Multicomponent Adversarial Domain Adaptation: A General Framework.
IEEE TRANSACTIONS ON NEURAL NETWORKS AND LEARNING SYSTEMS(2023)
摘要
Domain adaptation (DA) aims to transfer knowledge from one source domain to another different but related target domain. The mainstream approach embeds adversarial learning into deep neural networks (DNNs) to either learn domain-invariant features to reduce the domain discrepancy or generate data to fill in the domain gap. However, these adversarial DA (ADA) approaches mainly consider the domain-level data distributions, while ignoring the differences among components contained in different domains. Therefore, components that are not related to the target domain are not filtered out. This can cause a negative transfer. In addition, it is difficult to make full use of the relevant components between the source and target domains to enhance DA. To address these limitations, we propose a general two-stage framework, named multicomponent ADA (MCADA). This framework trains the target model by first learning a domain-level model and then fine-tuning that model at the component-level. In particular, MCADA constructs a bipartite graph to find the most relevant component in the source domain for each component in the target domain. Since the nonrelevant components are filtered out for each target component, fine-tuning the domain-level model can enhance positive transfer. Extensive experiments on several real-world datasets demonstrate that MCADA has significant advantages over state-of-the-art methods.
更多查看译文
关键词
Adversarial training,bipartite graph,domain adaptation (DA),multicomponent
AI 理解论文
溯源树
样例
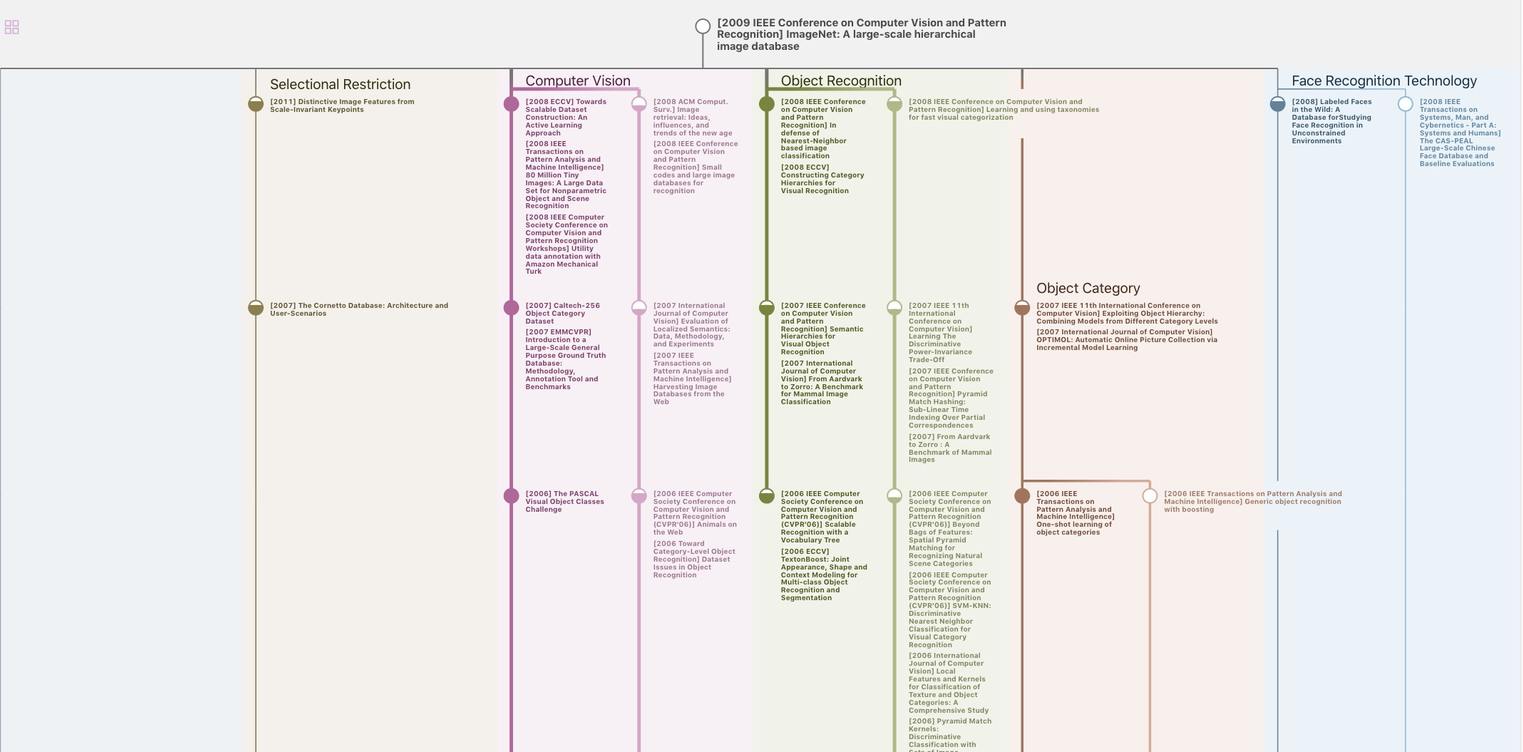
生成溯源树,研究论文发展脉络
Chat Paper
正在生成论文摘要