例
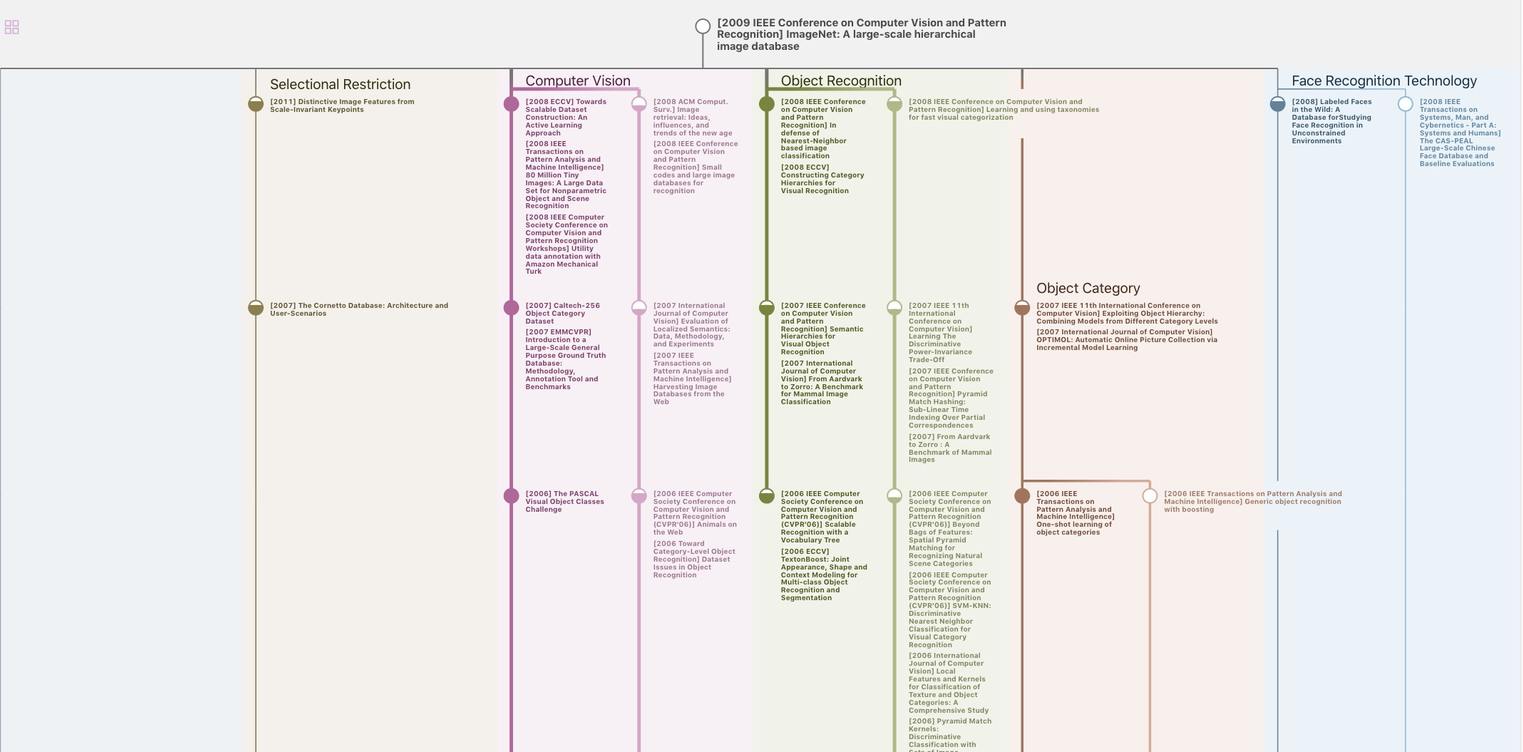
生成溯源树,研究论文发展脉络
Chat Paper
正在生成论文摘要