Dissimilarity-Preserving Representation Learning for One-Class Time Series Classification.
IEEE transactions on neural networks and learning systems(2023)
摘要
We propose to embed time series in a latent space where pairwise Euclidean distances (EDs) between samples are equal to pairwise dissimilarities in the original space, for a given dissimilarity measure. To this end, we use auto-encoder (AE) and encoder-only neural networks to learn elastic dissimilarity measures, e.g., dynamic time warping (DTW), that are central to time series classification (Bagnall et al., 2017). The learned representations are used in the context of one-class classification (Mauceri et al., 2020) on the datasets of UCR/UEA archive (Dau et al., 2019). Using a 1-nearest neighbor (1NN) classifier, we show that learned representations allow classification performance that is close to that of raw data, but in a space of substantially lower dimensionality. This implies substantial and compelling savings in terms of computational and storage requirements for nearest neighbor time series classification.
更多查看译文
关键词
Autoencoders (AEs),one-class classification,representation learning,time series classification
AI 理解论文
溯源树
样例
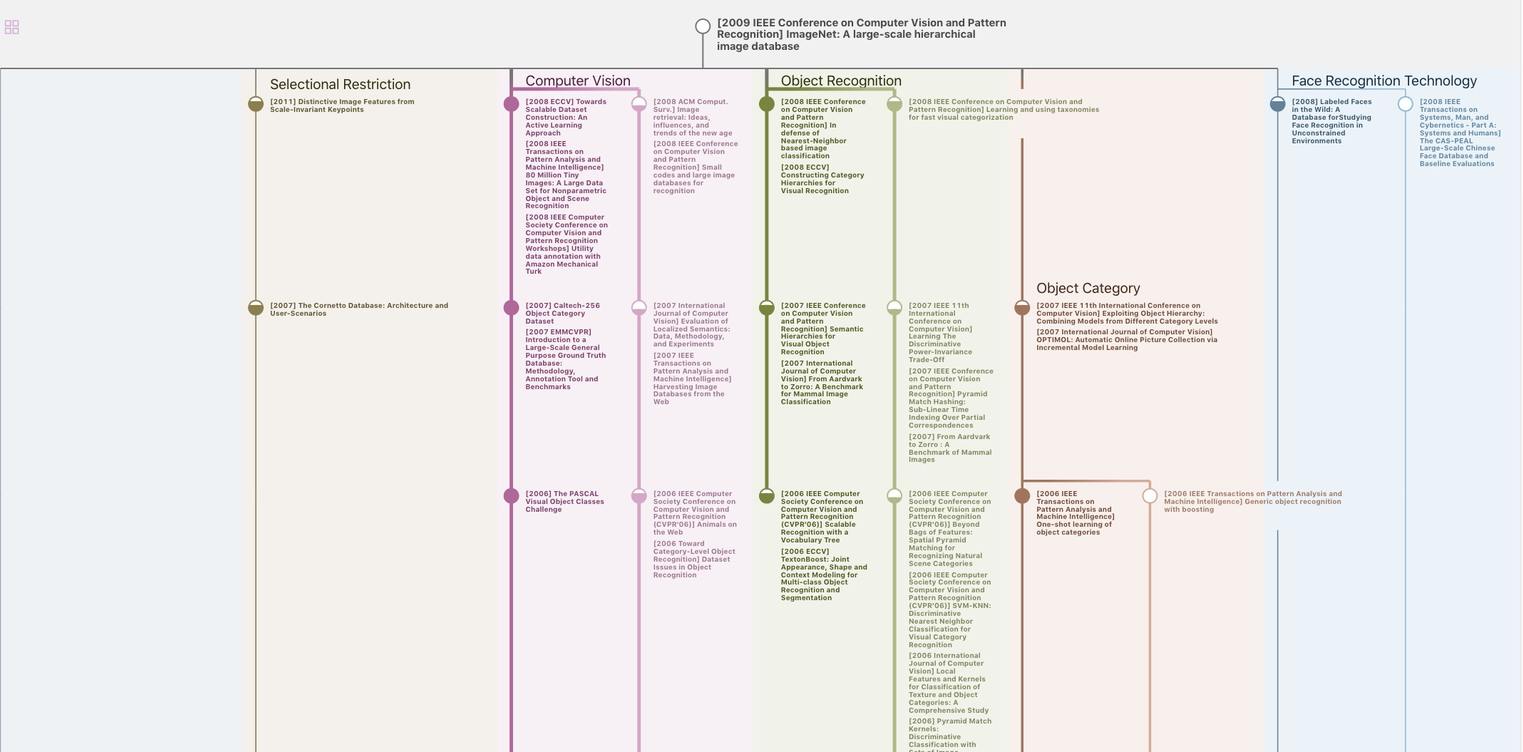
生成溯源树,研究论文发展脉络
Chat Paper
正在生成论文摘要