CFormerFaceNet: Efficient Lightweight Network Merging a CNN and Transformer for Face Recognition
APPLIED SCIENCES-BASEL(2023)
Abstract
Most face recognition methods rely on deep convolutional neural networks (CNNs) that construct multiple layers of processing units in a cascaded form and employ convolution operations to fuse local features. However, these methods are not conducive to modeling the global semantic information of the face and lack attention to important facial feature regions and their spatial relationships. In this work, a Group Depth-Wise Transpose Attention (GDTA) block is designed to effectively capture both local and global representations, mitigate the issue of limited receptive fields in CNNs, and establish long-range dependencies among different feature regions. Based on GDTA and CNNs, a novel, efficient, and lightweight face recognition model called CFormerFaceNet, which combines a CNN and Transformer, is proposed. The model significantly reduces the parameters and computational cost without compromising performance, greatly improving the computational efficiency of deep neural networks in face recognition tasks. The model achieves competitive accuracy on multiple challenging benchmark face datasets, including LFW, CPLFW, CALFW, SLLFW, CFP_FF, CFP_FP, and AgeDB-30, while maintaining the minimum computational cost compared to all other advanced face recognition models. The experimental results using computers and embedded devices also demonstrate that it can meet real-time requirements in practical applications.
MoreTranslated text
Key words
face recognition,CNN,transformer,lightweight network,transpose attention
AI Read Science
Must-Reading Tree
Example
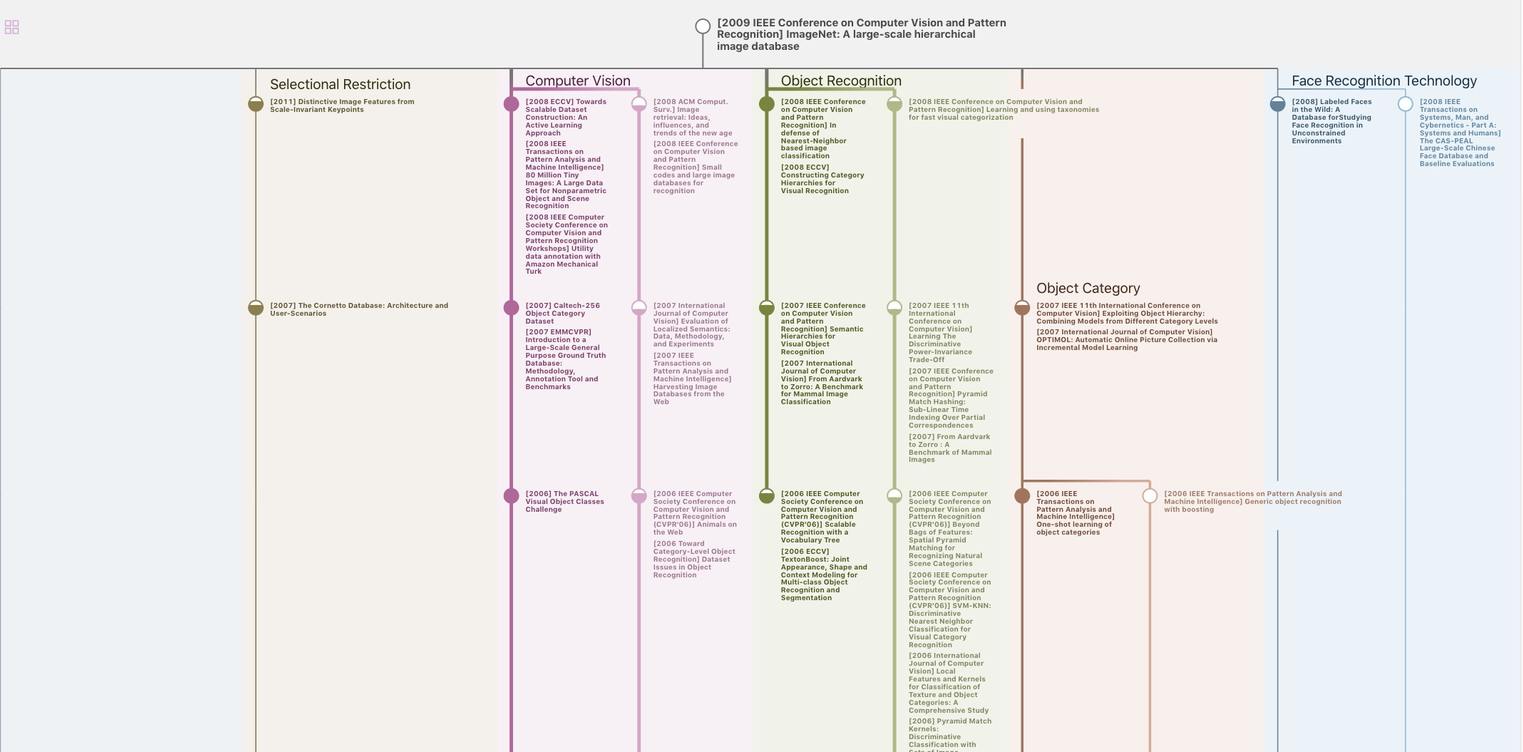
Generate MRT to find the research sequence of this paper
Chat Paper
Summary is being generated by the instructions you defined