A lightweight and stochastic depth residual attention network for remote sensing scene classification
IET IMAGE PROCESSING(2023)
摘要
Due to the rapid development of satellite technology, high-spatial-resolution remote sensing (HRRS) images have highly complex spatial distributions and multiscale features, making the classification of such images a challenging task. The key to scene classification is to accurately understand the main semantic information contained in images. Convolutional neural networks (CNNs) have outstanding advantages in this field. Deep CNNs (D-CNNs) with better performance tend to have more parameters and higher complexity. However, shallow CNNs have difficulty extracting the key features of complex remote sensing images. In this paper, we propose a lightweight network with a random depth strategy for remote sensing scene classification (LRSCM). We construct a convolutional feature extraction module, DCAB, which incorporates depthwise separable convolutional and inverted residual structures, effectively reducing the numbers of required parameters and computations, and retains and utilizes low-level features. In addition, coordinate attention (CA) is integrated into the module, thereby further improving the network's ability to extract key local information. To further reduce the complexity of model training, the residual module adopts a stochastic depth strategy, providing the network with a random depth. Comparative experiments on five public datasets show that the LRSCM network can achieve results comparable to those of other state-of-the-art methods.
更多查看译文
关键词
convolutional neural network (CNN),coordinate attention (CA),remote sensing scene image classification,stochastic Depth
AI 理解论文
溯源树
样例
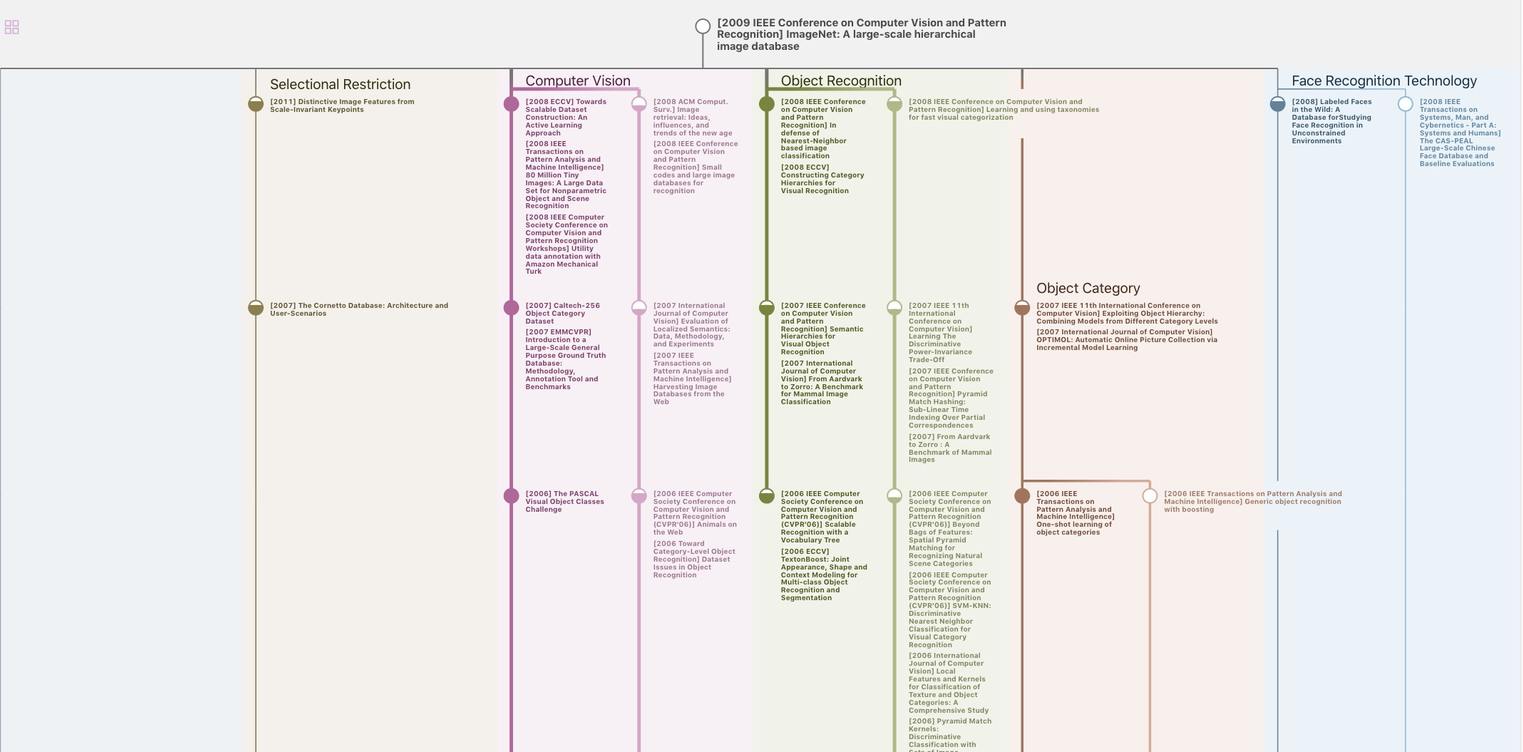
生成溯源树,研究论文发展脉络
Chat Paper
正在生成论文摘要