Machine Learning Algorithms for Identifying Dependencies in OT Protocols
ENERGIES(2023)
摘要
This study illustrates the utility and effectiveness of machine learning algorithms in identifying dependencies in data transmitted in industrial networks. The analysis was performed for two different algorithms. The study was carried out for the XGBoost (Extreme Gradient Boosting) algorithm based on a set of decision tree model classifiers, and the second algorithm tested was the EBM (Explainable Boosting Machines), which belongs to the class of Generalized Additive Models (GAM). Tests were conducted for several test scenarios. Simulated data from static equations were used, as were data from a simulator described by dynamic differential equations, and the final one used data from an actual physical laboratory bench connected via Modbus TCP/IP. Experimental results of both techniques are presented, thus demonstrating the effectiveness of the algorithms. The results show the strength of the algorithms studied, especially against static data. For dynamic data, the results are worse, but still at a level that allows using the researched methods to identify dependencies. The algorithms presented in this paper were used as a passive protection layer of a commercial IDS (Intrusion Detection System).
更多查看译文
关键词
cybersecurity,machine learning,XGBoost,EBM,GAM,Modbus TCP/IP
AI 理解论文
溯源树
样例
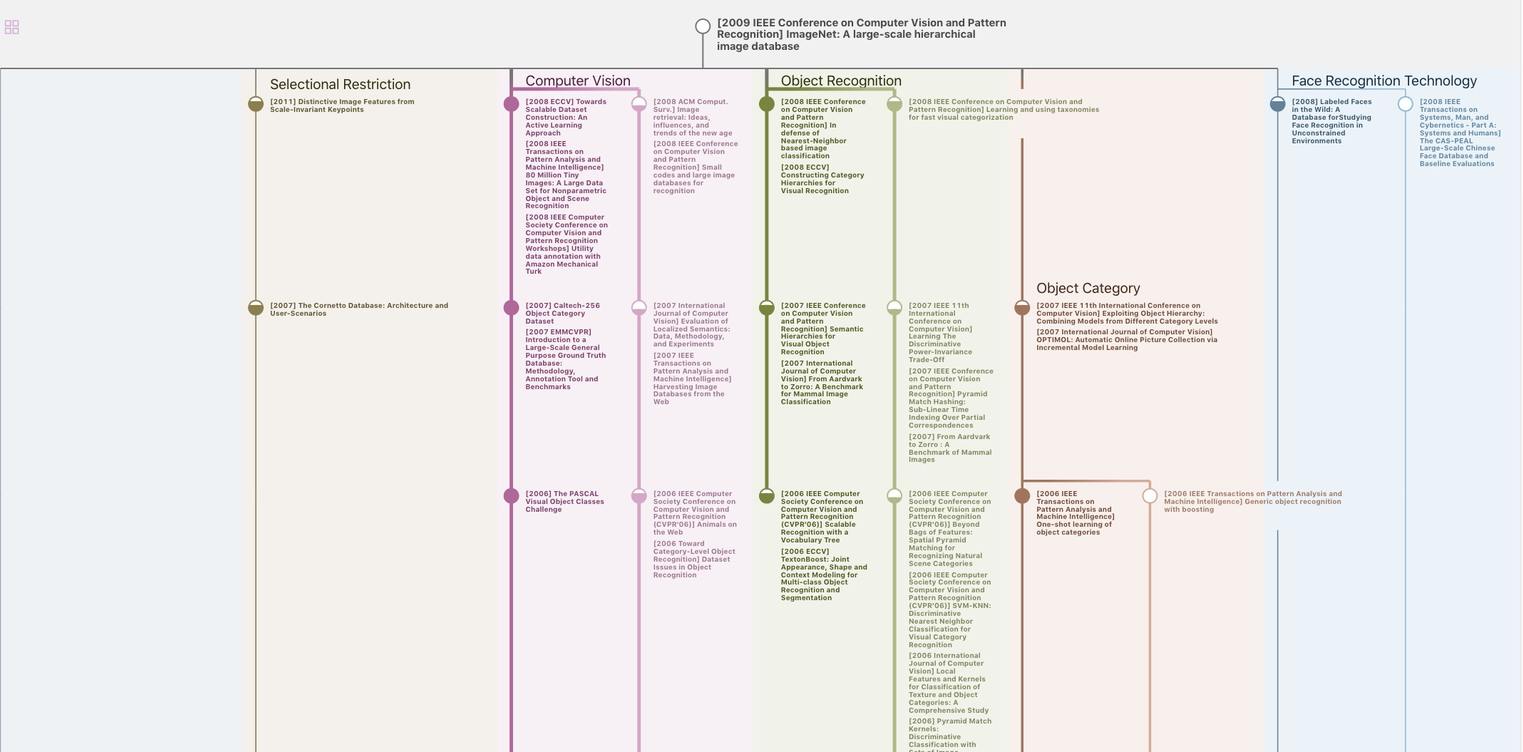
生成溯源树,研究论文发展脉络
Chat Paper
正在生成论文摘要