A dynamic clustering ensemble learning approach for crude oil price forecasting
ENGINEERING APPLICATIONS OF ARTIFICIAL INTELLIGENCE(2023)
Abstract
Accurate oil price forecasts matter, yet the nonstationarity of oil prices makes forecasting a challenging task. In this study, we propose a dynamic ensemble forecasting method for nonstationary oil prices using clustering approaches. Specifically, clustering is embedded in the ensemble forecasting framework, whereby the given period of historical observations is automatically classified into several clusters according to the data characteristics. This classification provides a solid groundwork for dynamically evaluating individual forecasting models in a targeted manner. We then propose a clustering-based regular increasing monotone weight assignment strategy that removes the influence of outliers and assigns appropriate weights to each forecasting model, thereby balancing the competitiveness and robustness of the proposed ensemble model. We verify the competitiveness and robustness of the proposed model by using West TX Intermediate oil prices. Results show that the proposed model significantly outperforms benchmarks and state-of-the-art methods in terms of horizontal and directional accuracy and is thus competitive. The robustness of the proposed model is validated using scenarios involving parameter variation and data missing assumptions. In summary, we present a model with promising effectiveness in promoting prediction performance in forecasting oil prices.
MoreTranslated text
Key words
Dynamic ensemble, Ensemble forecast, Oil price prediction, Clustering strategies
AI Read Science
Must-Reading Tree
Example
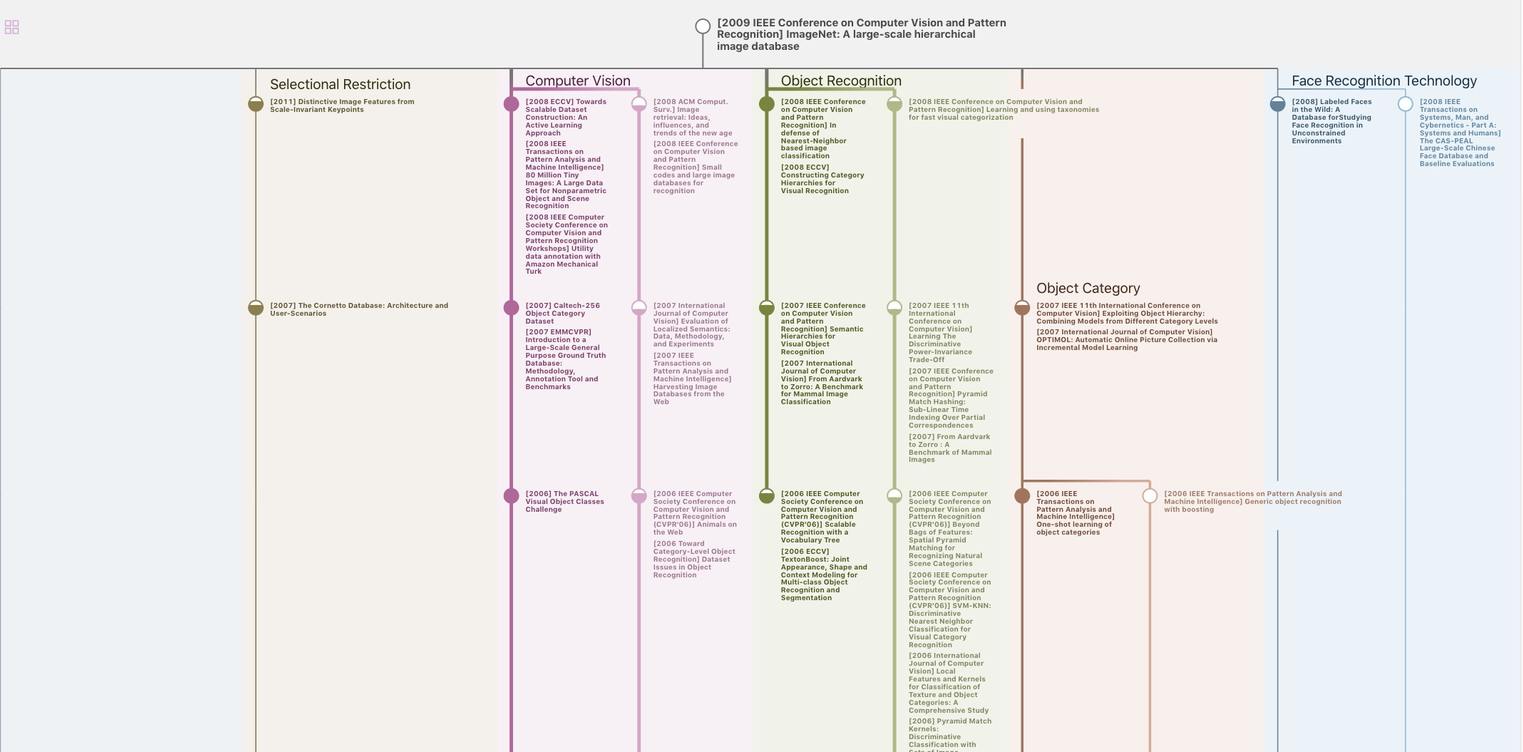
Generate MRT to find the research sequence of this paper
Chat Paper
Summary is being generated by the instructions you defined