Comparison of pulmonary congestion severity using artificial intelligence-assisted scoring versus clinical experts: a secondary analysis of BLUSHED-AHF
EUROPEAN JOURNAL OF HEART FAILURE(2023)
摘要
AimAcute decompensated heart failure (ADHF) is the leading cause of cardiovascular hospitalizations in the United States. Detecting B-lines through lung ultrasound (LUS) can enhance clinicians' prognostic and diagnostic capabilities. Artificial intelligence/machine learning (AI/ML)-based automated guidance systems may allow novice users to apply LUS to clinical care. We investigated whether an AI/ML automated LUS congestion score correlates with expert's interpretations of B-line quantification from an external patient dataset. Methods and resultsThis was a secondary analysis from the BLUSHED-AHF study which investigated the effect of LUS-guided therapy on patients with ADHF. In BLUSHED-AHF, LUS was performed and B-lines were quantified by ultrasound operators. Two experts then separately quantified the number of B-lines per ultrasound video clip recorded. Here, an AI/ML-based lung congestion score (LCS) was calculated for all LUS clips from BLUSHED-AHF. Spearman correlation was computed between LCS and counts from each of the original three raters. A total of 3858 LUS clips were analysed on 130 patients. The LCS demonstrated good agreement with the two experts' B-line quantification score (r = 0.894, 0.882). Both experts' B-line quantification scores had significantly better agreement with the LCS than they did with the ultrasound operator's score (p < 0.005, p < 0.001). ConclusionArtificial intelligence/machine learning-based LCS correlated with expert-level B-line quantification. Future studies are needed to determine whether automated tools may assist novice users in LUS interpretation.
更多查看译文
关键词
pulmonary congestion severity,clinical experts,artificial intelligence‐assisted
AI 理解论文
溯源树
样例
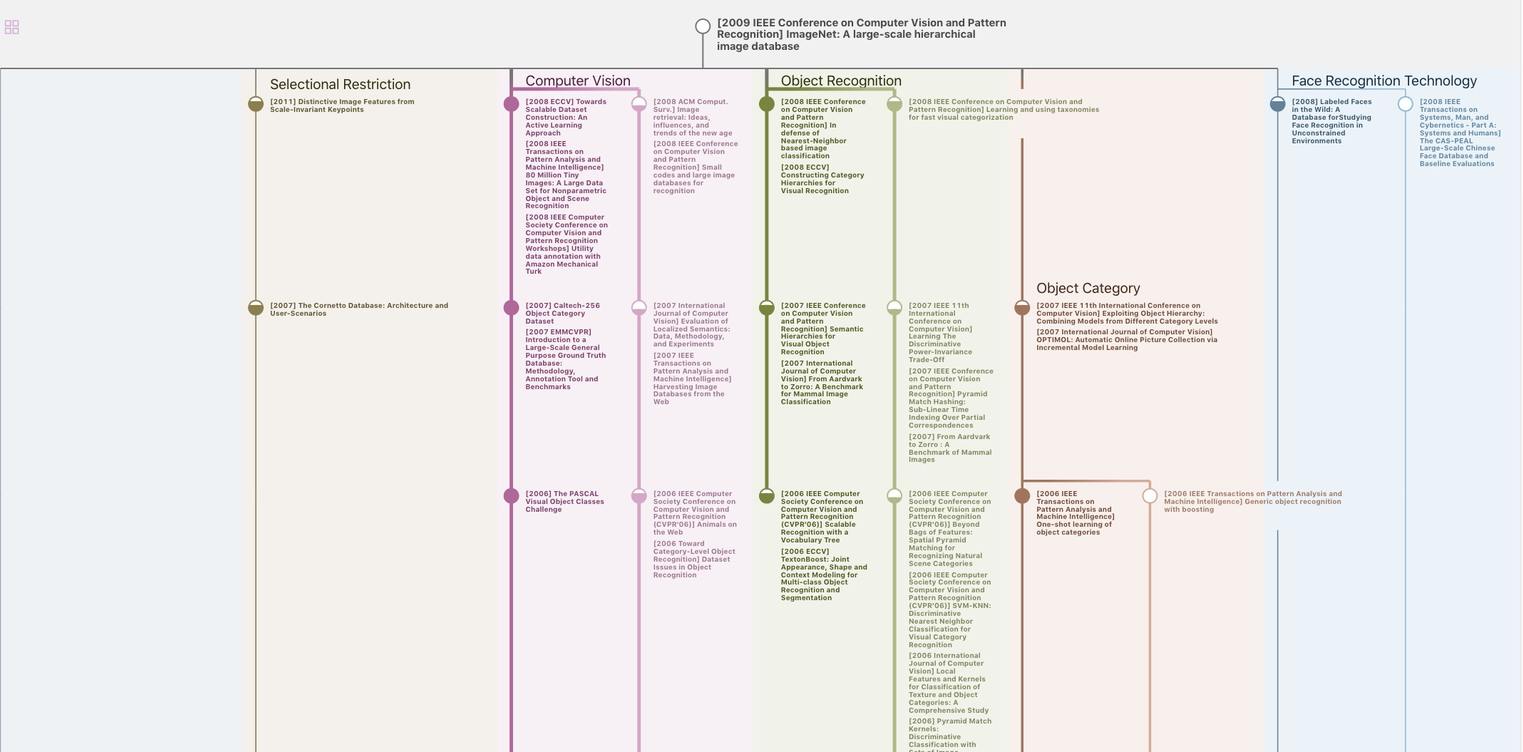
生成溯源树,研究论文发展脉络
Chat Paper
正在生成论文摘要