XGL-T transformer model for intelligent image captioning
MULTIMEDIA TOOLS AND APPLICATIONS(2024)
摘要
Image captioning extracts multiple semantic features from an image and integrates them into a sentence-level description. For efficient description of the captions, it becomes necessary to learn higher order interactions between detected objects and the relationship among them. Most of the existing systems take into account the first order interactions while ignoring the higher order ones. It is challenging to extract discriminant higher order semantics visual features in images with highly populated objects for caption generation. In this paper, an efficient higher order interaction learning framework is proposed using encoder-decoder based image captioning. A scaled version of Gaussian Error Linear Unit (GELU) activation function, x-GELU is introduced that controls the vanishing gradients and enhances the feature learning. To leverage higher order interactions among multiple objects, an efficient XGL Transformer (XGL-T) model is introduced that exploits both spatial and channel-wise attention by integrating four XGL attention modules in image encoder and one in Bilinear Long Short-Term Memory guided sentence decoder. The proposed model captures rich semantic concepts from objects, attributes, and their relationships. Extensive experiments are conducted on publicly available MSCOCO Karapathy test split and the best performance of the work is observed as 81.5 BLEU@1, 67.1 BLEU@2, 51.6 BLEU@3, 39.9 BLEU@4, 134 CIDEr, 59.9 ROUGE-L, 29.8 METEOR, 23.8 SPICE using CIDEr-D Score Optimization Strategy. The scores validate the significant improvements over state-of-the-art results. An ablation study is also carried out to support the experimental observations.
更多查看译文
关键词
Activation Function,Attention,Computer Vision,Higher Order Interaction,Image Captioning,XGL,Transformer
AI 理解论文
溯源树
样例
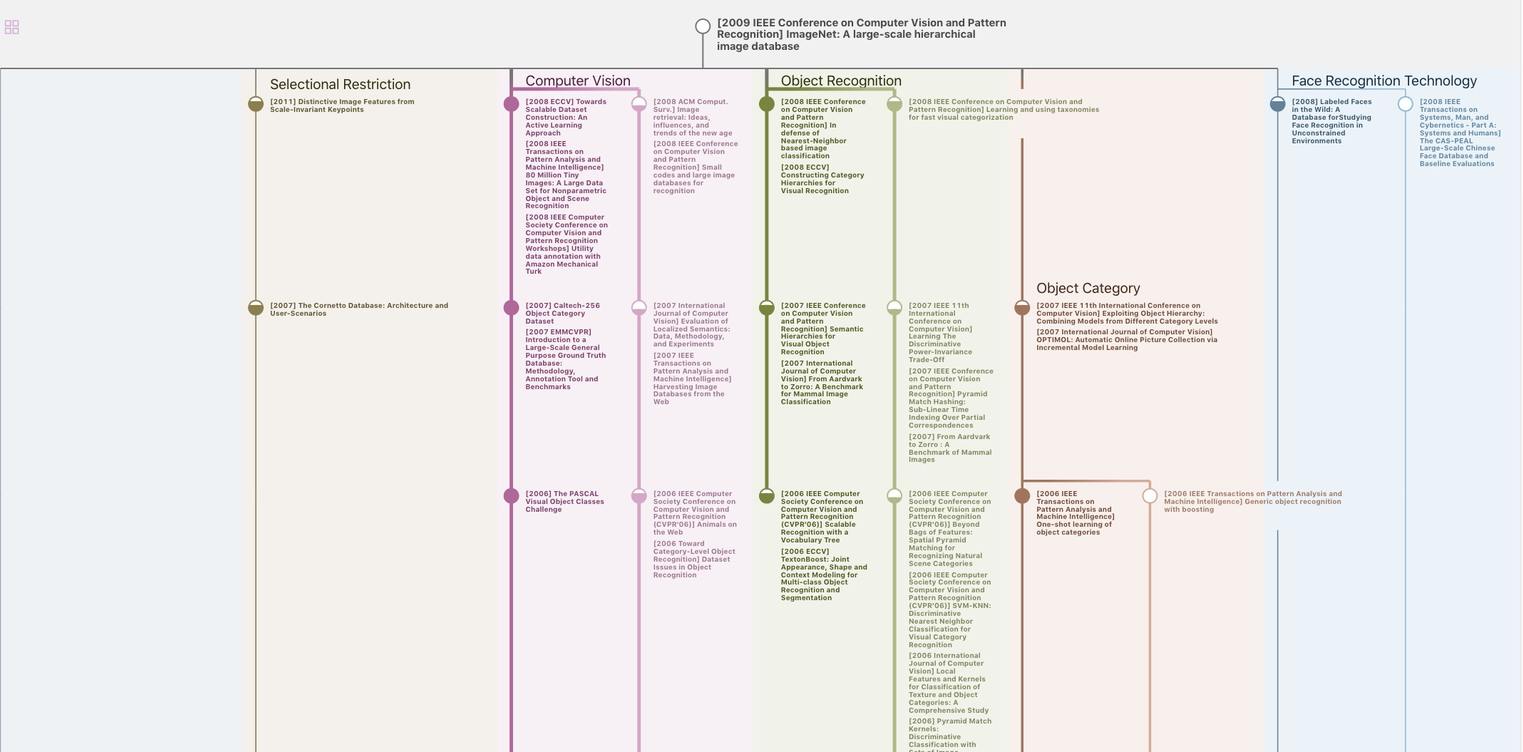
生成溯源树,研究论文发展脉络
Chat Paper
正在生成论文摘要