Do Sentence-Level Sentiment Interactions Matter? Sentiment Mixed Heterogeneous Network for Fake News Detection
IEEE TRANSACTIONS ON COMPUTATIONAL SOCIAL SYSTEMS(2023)
Abstract
With the proliferation of fake news, the spread of misleading information can easily cause social panic and group polarization. Many existing methods for detecting fake news rely on linguistic and semantic features extracted from the content of the news. Some existing approaches focus on sentiment analysis for fake news detection, but the sentiment changes and sentence-level emotional interactions in news classification are not fully analyzed. Fortunately, we observe that in long-form news, the change and mutual influence of sentiment between sentences are different. To extract the features of sentiment interaction between sentences in the article, we propose a graph attention network-based model that combines both sentiment and external knowledge comparison to meet the needs of fake news classification. We obtain the contextual sentiment representation and entity representation of the sentence through the heterogeneous network and the emotion interaction network and obtain the change of the sentiment vector through the emotion comparison network. We compare the entity vectors in the context with those corresponding knowledge base (KB)-based, combine them with the contextual semantic representation of the sentence, and finally input them into the classifier. In experiments, our model performs well in both single and multiclass classification, achieving the state-of-the-art accuracy on existing datasets.
MoreTranslated text
Key words
Fake news,Feature extraction,Semantics,Internet,Social networking (online),Sentiment analysis,Knowledge engineering,Fake news detection,heterogeneous graph,knowledge graph,sentiment analysis,sentiment comparison,text classification
AI Read Science
Must-Reading Tree
Example
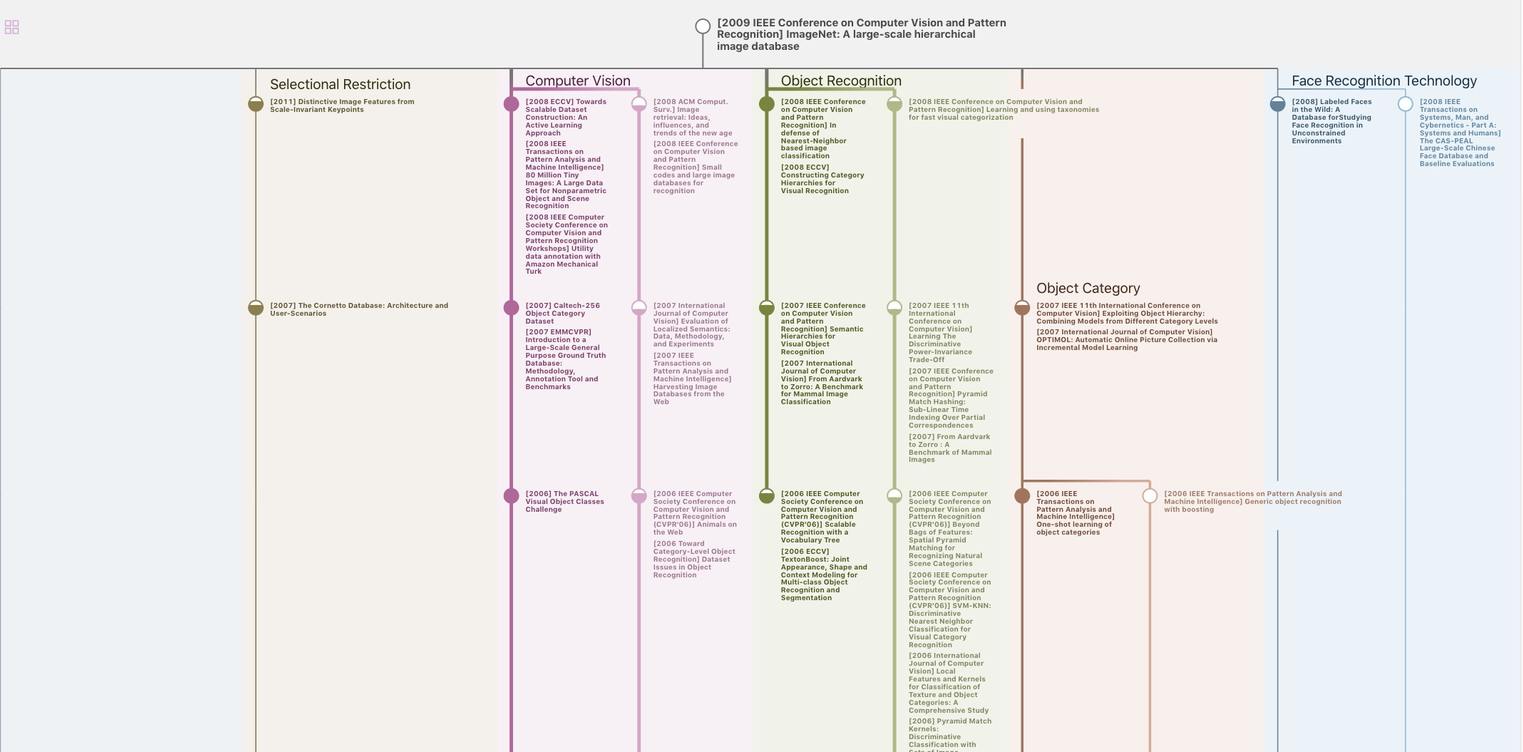
Generate MRT to find the research sequence of this paper
Chat Paper
Summary is being generated by the instructions you defined