UnIC-Net: Uncertainty Aware Involution-Convolution Hybrid Network for Two-level Disease Identification
SOUTHEASTCON 2023(2023)
摘要
Convolution is commonly used in deep learning models for image classification problems, and its primary purpose is to retrieve representations in the spatial domain that are concealed from view. However, since convolution only works on a single channel at a time, it often ignores the cross-channel correlations that may exist in an image. Involution is an inversed process of convolutions, resolving the issues convolutions create. Involution is a location-specific process that are skillfully coupled with the well-established design of convolutions, which results in an excellent efficiency for the network, especially in cell-like images. But ensuring and analyzing robust and reliable performance in medical images are also very important, since uncertainty aware models are helpful when it comes to reducing the risk factors. We utilize Monte Carlo dropout (MCD), for introducing uncertainty-aware feature to our model. In this paper, we propose an uncertainty aware involution-convolution hybrid network that achieves 98.79% accuracy in HAM10000 dataset and 96.81% accuracy in Malaria parasitized cells dataset.
更多查看译文
关键词
involution,convolution,skin cancer,malaria parasite,image classification
AI 理解论文
溯源树
样例
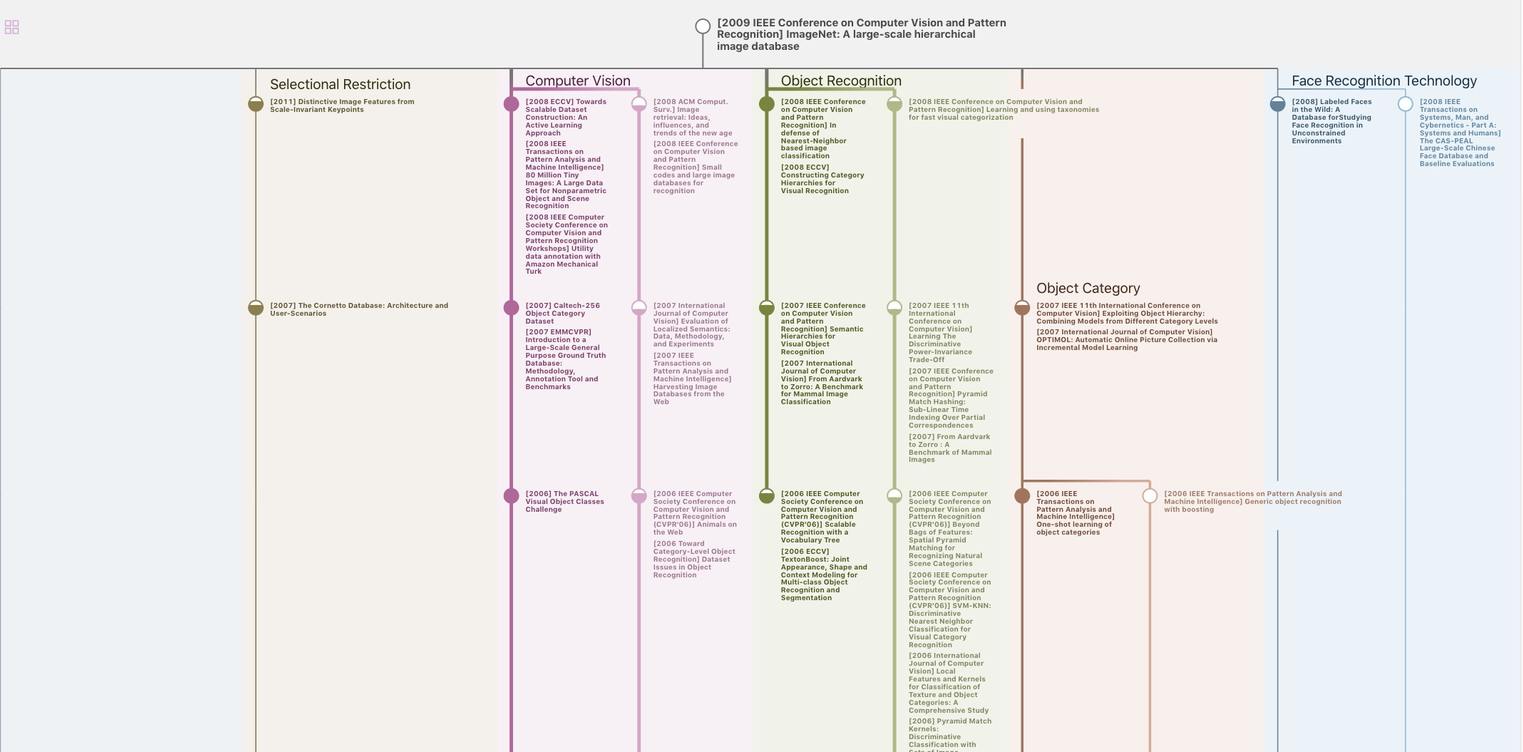
生成溯源树,研究论文发展脉络
Chat Paper
正在生成论文摘要