Detecting subpixel human settlements in mountains using deep learning: A case of the Hindu Kush Himalaya 1990-2020
REMOTE SENSING OF ENVIRONMENT(2023)
Abstract
The majority of future population growth in mountains will occur in small- and medium-sized cities and towns and affect vulnerable ecosystems. However, mountain settlements are often omitted from global land cover analyses due to the low spatial resolution of satellite images, which cannot resolve the small scale of mountains settlements. This study demonstrates, for the first time, the potential of deep learning to detect human settlements in mountains at the sub-pixel level, based on Landsat satellite imagery. We hypothesized that adding spatial and temporal features could improve the detection of mountain settlements since spectral information alone led to inaccurate results. For spatial features, we compared a U-shaped neural network (U-Net), a deep learning algorithm that automatically learns spatial features, with a simple random forest (RF) algorithm. Then, we assessed whether temporal features would increase accuracy by comparing two input datasets, multispectral imagery and temporal features from the Continuous Change Detection and Classification (CCDC) algorithm. We evaluated each method by calculating the accuracies of (1) the binary settlement footprint, (2) the subpixel estimates of impervious surfaces, and (3) urban growth. We tested the accuracies using visually interpreted datasets from time-series Google Earth images across the Hindu Kush Himalaya that were not used for training to evaluate model transferability. The U-Net successfully improved mountain settlement mapping compared to the random forest, with a substantial discrepancy in small settlements. The time-series results from the U-Net successfully captured long-term urban growth but fewer short-term changes. Contrary to expectations, the CCDC temporal features reduced the accuracy of mountain settlement mapping due to frequent cloud cover in hilly areas. Our subpixel analysis reveals that the built-up area of the Hindu Kush Himalaya has expanded at a rate of 61 km2 per year from 1990 to 2020, which is about twice the estimate of the Global Human Settlement Layer using binary urban/non-urban classifications.
MoreTranslated text
Key words
Urban land cover,Land cover fraction,Peri-urban,Built-up area,Subpixel mapping,Machine learning,Time -series,Himalaya,CCDC
AI Read Science
Must-Reading Tree
Example
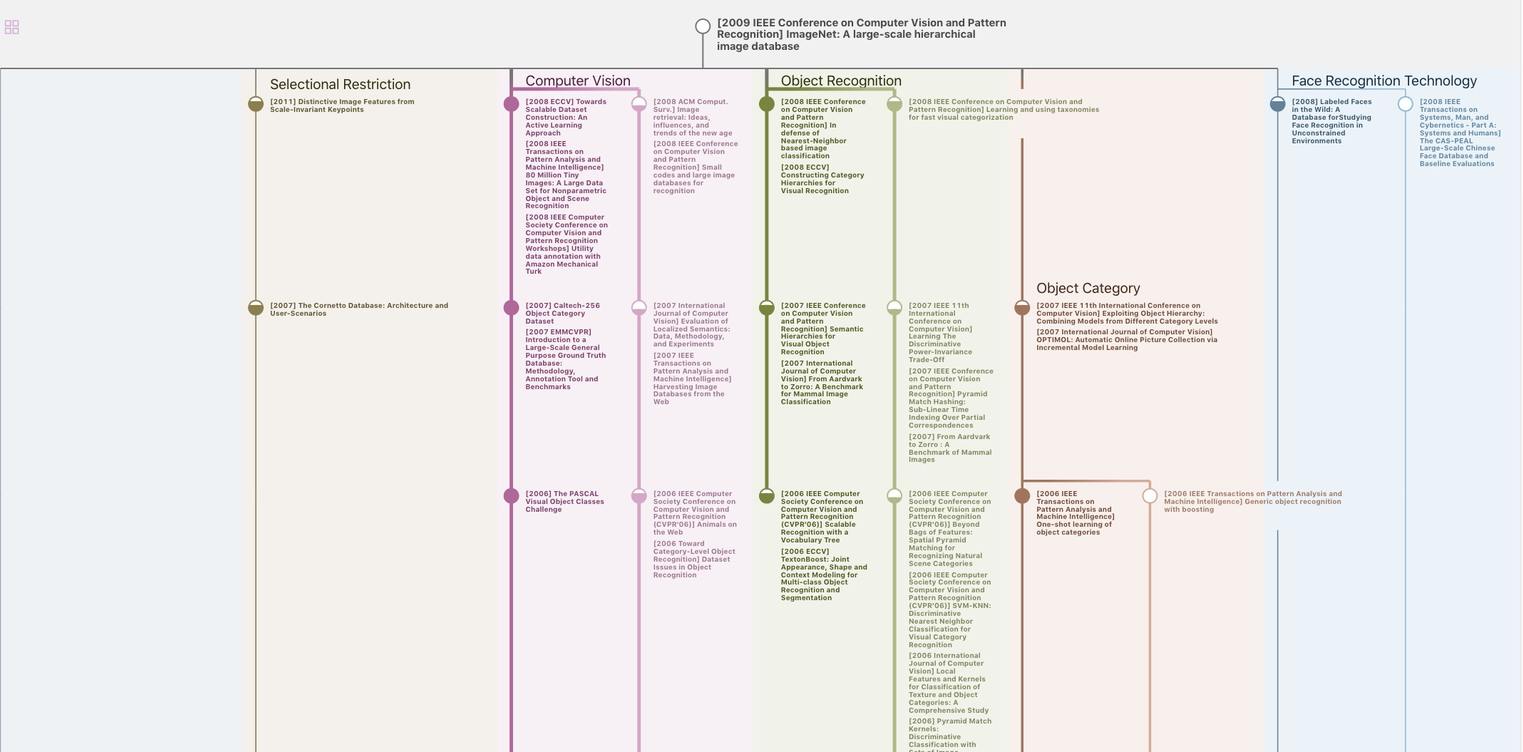
Generate MRT to find the research sequence of this paper
Chat Paper
Summary is being generated by the instructions you defined