ESSR-GAN: Enhanced super and semi supervised remora resolution based generative adversarial learning framework model for smartphone based road damage detection
MULTIMEDIA TOOLS AND APPLICATIONS(2024)
摘要
Road surface condition detection is a significant application for many intelligent transportation systems (ITSs) to uphold favourable driving conditions and avoid accidents. However, the accurate detection and classification of road damages have become more challenging. Thus, this paper proposed an enhanced sensor based technology that determines road damage by employing a deep learning (DL) algorithm. Initially, the road damage image is acquired from mobilephone devices and pre-processed using the adaptive Gaussian bilateral filter (AGBF). Then, after data augmentation, the proposed method has applied the super and semi-supervised remora adversarial resolution learning generative (S3-RARLG) model for road damage detection and classification. Initially, a Super-Generative Adversarial Network (SGAN) is used in S3-RARLG to maximize road image clarity and improve road damage detection performance. Then semi-supervised learning is adapted to address the insufficiency of label images by maximizing the expandability of training data. Finally, adversarial learning is enhanced by integrating with SGAN to improve detection performance. Moreover, in S3-RARLG, the weight updation is executed using bionic remora meta-heuristic optimization (BRMO). The proposed ESSR-GAN is simulated on the Python platform, and the performance metrics are determined to demonstrate the effectiveness of the proposed model using the road damage dataset 2019. As a result, the proposed ESSR-GAN accomplishes a higher accuracy of 99.12%, and the acquired results outperform the existing architectures.
更多查看译文
关键词
Super-generative adversarial network,Adaptive Gaussian bilateral filter,Bionic remora meta-heuristic optimization,Semi-supervised learning,Road damage detection
AI 理解论文
溯源树
样例
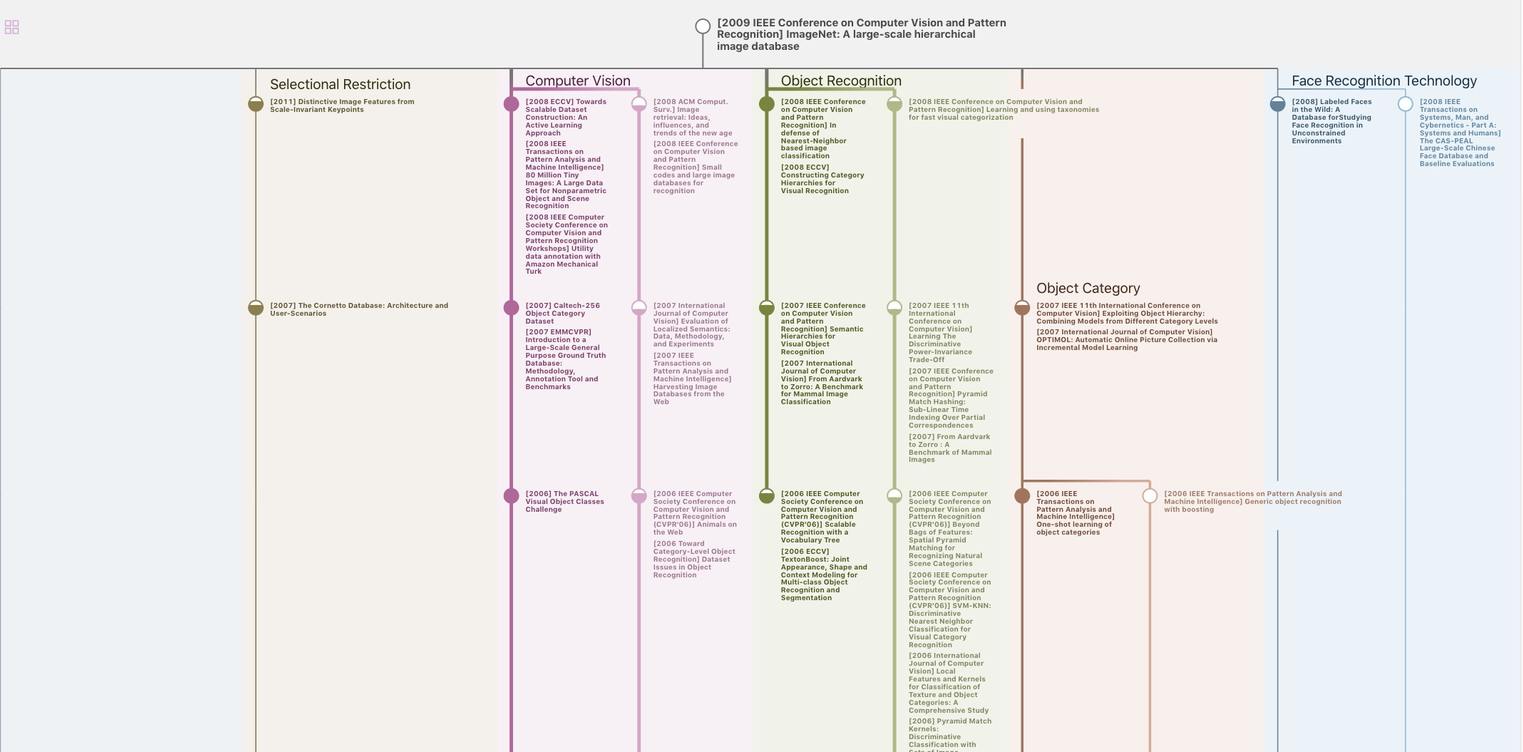
生成溯源树,研究论文发展脉络
Chat Paper
正在生成论文摘要