RDJCNN: A micro-convolutional neural network for radar active jamming signal classification
Engineering Applications of Artificial Intelligence(2023)
摘要
Timely awareness of jamming situations and classification of jamming categories are vital for radars to suppress jamming, ensure viability, and maintain functions in complex electromagnetic environments. To satisfy strict time requirements for radars on embedded devices, a micro-dynamic convolutional neural network for jamming signal classification is proposed in this paper. The proposed network takes the range-Doppler distribution obtained from built-in radar signal processing as input. The proposed data augmentation algorithm, together with the attention mechanism and the efficient convolutional architecture, improves the generalization capability and reduces the computational complexity. In addition, we propose a dynamic depth mechanism based on a task difficulty evaluator that enables the network to be adjusted automatically and further reduces the average computational complexity of classification. Simulation results verify the advantages of our approach in size, accuracy, and efficiency. The proposed network achieved 98.82% and 85.00% top-1 accuracy in two datasets with only 1.73 M multiply–accumulate operations.
更多查看译文
关键词
Convolutional neural networks,Radar signal-processing,Jamming situation awareness,Jamming signal classification
AI 理解论文
溯源树
样例
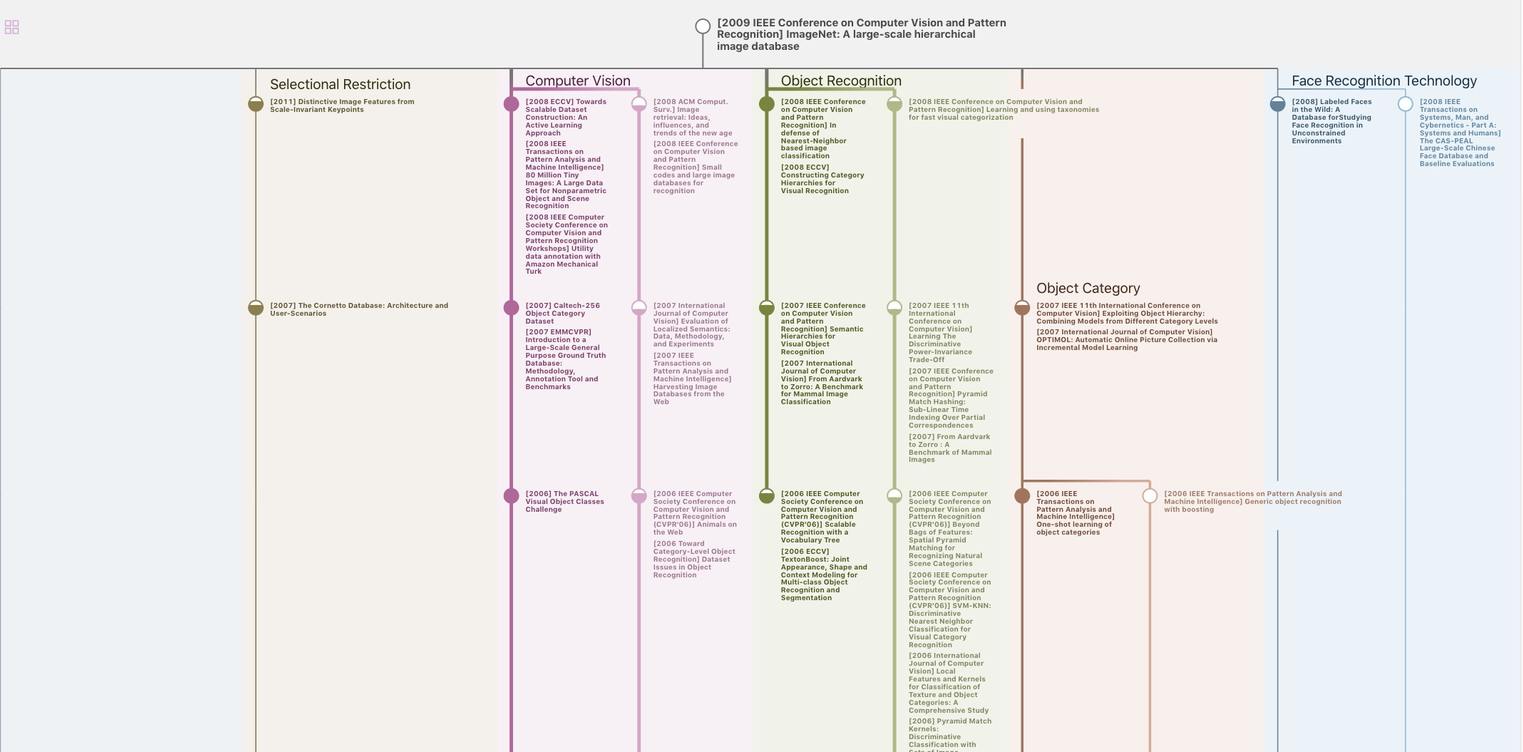
生成溯源树,研究论文发展脉络
Chat Paper
正在生成论文摘要